Relation Classification Model Performance as a Function of Dataset Label Noise.
COMAD/CODS(2023)
摘要
The central question that this paper address is: How does the performance of a supervised model vary with respect to the label noise in the testing and training dataset? Answering this question is crucial for many real-world applications for two main reasons. First, most datasets used for training large supervised models such as Deep Neural Networks (DNNs) are crowd-sourced. Such datasets are known to have significant label noise due to multiple factors such as the complexity of the annotation task and low wages for crowd-sourced annotators. Second, these crowd-sourced datasets are large. Reannotating such large datasets is a time-consuming and costly process. Our work aims to understand the relation between the cost of data reannotation and the performance of supervised models. Understanding this relationship can be helpful while planning for data reannotation. We focus on a specific supervised learning task of Relation Classification (RC). It is the task of predicting a relation label between a pair of real-world entities in a given natural language sentence. It is an important NLP task with applications in multiple domains such as knowledge graph completion, and question answering. All the recent and state-of-the-art RC models are based on the Deep Learning paradigm. Recent studies have shown that the quality of the dataset is a significant bottleneck in improving the performance of supervised machine learning models for RC [1, 2]. One possible solution for improving the data quality is to reannotate the data to reduce the label noise. Existing works on RC dataset reannotation follow two extremes. They either select only a tiny fraction for reannotation [1] or go for reannotation of the complete data [2]. To overcome this rigidity, we introduce the concept of a reannotation budget. It provides flexibility about what fraction of the dataset to reannotate. For a given reannotation budget, we also have to decide which subset of the data to reannotate. We explore four strategies to select sentences for reannotation. We perform extensive experiments using a popular RC dataset TACRED[3] and a set of recent Deep Learning-based RC models. While varying the reannotation budget, we track the performance of RC models through the F1 score. Our work has two specific research contributions. First, this is the first work that analyzes RC model performance as a function of the amount of label noise in the data. Such analysis will be helpful while planning the data reannotation process. We observe that a significant improvement can be achieved in the RC model performance by reannotating only a part of the training data (Figure 1a). Second, we show that the reported performance of RC models on noisy datasets is inflated. The F1 score of these models drops from the reported range of 60%-70% to as low as below 50% when tested on clean test data (Figure 1b). This drop in performance should be considered while deploying the RC models for real-world applications.
更多查看译文
AI 理解论文
溯源树
样例
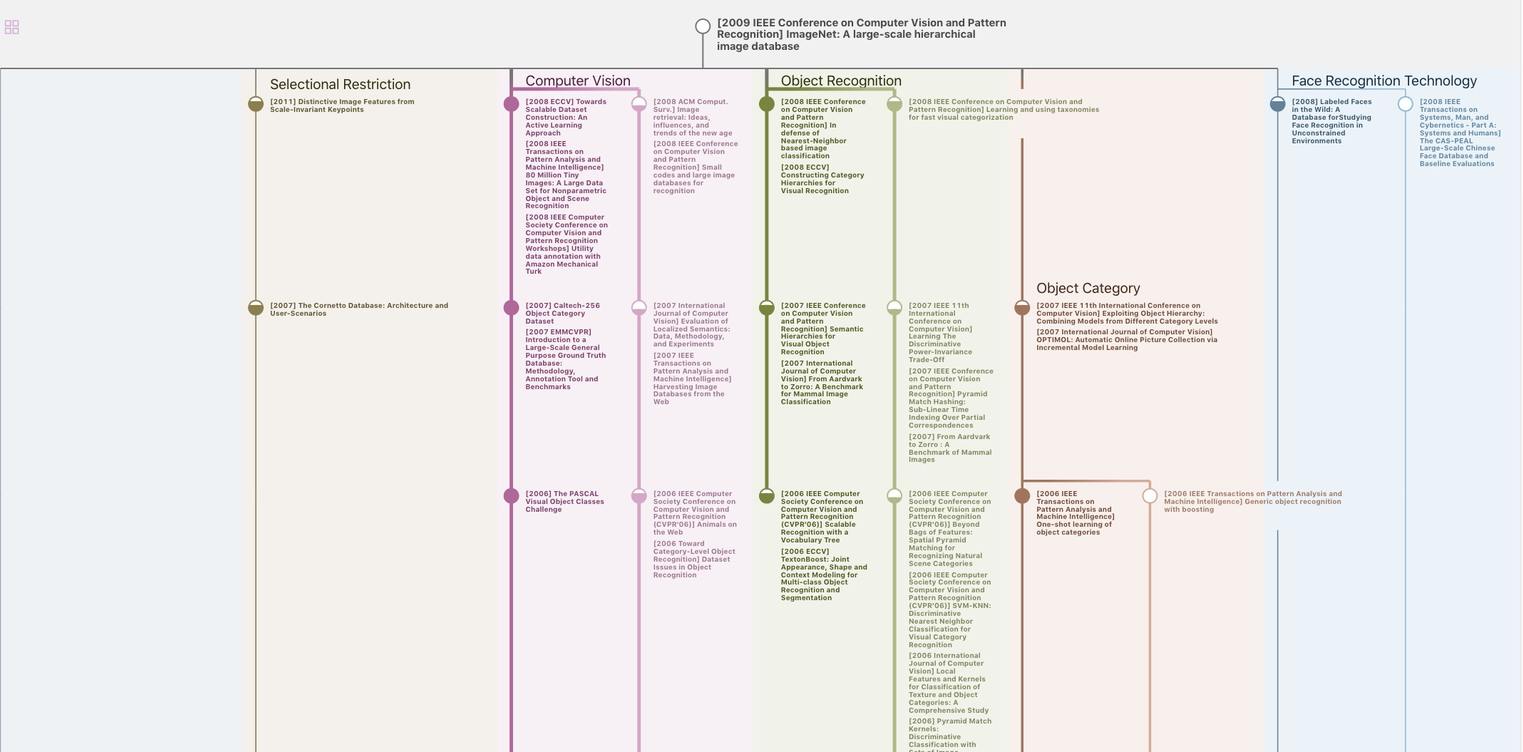
生成溯源树,研究论文发展脉络
Chat Paper
正在生成论文摘要