Improving Generalization of Reinforcement Learning Using a Bilinear Policy Network.
ICIP(2022)
摘要
In deep reinforcement learning (DRL), the agent is usually trained on seen environments by optimizing a policy network. However, it is difficult to be generalized to unseen environments properly, even when the environmental variations are insignificant. This is partly because the policy network cannot effectively learn the representation of visual difference that is subtle among highly similar states in the environments. Because a bilinear structured model containing two feature extractors allows pairwise feature interactions in a translationally invariant manner which makes it particularly useful for subtle difference recognition among highly similar states, in this work, a bilinear policy network is employed to enhance representation learning, and thus to improve generalization of the DRL. The proposed bilinear policy network is tested on various DRL task, including a control task on path planning for active object detection, and Grid World, an AI game task. The test results show that the generalization of DRL can be improved by the proposed network.
更多查看译文
关键词
bilinear policy network,reinforcement learning,generalization
AI 理解论文
溯源树
样例
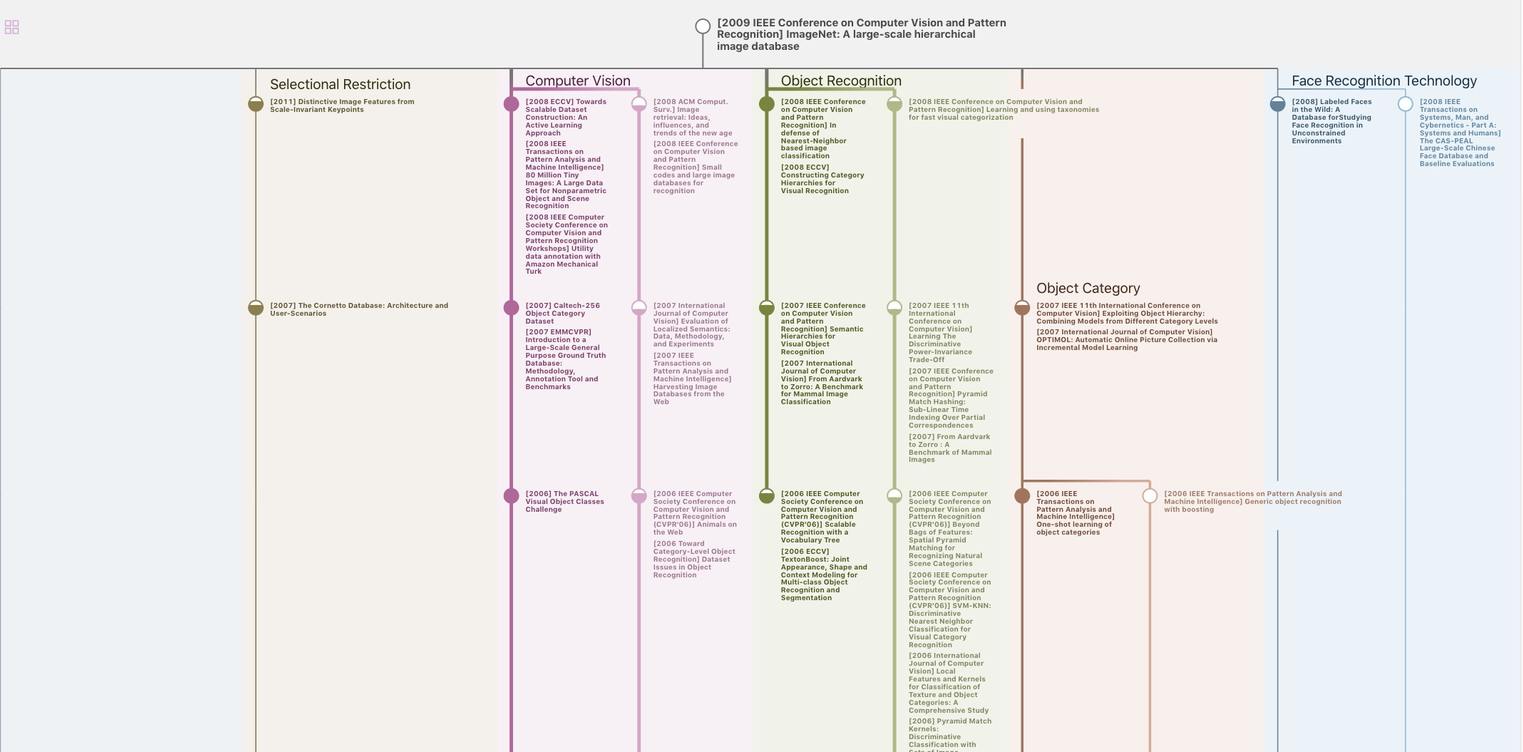
生成溯源树,研究论文发展脉络
Chat Paper
正在生成论文摘要