SHCN: Self-supervised General Hypergraph Clustering Network
Big Data(2022)
摘要
Clustering is a fundamental and hot issue in the unsupervised learning area. With the rapid development of deep learning and graph neural networks (GNNs) techniques, researchers have proposed a series of effective clustering methods. However, most existing approaches adopt a conventional graph to aggregate the neighborhood information, where only the pairwise relations are considered. Moreover, the redundancy/noise in the raw data samples may result in less accurate sample relations and inferior clustering results. In this paper, we proposed a new GNNs based clustering method, which adopts the hypergraph learning approach to explore the high-order relationship for accurate relation learning. Specifically, we first construct two hypergraph representations based on the topology feature and attribute feature from data samples. Then, a self-supervised structure is integrated to learn a cross-correlation matrix from the original hypergraph to act as a higher-order neighborhood with reduced redundancy and noise. Finally the embedding representation of the clustering space is learned in the graph convolution. The proposed method has been evaluated on six public datasets for clustering tasks. Experimental results show that our proposed method outperforms the state-of-the-art ones.
更多查看译文
关键词
Hypergraph,Self-supervised Learning,Deep hypergraph embedding,Deep clustering
AI 理解论文
溯源树
样例
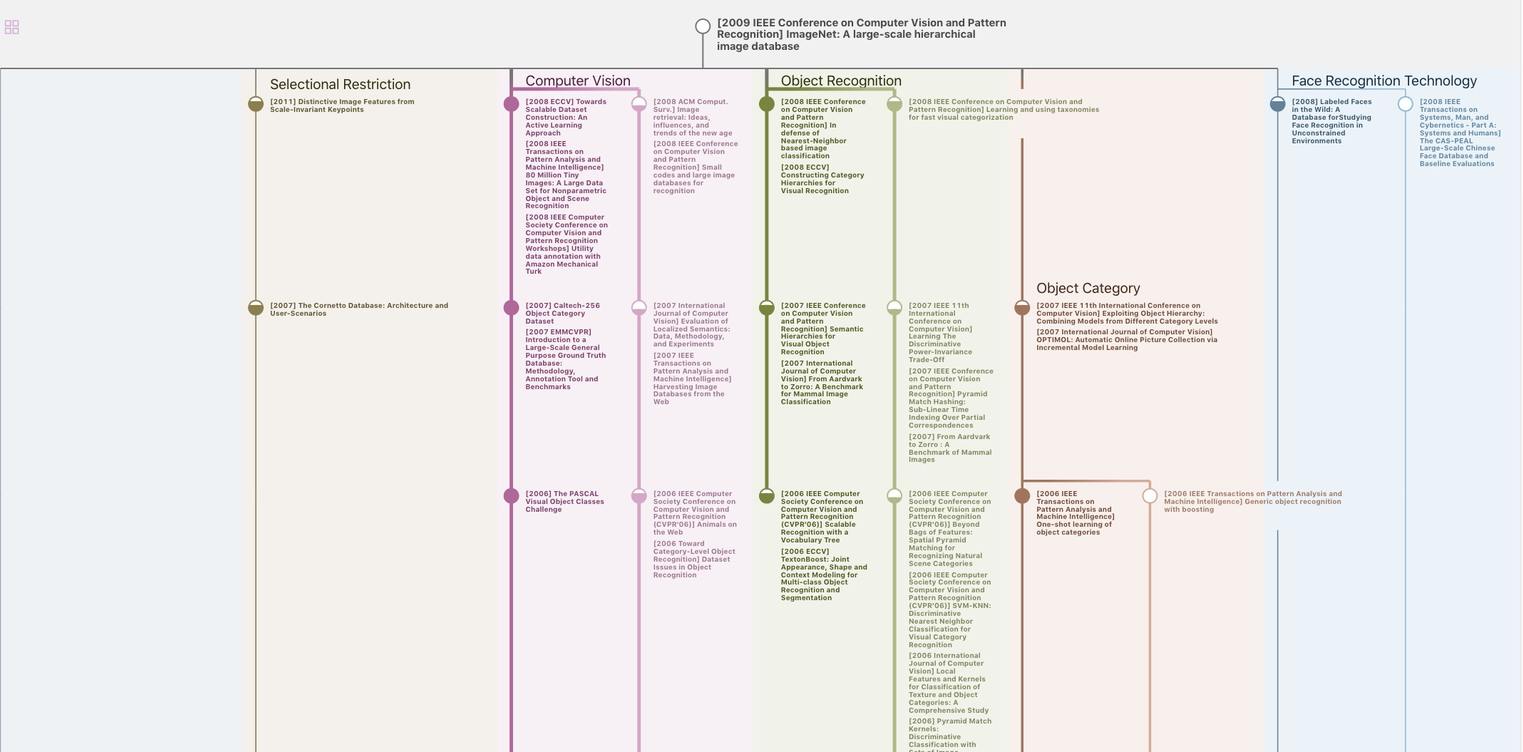
生成溯源树,研究论文发展脉络
Chat Paper
正在生成论文摘要