Incorporating Physics-Based Models into Data Driven Approaches for Air Leak Detection in City Buses.
PKDD/ECML Workshops (2)(2022)
摘要
In this work-in-progress paper two types of physics-based models, for accessing elastic and non-elastic air leakage processes, were evaluated and compared with conventional statistical methods to detect air leaks in city buses, via a data-driven approach. We have access to data streamed from a pressure sensor located in the air tanks of a few city buses, during their daily operations. The air tank in these buses supplies compressed air to drive various components, e.g. air brake, suspension, doors, gearbox, etc. We fitted three physics-based models only to the leakage segments extracted from the air pressure signal and used fitted model parameters as expert features for detecting air leaks. Furthermore, statistical moments of these fitted parameters, over predetermined time intervals, were compared to conventional statistical features on raw pressure values, under a classification setting in discriminating samples before and after the repair of air leak problems. The result of this exploratory study, on six air leak cases, shows that the fitted parameters of the physics-based models are useful for discriminating samples with air leak faults from the fault-free samples, which were observed right after the repair was performed to deal with the air leak problem. The comparison based on ANOVA F-score shows that the proposed features based on fitted parameters of physics-based models outrank the conventional features. It is observed that features of a non-elastic leakage model perform the best.
更多查看译文
关键词
Fault detection, Air leaks, Elastic air leakage model, Non-elastic air leakage model, Physics-informed machine learning, Explainable predictive maintenance
AI 理解论文
溯源树
样例
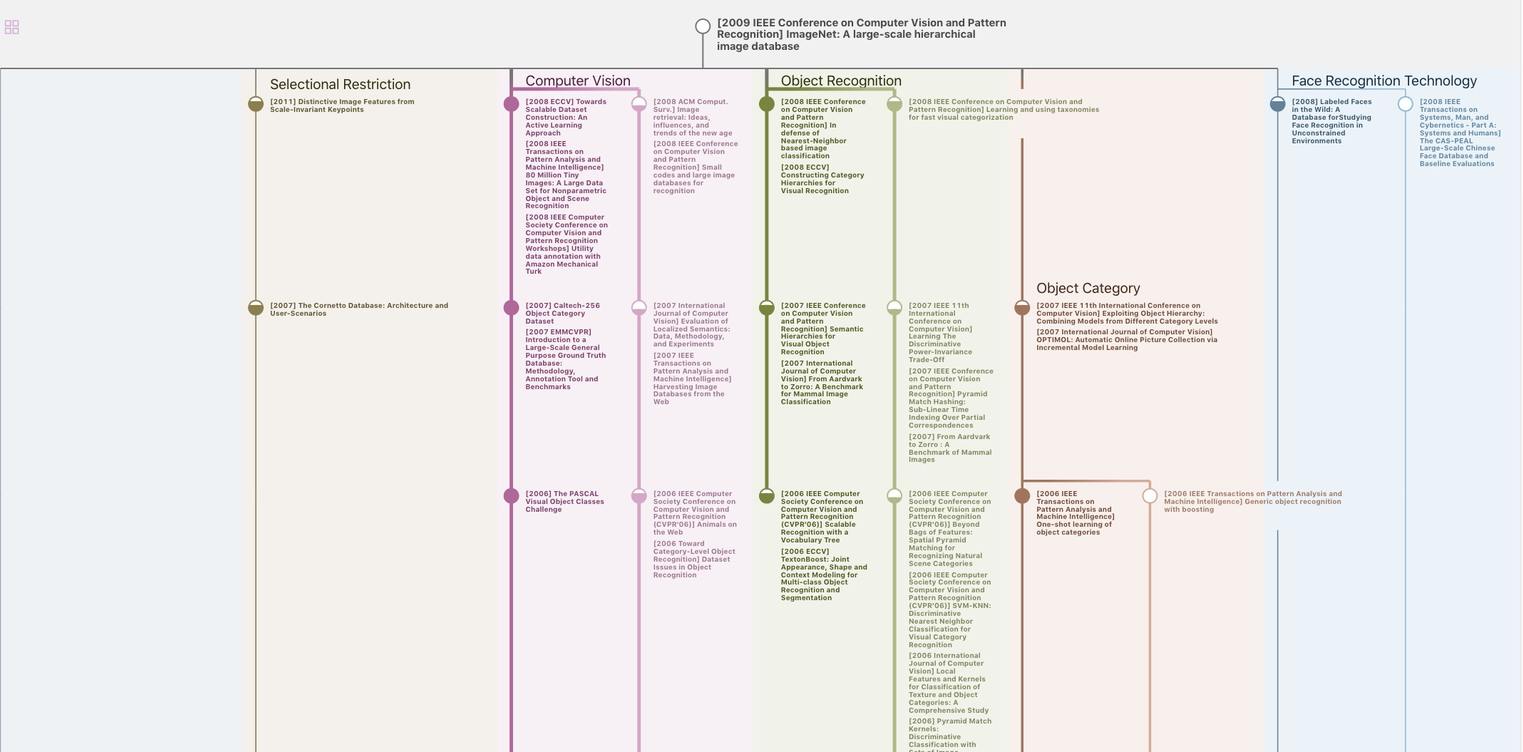
生成溯源树,研究论文发展脉络
Chat Paper
正在生成论文摘要