Cost-Effective Transfer Learning for Data Streams.
ICDM(2022)
摘要
In the online data stream environment, a model is typically not effective until a sufficient number of data instances have been seen. One solution to alleviate this issue is using model transfer. A major gap in current research is that they do not address the cost-effectiveness of model transfer in the online context, where processing time is crucial as data instances continuously arrive at high-speed. Model transfer in data streams involves continuously adapting the model to new data in the target stream. Suppose the target stream contains hard-to-learn patterns not covered by the transferred model. In that case, adaptation may incur extra processing time with little accuracy performance gains compared to simply building a new model for the target stream from scratch. Therefore, considering the cost of model transfer and target model construction is essential to balance the accuracy performance gains against computation cost for cost-effective model transfer. To address this gap, we propose a framework called OPERA (Online Transfer using Phantom Tree for Real-Time Adaptation), that orchestrates transfer learning based on the accuracy gain and runtime tradeoffs between transferring and adapting a source model, versus no transfer and constructing a new model for the target stream. We conduct extensive empirical studies to show that our framework can balance accuracy performance gains and runtime for costeffective transfer learning in the data stream environment.
更多查看译文
关键词
data streams,transfer,learning,cost-effective
AI 理解论文
溯源树
样例
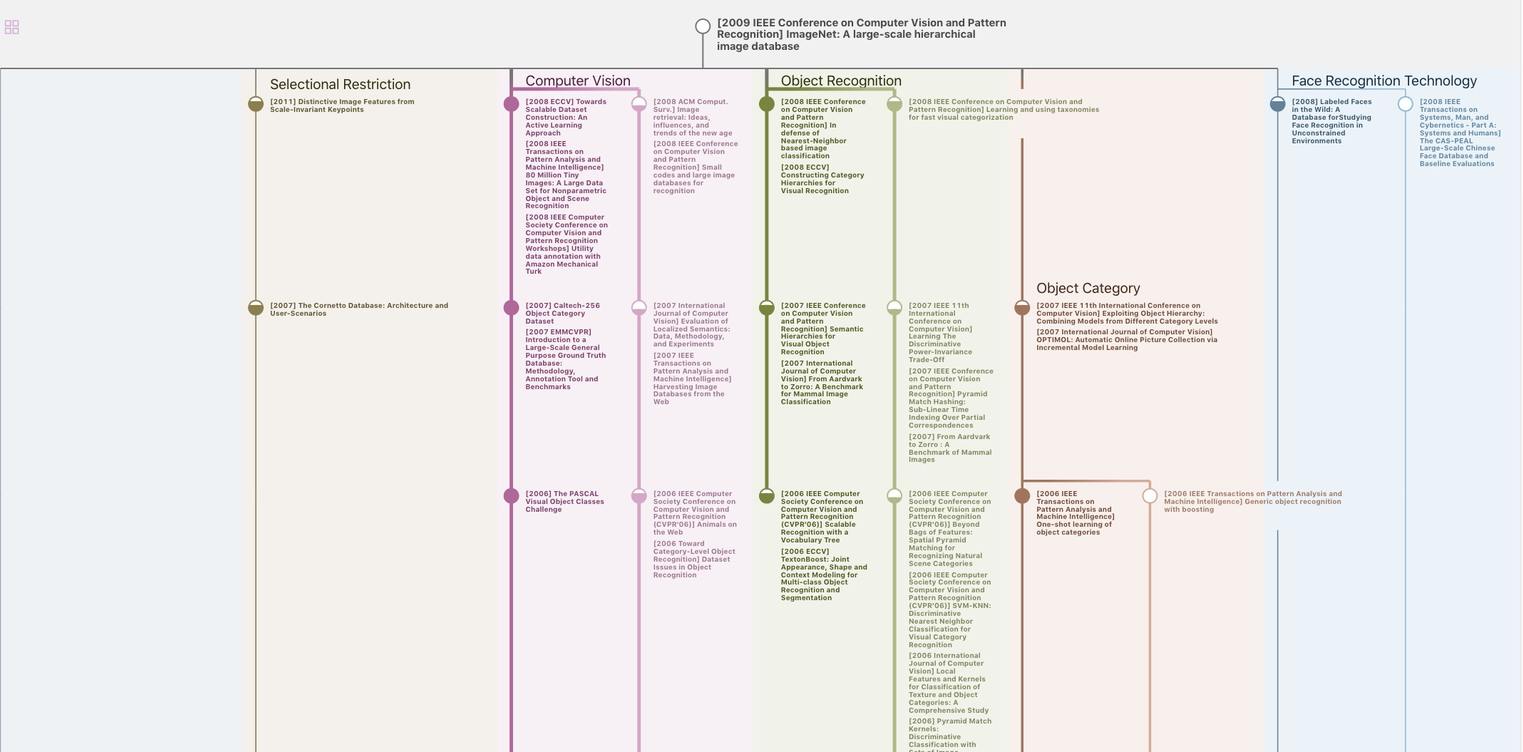
生成溯源树,研究论文发展脉络
Chat Paper
正在生成论文摘要