Heterogeneous Mean-Field Multi-Agent Reinforcement Learning for Communication Routing Selection in SAGI-Net.
VTC Fall(2022)
摘要
The utilization of heterogeneous end devices such as the low earth orbit (LEO) satellite, unmanned aerial vehicles (UAVs) and ground users (GUs) deployed at different altitudes, known as the space-air-ground integrated network (SAGI-Net), can be quite promising towards a bunch of advanced applications. Whereas, the energy efficiency of the SAGI-Net communication system is a key criterion needed to be improved urgently in consideration that the inappropriate communication routing will undoubtedly cause a huge communication energy cost of the system especially with a large number of communication devices inside. In this paper, we proposed a novel communication routing selection model for the SAGI-Net system and established a heterogeneous multi-agent reinforcement learning (HMF-MARL) framework to optimize the communication energy efficiency of this system, where the mean-field theory was introduced to enhance the ability of classic MARL method while still maintaining a relatively low computational complexity. The experiment results show that the capacity of the heterogeneous multi-agent system has been improved by nearly 80% using the proposed HMF-MARL method compared with the classic MARL one, which hopefully shows the potential value on the implementation of the SAGI-Net system in the future.
更多查看译文
关键词
communication routing selection,reinforcement learning,mean-field,multi-agent,sagi-net
AI 理解论文
溯源树
样例
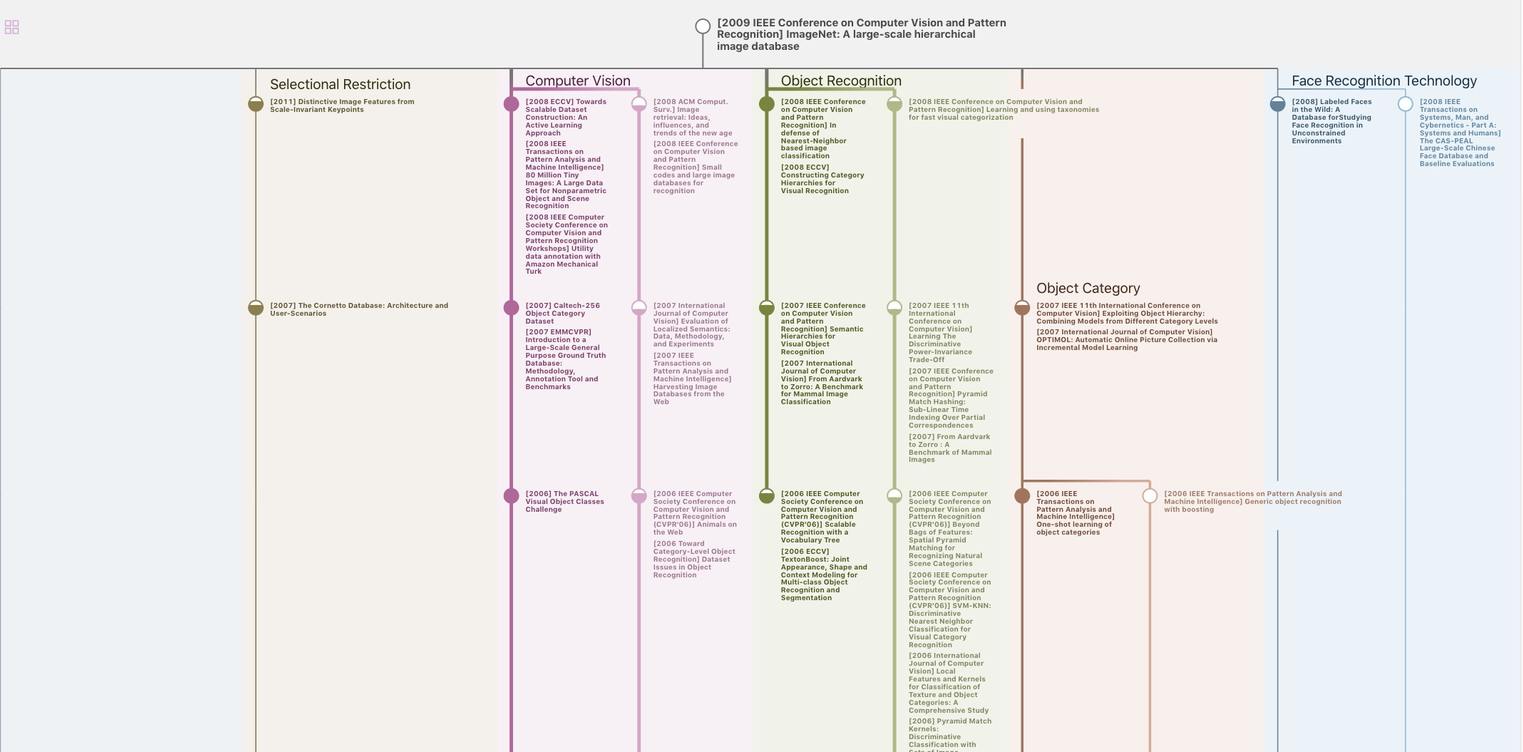
生成溯源树,研究论文发展脉络
Chat Paper
正在生成论文摘要