Risk ratio, odds ratio, risk difference... Which causal measure is easier to generalize?
arxiv(2023)
摘要
There are many measures to report so-called treatment or causal effects:
absolute difference, ratio, odds ratio, number needed to treat, and so on. The
choice of a measure, eg absolute versus relative, is often debated because it
leads to different impressions of the benefit or risk of a treatment. Besides,
different causal measures may lead to various treatment effect heterogeneity:
some input variables may have an influence on some causal measures and no
effect at all on others. In addition some measures - but not all - have
appealing properties such as collapsibility, matching the intuition of a
population summary. In this paper, we first review common causal measures and
their pros and cons typically brought forward. Doing so, we clarify the notions
of collapsibility and treatment effect heterogeneity, unifying existing
definitions. Then, we show that for any causal measures there exists a
generative model such that the conditional average treatment effect (CATE)
captures the treatment effect. However, only the risk difference can
disentangle the treatment effect from the baseline at both population and
strata levels, regardless of the outcome type (continuous or binary). As our
primary goal is the generalization of causal measures, we show that different
sets of covariates are needed to generalize an effect to a target population
depending on (i) the causal measure of interest, and (ii) the identification
method chosen, that is generalizing either conditional outcome or local
effects.
更多查看译文
AI 理解论文
溯源树
样例
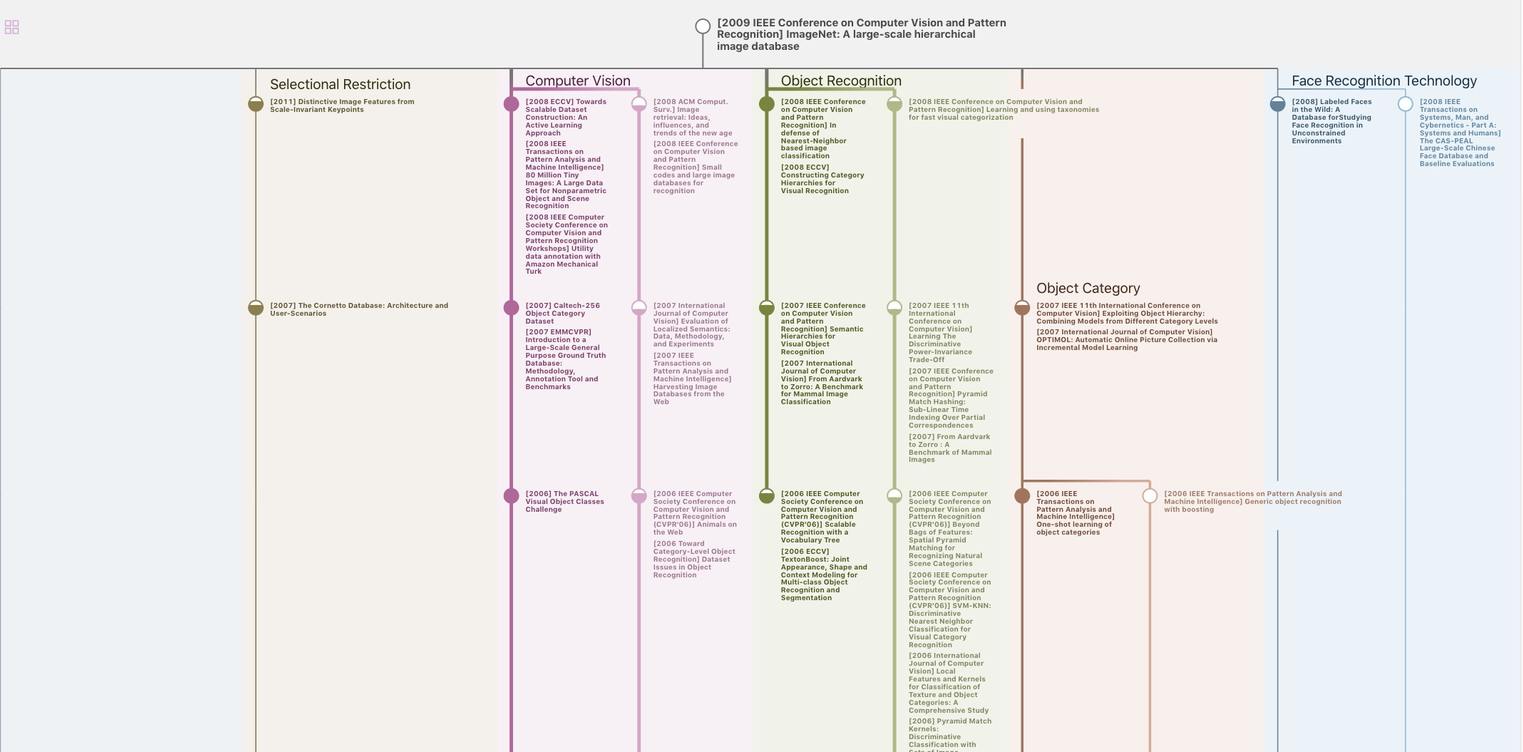
生成溯源树,研究论文发展脉络
Chat Paper
正在生成论文摘要