Tracking trajectories of multiple long-term conditions using dynamic patient-cluster associations.
Big Data(2022)
摘要
Momentum has been growing into research to better understand the dynamics of multiple long-term conditions – multimorbidity (MLTC-M), defined as the co-occurrence of two or more long-term or chronic conditions within an individual. Several research efforts make use of Electronic Health Records (EHR), which represent patients’ medical histories. These range from discovering patterns of multimorbidity, namely by clustering diseases based on their co-occurrence in EHRs, to using EHRs to predict the next disease or other specific outcomes. One problem with the former approach is that it discards important temporal information on the co-occurrence, while the latter requires "big" data volumes that are not always available from routinely collected EHRs, limiting the robustness of the resulting models.In this paper we take an intermediate approach, where initially we use about 143,000 EHRs from UK Biobank to perform time-independent clustering using topic modelling, and Latent Dirichlet Allocation specifically. We then propose a metric to measure how strongly a patient is "attracted" into any given cluster at any point through their medical history. By tracking how such gravitational pull changes over time, we may then be able to narrow the scope for potential interventions and preventative measures to specific clusters, without having to resort to full-fledged predictive modelling.In this preliminary work we show exemplars of these dynamic associations, which suggest that further exploration may lead to actionable insights into patients’ medical trajectories.
更多查看译文
关键词
trajectories,dynamic,long-term,patient-cluster
AI 理解论文
溯源树
样例
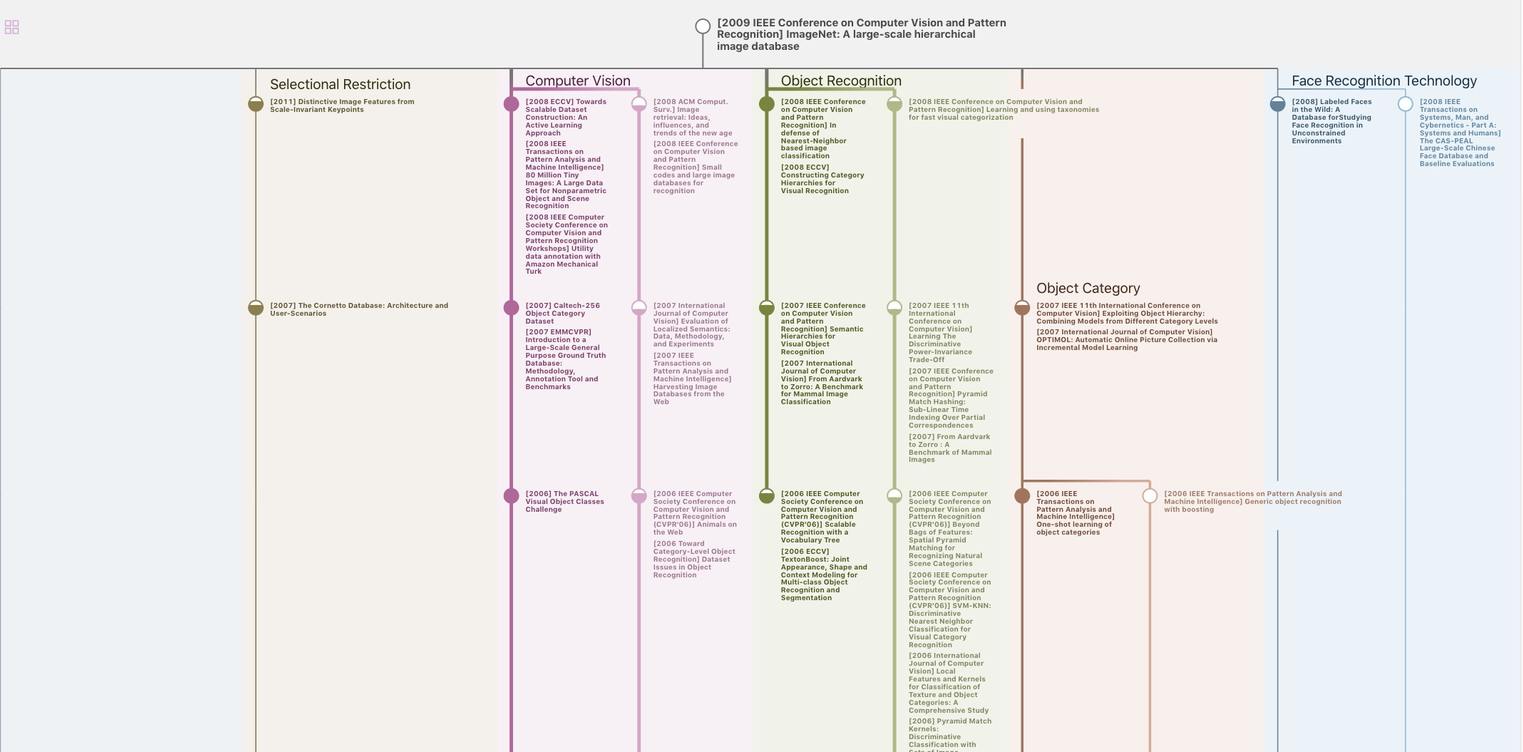
生成溯源树,研究论文发展脉络
Chat Paper
正在生成论文摘要