Lossy Predictive Models for Accurate Classification Algorithms
Big Data(2022)
摘要
Recent years have witnessed an upsurge of interest in lossy compression due to its potential to significantly reduce data volume with adequate exploitation of the spatiotemporal properties of IoT datasets. However, striking a balance between compression ratios and data fidelity is challenging, particularly when losing data fidelity impacts downstream data analytics noticeably. In this paper, we propose a lossy prediction model dealing with binary classification analytics tasks to minimize the impact of the error introduced due to lossy compression. We specifically focus on five classification algorithms for frost prediction in agricultural fields allowing preparation by the predictive advisories to provide helpful information for timely services. While our experimental evaluations reaffirm the nature of lossy compressions where allowing higher errors offers higher compression ratios, we also observe that the classification performance in terms of accuracy and F-1 score differs among all the algorithms we evaluated. Specifically, random forest is the best lossy prediction model for classifying frost. Lastly, we show the robustness of the lossy prediction model based on the data fidelity in prediction performance.
更多查看译文
关键词
lossy predictive models,accurate classification algorithms
AI 理解论文
溯源树
样例
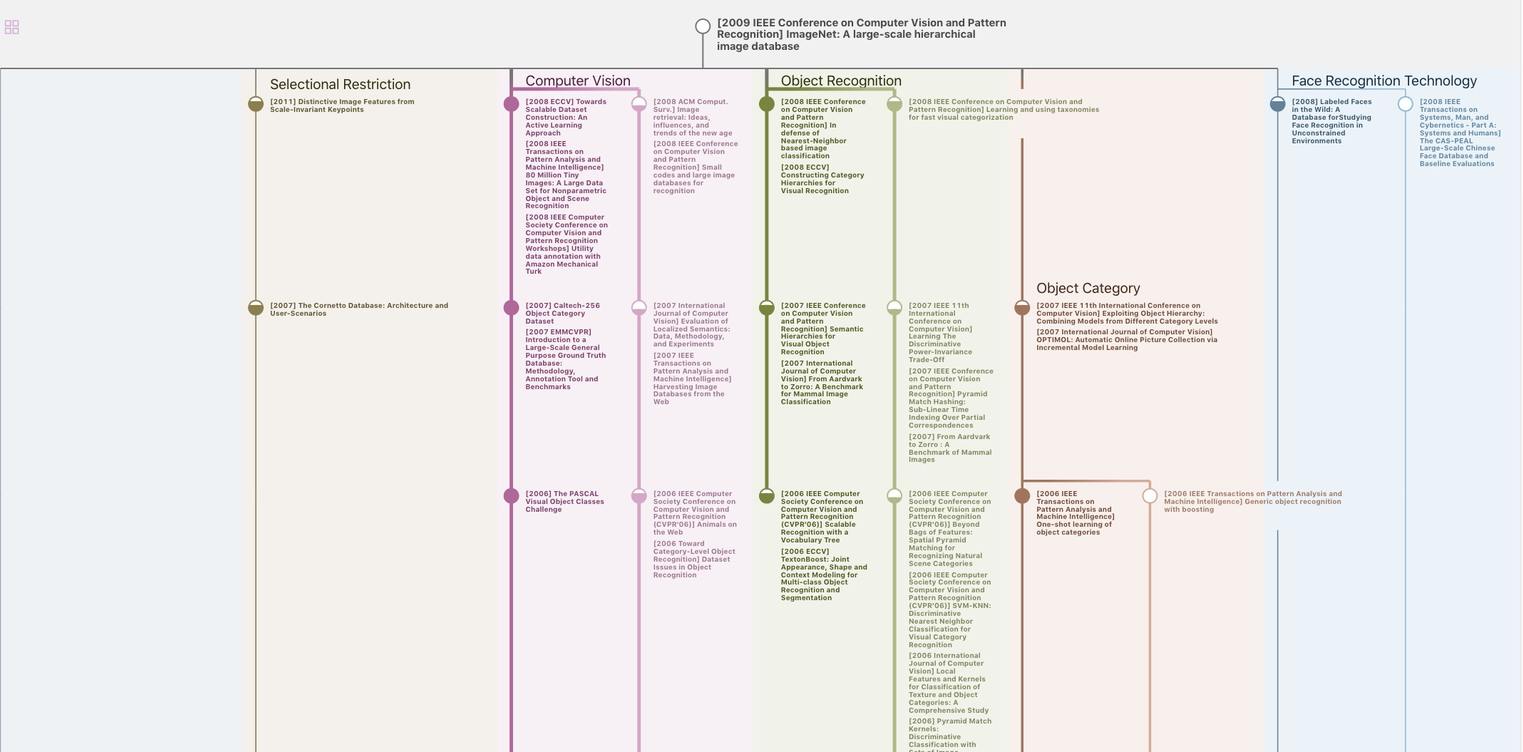
生成溯源树,研究论文发展脉络
Chat Paper
正在生成论文摘要