Typhon: Parallel Transfer on Heterogeneous Datasets for Cancer Detection in Computer-Aided Diagnosis
Big Data(2022)
摘要
We present Typhon, a new Deep Learning framework that trains a single model using multiple, heterogeneous datasets leveraging parallel transfer. This aims to improve the performance of Deep Learning methods in critical applications afflicted by data scarcity, such as computer-aided diagnosis for cancer detection, where large datasets are rare or unfeasible but many smaller datasets may be available. The key idea is to assemble sufficient data for t raining deep models by selecting a set of multiple, potentially smaller and heterogeneous datasets, as long as they all exhibit similar visual features, such as common with medical imaging applications. The Typhon model architecture is composed of a single Feature Extractor and multiple Decision Makers, in sequence but explicitly separated. The Feature Extractor is trained using all datasets with a focus on producing generic features which are useful across all datasets. The Decision Makers are each paired with a different dataset, and specialized to take decisions based on the output of the Feature Extractor. Our training method is based on the concept of parallel transfer: on each epoch, we train on just one batch from each dataset in turn. This is done by pairing the correct Decision Maker on top of the shared Feature Extractor, then training the resulting model end-to-end on the data batch using classical methods. The actual design is inherently more complex, as we had to overcome a set of major challenges such as dataset imbalance, moving target, catastrophic forgetting, and issues with initialization viability. Once made viable, however, this methods excels at strictly enforcing feature generalization and delaying or even preventing overfitting. We present our results on the widely adopted PROSTATEx MRI dataset for prostate cancer classification, using additional datasets of brain MRI and lung CT images to boost the model’s performance. Typhon improves on our previous work based on sequential transfer (Hydra) by over 7%, which compounds to a 15% improvement over classical methods and 12% over transfer learning, while only seeing 54% more samples than classical end-to-end training on a single dataset.
更多查看译文
关键词
Data Scarcity,Parallel Transfer,Medical Imaging,Cancer Detection,Overfitting
AI 理解论文
溯源树
样例
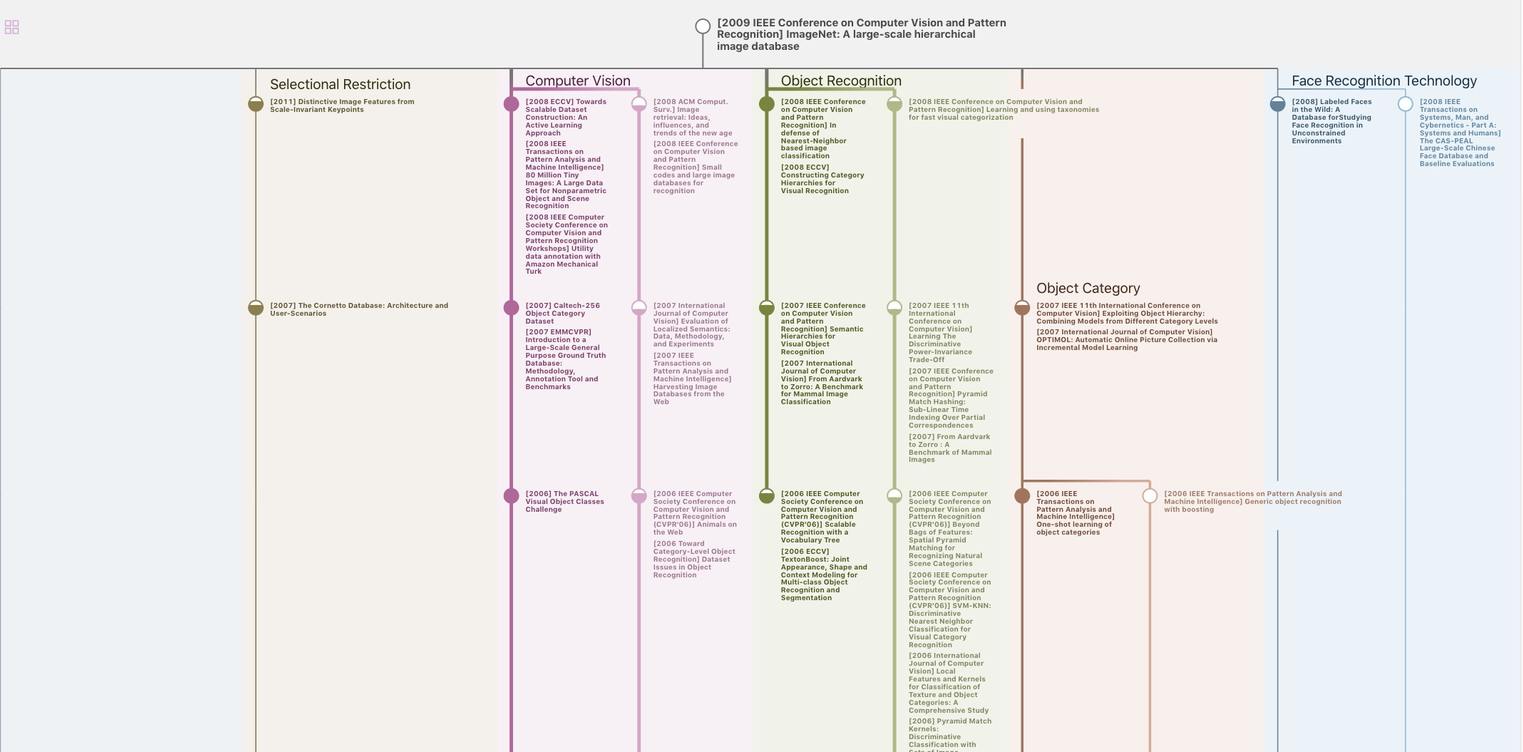
生成溯源树,研究论文发展脉络
Chat Paper
正在生成论文摘要