A Flow Prediction Model of Bike-Sharing Based on Cycling Context.
CollaborateCom (1)(2022)
摘要
The prediction of the number of bike-sharing is of great significance to maintain the balance of the number of bikes at each station. The cycling trajectory of users is dynamically changing and different in different districts of a city. This has caused the problem of no bikes at some stations, while others have accumulated bikes. However, most of the research work adds contexts such as spatiotemporal and weather features to the bike flow prediction, but ignores the problem of the imbalance of the number of bikes at each station. Therefore, we predict the number of bikes at the station based on the context features. To this end, we study the context features based on user's cycling data, and consider the features of time and climate. Along this line, we first analyze the features of time and climate to find the user's cycling habits. Then, we introduce the Long Short-Term Memory (LSTM) to capture the dependence relationship between time series. Using the Attention Mechanism to obtain key features can reduce prediction errors. We propose the context-based prediction model of the number of bike-sharing on the station with LSTM and Attention Mechanism (C-LSTMAM). This model can specifically capture more important context feature for the prediction. Finally, extensive experiments on real-world datasets demonstrate the effectiveness of the C-LSTMAM.
更多查看译文
关键词
Prediction of the number of bike-sharing, Context analysis, Long Short-Term Memory, Attention mechanism
AI 理解论文
溯源树
样例
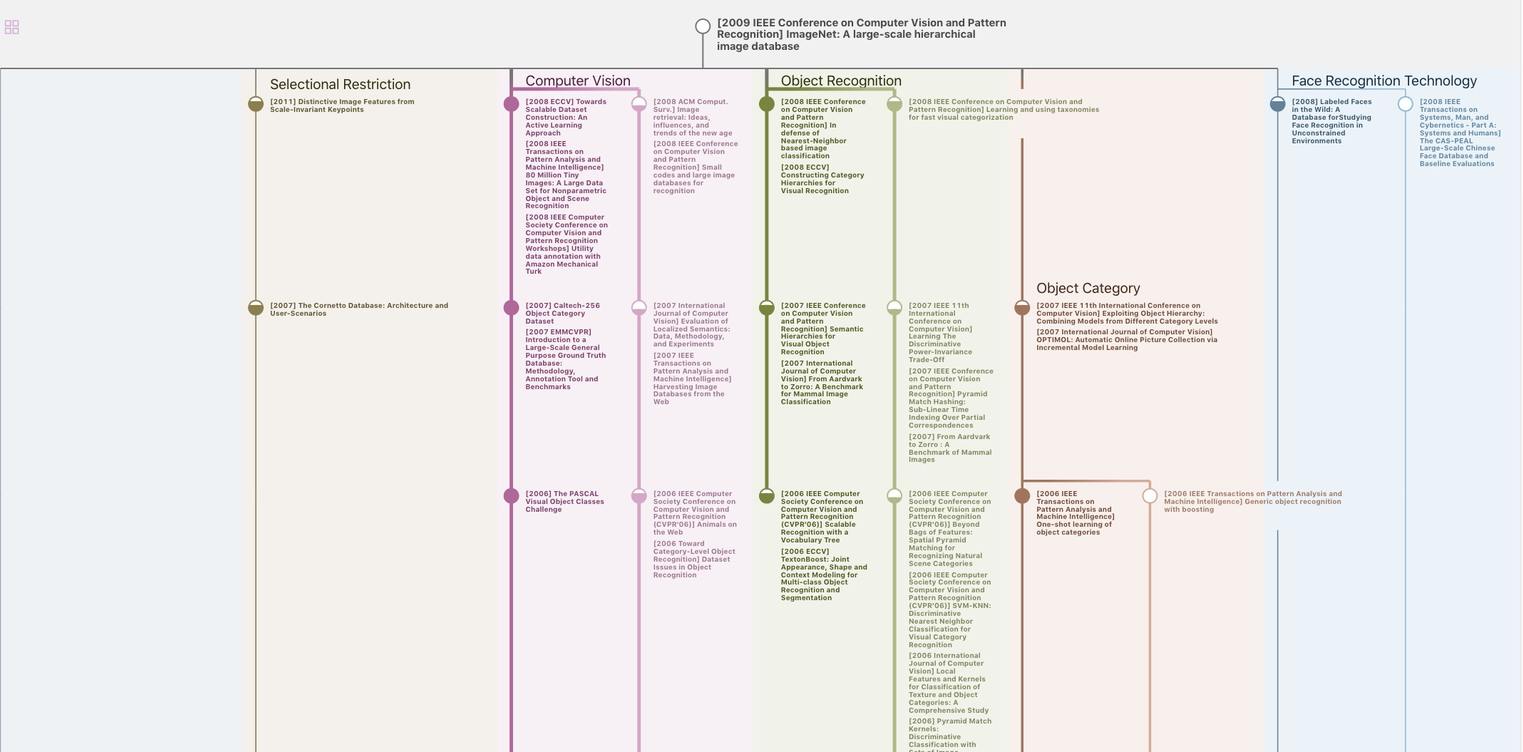
生成溯源树,研究论文发展脉络
Chat Paper
正在生成论文摘要