Attention multiple instance learning with Transformer aggregation for breast cancer whole slide image classification.
BIBM(2022)
摘要
Recently, attention-based multiple instance learning (MIL) methods have received more concentration in histopathology whole slide image (WSI) applications. However, existing attention-based MIL methods rarely consider the cross-channel information interaction of pathology images when identifying discriminant patches. Additionally, they also have limitations on capturing the correlation between different discriminant instances for the bag-level classification. To address these challenges, we present a novel attention-based MIL model (AMIL-Trans) for breast cancer WSI classification. AMIL-Trans first embeds the efficient channel attention to realize the cross-channel interaction of pathology images, thus computing more robust features for instance selection without introducing too much computation cost. Then, it leverages vision Transformer encoder to directly aggregate selected instance features for better bag-level prediction, which effectively considers the correlation between different discriminant instances. Experiment results illustrate that AMIL-Trans respectively achieves its optimal AUC of 94.27% and 84.22% on the Camelyon-16 dataset and MSK external validation dataset, demonstrating the competitive performance compared with state-of-the-art MIL methods on breast cancer WSI classification task. The code will be available at https://github.con CunqiaoHou/AMIL-Trans.
更多查看译文
关键词
whole slide image classification,transformer aggregation,multiple instance,attention
AI 理解论文
溯源树
样例
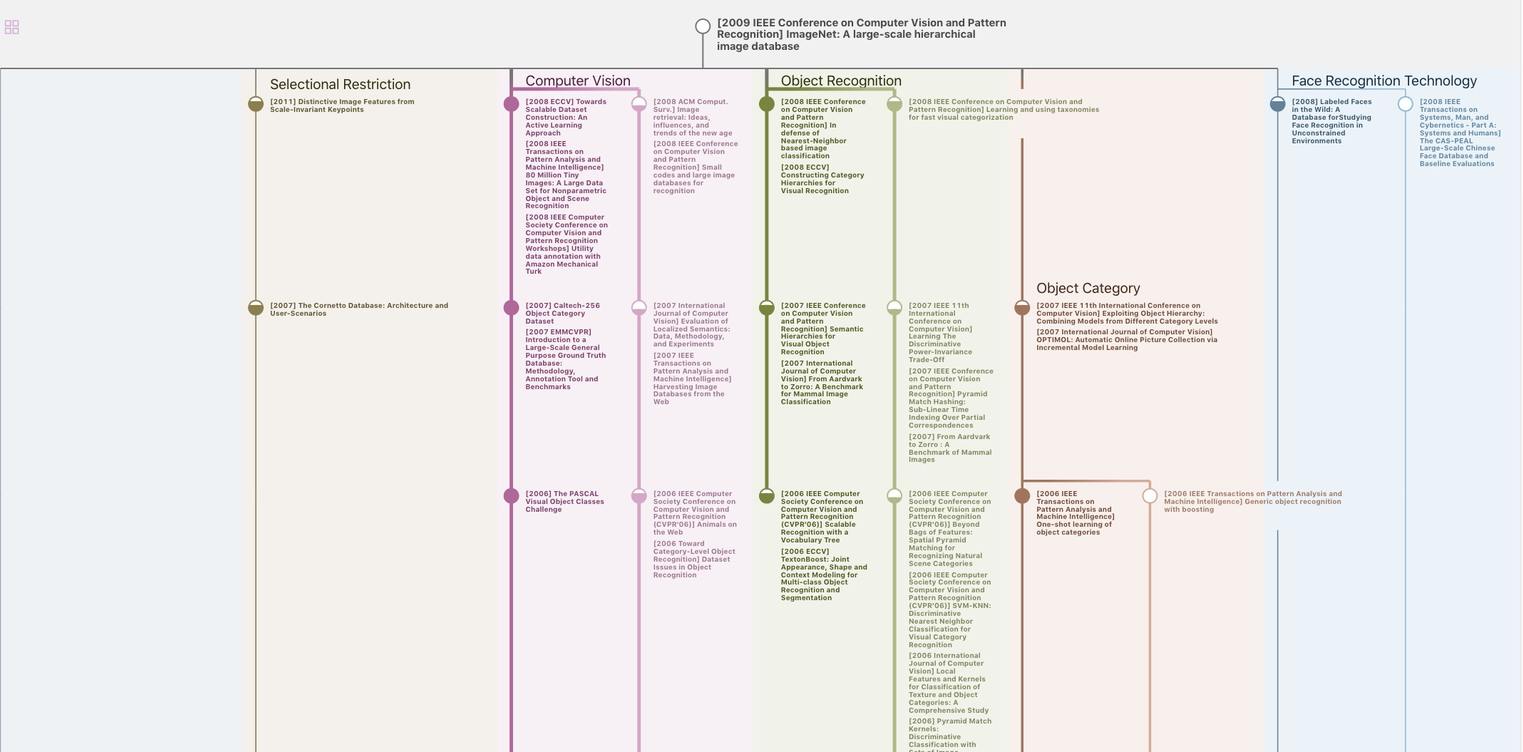
生成溯源树,研究论文发展脉络
Chat Paper
正在生成论文摘要