Learning Based Stochastic Data-Driven Predictive Control.
CDC(2022)
摘要
We consider a stochastic linear system with additive Gaussian noise and formulate a stochastic variant of Willems et al. fundamental lemma. Based on this, we formulate a stochastic optimal control problem wherein the system behavior is specified using the developed stochastic fundamental lemma. We call this the stochastic data-driven optimal control problem, which we then show to be equivalent to a statistical regression problem. Following this we construct a parameterized nonlinear estimator and use it to develop a learning algorithm to solve a stochastic data-driven predictive control problem. The proposed algorithm further enables us to consider different generalizations of the problem such as varying initial and Hankel matrix data obtained from stochastic linear and nonlinear system. Based on numerical simulations, we observe that the condition of persistency of excitation of inputs is not necessary for learning. This motivates us to formulate a lemma which indicates that the order of persistency of excitation required by inputs in the fundamental lemma is not strictly necessary.
更多查看译文
关键词
additive Gaussian noise,initial Hankel matrix data,learning algorithm,nonlinear system,numerical simulation,parameterized nonlinear estimator,statistical regression problem,stochastic data-driven optimal control problem,stochastic data-driven predictive control problem,stochastic fundamental lemma,stochastic linear system,stochastic optimal control problem
AI 理解论文
溯源树
样例
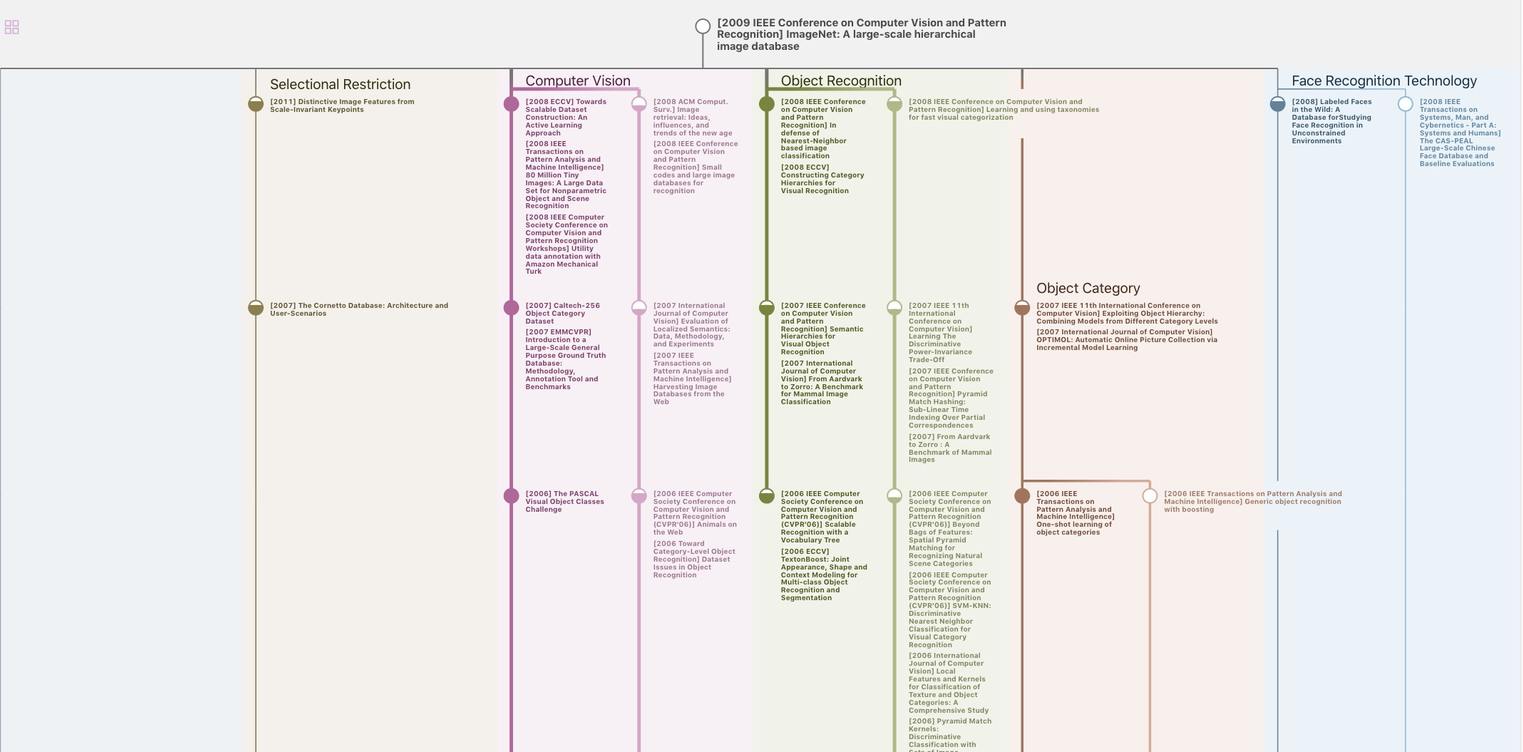
生成溯源树,研究论文发展脉络
Chat Paper
正在生成论文摘要