Computationally-Efficient Vision Transformer for Medical Image Semantic Segmentation Via Dual Pseudo-Label Supervision
ICIP(2022)
摘要
Ubiquitous accumulation of large volumes of data, and increased availability of annotated medical data in particular, has made it possible to show the many and varied benefits of deep learning to the semantic segmentation of medical images. Nevertheless, data access and annotation come at a high cost in clinician time. The power of Vision Transformer (ViT) is well-documented for generic computer vision tasks involving millions of images of every day objects, of which only relatively few have been annotated. Its translation to relatively more modest (i.e. thousands of images of) medical data is not immediately straightforward. This paper presents practical avenues for training a Computationally-Efficient Semi-Supervised Vision Transformer (CESS-ViT) for medical image segmentation task.We propose a self-attention-based image segmentation network which requires only limited computational resources. Additionally, we develop a dual pseudo-label supervision scheme for use with semi-supervision in a simple pure ViT.Our method has been evaluated on a publicly available cardiac MRI dataset with direct comparison against other semi-supervised methods. Our results illustrate the proposed ViT-based semi-supervised method outperforms the existing methods in the semantic segmentation of cardiac ventricles.
更多查看译文
关键词
Semantic Segmentation, Semi-Supervised Learning, Vision Transformer, Cardiac MRI
AI 理解论文
溯源树
样例
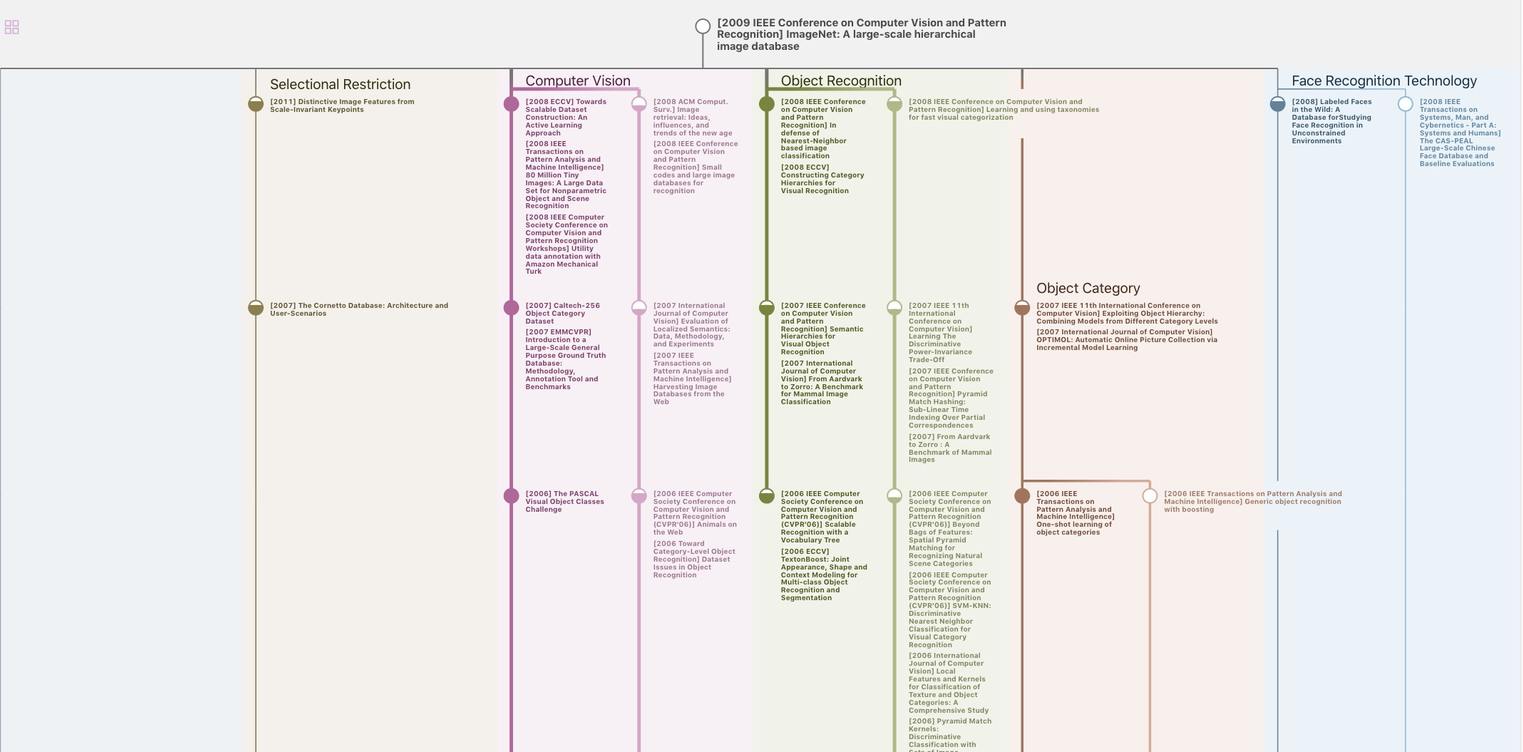
生成溯源树,研究论文发展脉络
Chat Paper
正在生成论文摘要