Deep Learning Initialized Compressed Sensing (Deli-CS) in Volumetric Spatio-Temporal Subspace Reconstruction.
bioRxiv : the preprint server for biology(2023)
摘要
Introduction:Spatio-temporal MRI methods enable whole-brain multi-parametric mapping at ultra-fast acquisition times through efficient k-space encoding, but can have very long reconstruction times, which limit their integration into clinical practice. Deep learning (DL) is a promising approach to accelerate reconstruction, but can be computationally intensive to train and deploy due to the large dimensionality of spatio-temporal MRI. DL methods also need large training data sets and can produce results that don't match the acquired data if data consistency is not enforced. The aim of this project is to reduce reconstruction time using DL whilst simultaneously limiting the risk of deep learning induced hallucinations, all with modest hardware requirements.
Methods:Deep Learning Initialized Compressed Sensing (Deli-CS) is proposed to reduce the reconstruction time of iterative reconstructions by "kick-starting" the iterative reconstruction with a DL generated starting point. The proposed framework is applied to volumetric multi-axis spiral projection MRF that achieves whole-brain T1 and T2 mapping at 1-mm isotropic resolution for a 2-minute acquisition. First, the traditional reconstruction is optimized from over two hours to less than 40 minutes while using more than 90% less RAM and only 4.7 GB GPU memory, by using a memory-efficient GPU implementation. The Deli-CS framework is then implemented and evaluated against the above reconstruction.
Results:Deli-CS achieves comparable reconstruction quality with 50% fewer iterations bringing the full reconstruction time to 20 minutes.
Conclusion:Deli-CS reduces the reconstruction time of subspace reconstruction of volumetric spatio-temporal acquisitions by providing a warm start to the iterative reconstruction algorithm.
更多查看译文
关键词
compressed sensing,deep learning,spatio-temporal
AI 理解论文
溯源树
样例
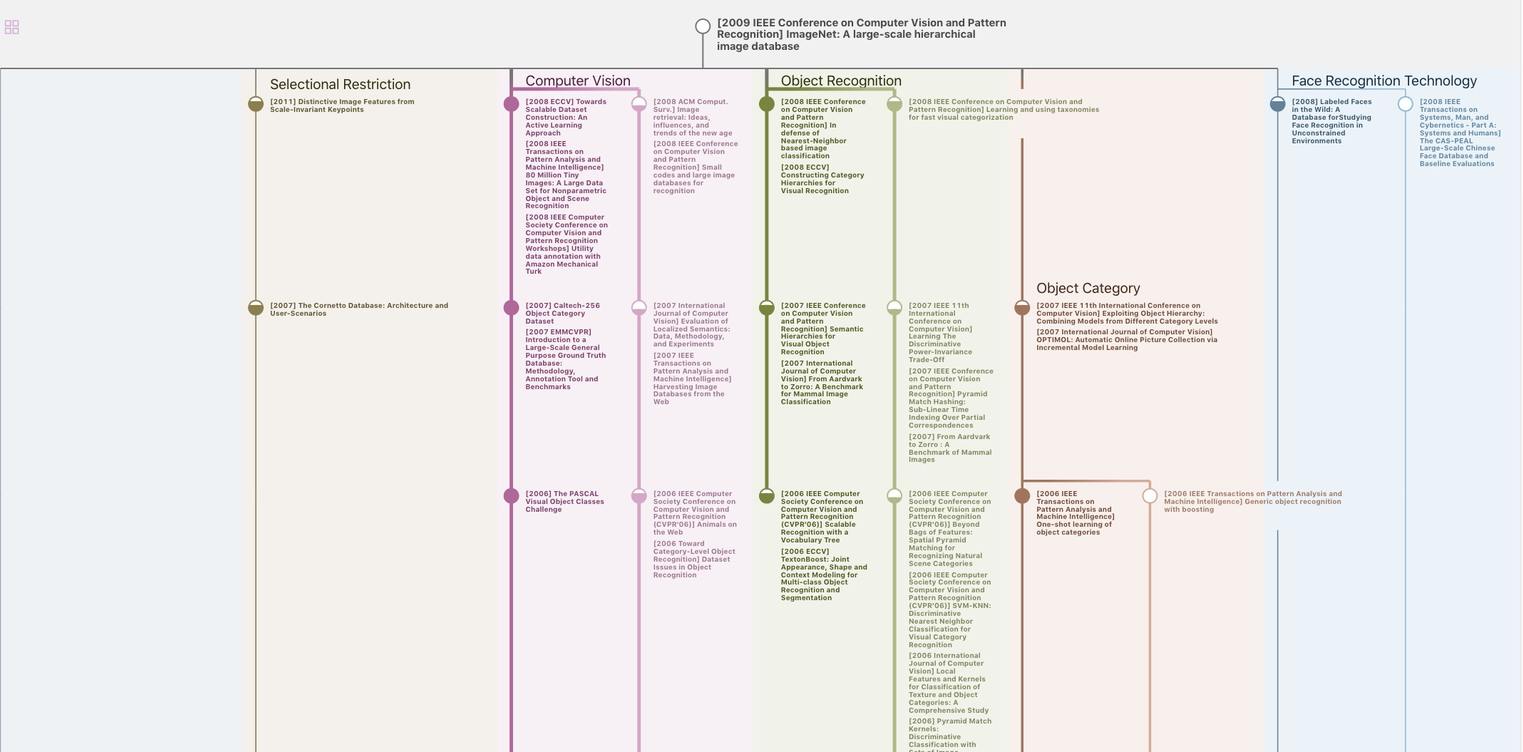
生成溯源树,研究论文发展脉络
Chat Paper
正在生成论文摘要