Physical–statistical learning in resilience assessment for power generation systems
Physica A: Statistical Mechanics and its Applications(2023)
摘要
Upswing in extreme weather conditions and natural disasters in conjunction with the relentless penetration of the intermittent renewable energy have brought resilience of the power generation systems into sharp relief. In this study, we adopt a high-order physical model to characterize the full-detail sub-transient behaviors in synchronous generator dynamics, and consequently utilize basin stability (BS) to quantify system resilience against potentially large perturbations. This high-fidelity model has not been extensive probed in estimate of BS, largely owing to the tremendous computational overhead involved. We conduct sensitivity analysis to pick out the most critical system states, whose perturbation exerts huge impact and hence are sensitive on BS or system resilience. Following this, we develop a diversity-enhanced active learning framework to sequentially identify the informative perturbed states, which will be further evaluated by the high-fidelity sub-transient model. This approach only incurs a paltry of simulation effort compared to the crude Monte Carlo simulation but with comparable accuracy on BS estimation.
更多查看译文
关键词
Basin stability,Sensitivity analysis,Surrogate,Active learning
AI 理解论文
溯源树
样例
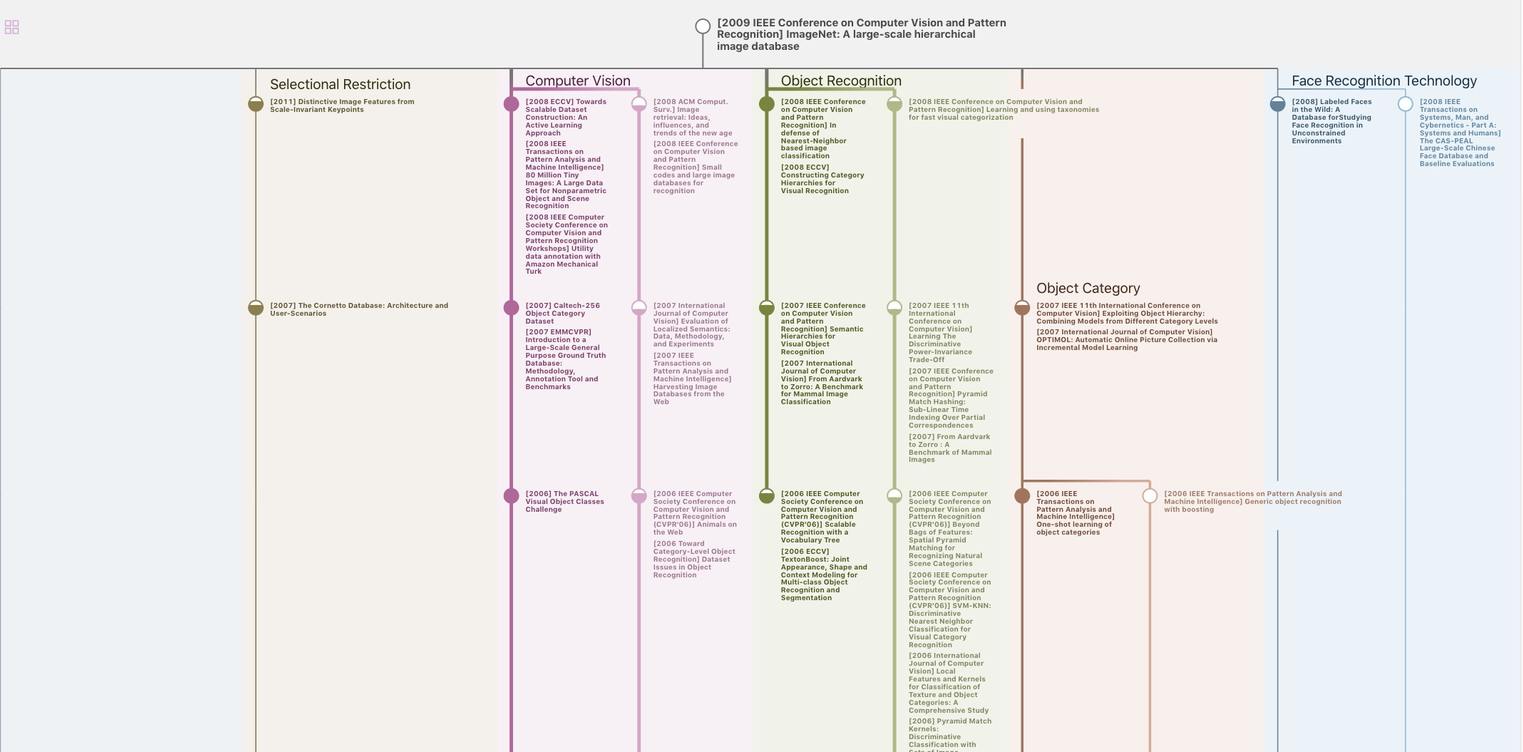
生成溯源树,研究论文发展脉络
Chat Paper
正在生成论文摘要