Quantum generative adversarial imitation learning
New Journal of Physics(2023)
摘要
Investigating quantum advantage in the NISQ era is a challenging problem whereas quantum machine learning becomes the most promising application that can be resorted to. However, no proposal has been investigated for arguably challenging inverse reinforcement learning to demonstrate the potential advantage. In this work, we propose a hybrid quantum–classical inverse reinforcement learning algorithm based on the variational quantum circuit with the generative adversarial framework. We find an important connection between the quantum gradient anomaly and the performance degradation, which suggest a gradient clipping strategy to stabilize the training process. In light of the algorithm, we study three classic control problems and the Hamiltonian parameter estimation in quantum sensing with shallow quantum circuits. The numerical results showcase that the control-enhanced quantum sensor can saturate quantum Cramér-Rao bound only with a single variational layer, empirically demonstrating a parameter complexity advantage over the classical learning control. The proposed generative adversarial reinforcement learning algorithm achieves state-of-the-art performance in classical and quantum sensor control in terms of required number of parameters.
更多查看译文
关键词
quantum machine learning,quantum sensing,quantum control
AI 理解论文
溯源树
样例
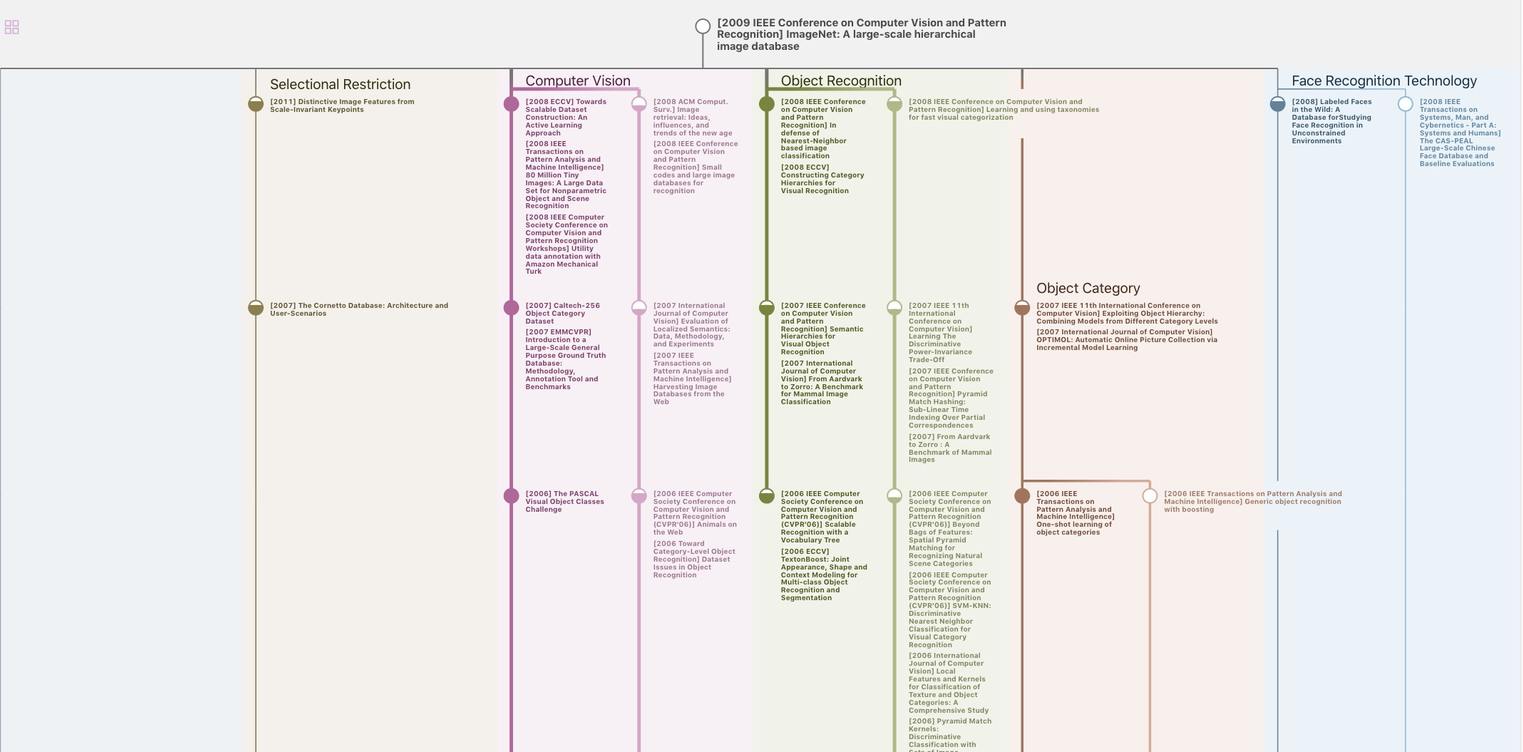
生成溯源树,研究论文发展脉络
Chat Paper
正在生成论文摘要