Beat-by-Beat Classification of ECG Signals Using Machine Learning Algorithms to Detect PVC Beats for Real-time Predictive Cardiac Health Monitoring
BIBM(2022)
摘要
A premature ventricular contraction (PVC) disrupt the normal heart rhythm and indicate underlying cardiac disease. We aim to detect these PVC beats from electrocardiogram (ECG/EKG) data by automatically classifying these ECG beats with high accuracy in real-time. In this study, we used MIT BIH Long-Term Electrocardiogram Database (ltdb) dataset from the PhysioNet database. We extract signal-specific features and signal-independent features and combine them for feature ranking. We use principal component analysis (PCA), elastic net regularization (ENR), univariate filter of constant, quasi constant and duplicate feature removal (CQCDFR) and analysis of variance test (ANOVA) for feature selection. We take the top 10 features for four methods and classify them separately. The machine learning model explored is the random forest classifier. In our analysis, elastic net regularization performed best in terms of accuracy in cardiac patients. We further use the feature with the best accuracy in four algorithms to test sensitivity, specificity, accuracy, precision, f1-score to evaluate statistics. The overall accuracy of elastic net regularization for classifying the highest first 8 feature data is 97.8%. The sensitivity was 94.7% and the specificity was 99.6%. The accuracy rate is 99.6%, and the F1 score is 97.1%. The method can accurately detect ECG beats and analyze categories for real-time cardiac monitoring for feedback to the use patient. Efficient feature selection minimizes the number of features used and reduces the power consumption of the monitoring device.
更多查看译文
关键词
ecg signals,pvc beats,machine learning algorithms,beat-by-beat,real-time
AI 理解论文
溯源树
样例
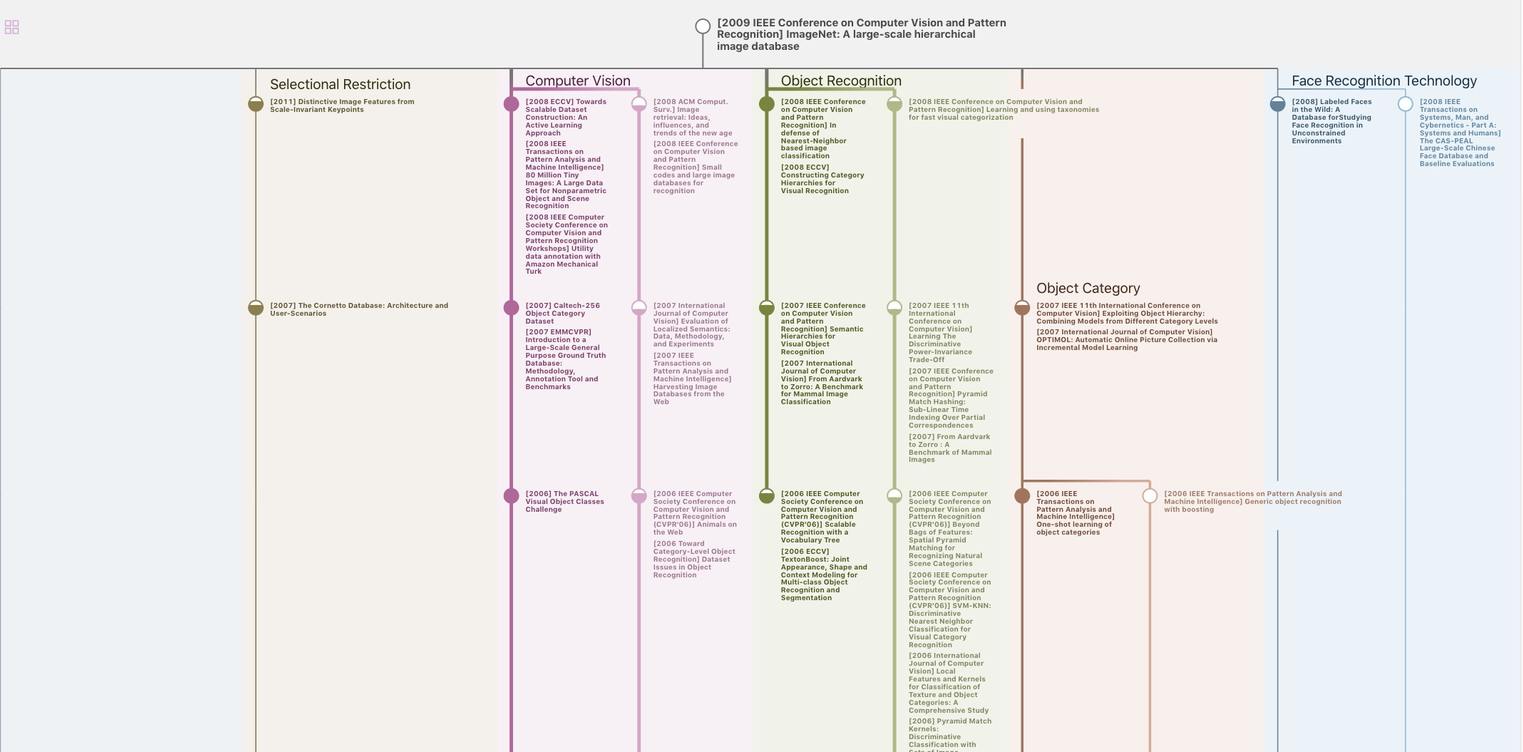
生成溯源树,研究论文发展脉络
Chat Paper
正在生成论文摘要