KGIQ: Scalable Translation of User-Specified Examples into Knowledge-Graph Queries
Big Data(2022)
摘要
Querying large-scale knowledge graphs (KGs) can be difficult for users in real-life scenarios, in which formal graph query languages potentially present usability barriers. Query-By-Example (QBE) approaches, which allow users to specify their query intent with examples, have become an emerging trend to address this issue. However, existing QBE approaches either require user-specified examples to provide values of all the KG attributes, or may return incorrect formalizations of user query intent due to the lack of user interaction. In this paper we propose an approach called Knowledge Graph Intuitive Querier (KGIQ) that addresses both challenges on large-scale KGs by using novel scalable algorithms. Unlike existing approaches, KGIQ ensures correct translation of user-specified examples into formal executable graph queries by interacting with users in a lightweight manner. Our experimental results suggest that KGIQ can correctly formalize graph queries on large-scale KGs with the help of uncomplicated user interactions and a feedback loop to improve user experience, while consistently outperforming the state of the art in terms of efficiency and outcome quality.
更多查看译文
关键词
scalable translation,examples,user-specified,knowledge-graph
AI 理解论文
溯源树
样例
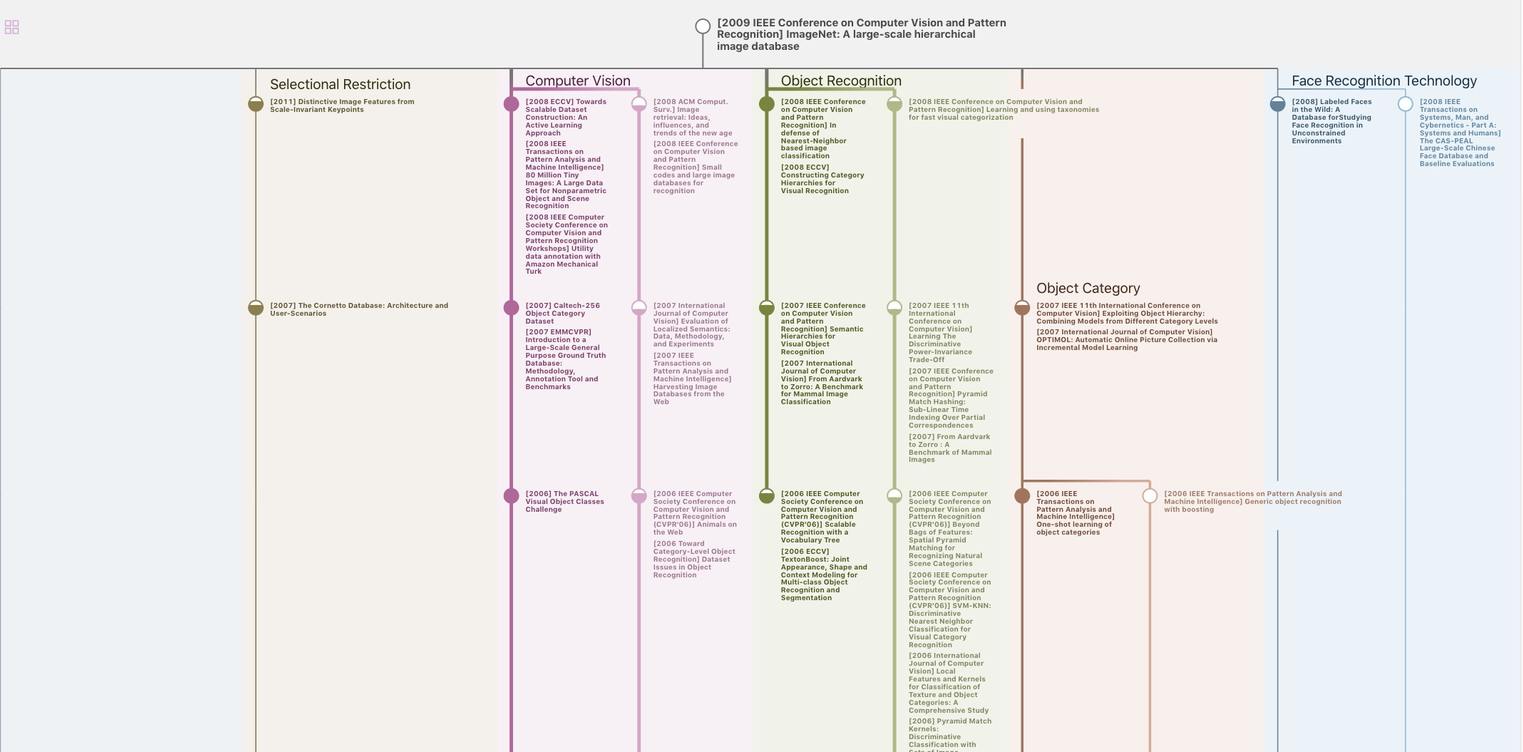
生成溯源树,研究论文发展脉络
Chat Paper
正在生成论文摘要