Probabilistic modeling of long-term joint wind and wave load conditions via generative adversarial network
Stochastic Environmental Research and Risk Assessment(2023)
摘要
Offshore structures, such as oil and gas platforms and offshore wind turbines, are subjected to wind and wave loads simultaneously during their service lifetime. Since the wind and wave states are of significant randomness and dependence, the probabilistic modeling of joint wind and wave conditions plays an essential role in the safety design of offshore structures. Currently, three different methods can be adopted to establish the joint probabilistic model, which, however, are somewhat inconvenient in applications. The recently emerged generative adversarial networks has been demonstrated to be effective in dealing with high-dimensional random variables in several fields. In this study, the implicit joint probabilistic model of joint wind and wave load conditions is developed based on the Wasserstein generative adversarial network with gradient penalty. Long-term metocean reanalysis data of the site in the South China Sea is used to train and validate the model. After one million training steps, high-quality samples that are quite similar to the original data can be generated by the developed model. In addition, statistical comparisons of the generated samples obtained by the C-vine copula approach and the developed generative adversarial network model are performed as well, which demonstrates the effectiveness and superiority of the developed model.
更多查看译文
关键词
Joint wind and wave conditions,Offshore structures,Joint probabilistic modeling,Deep learning,Generative adversarial network
AI 理解论文
溯源树
样例
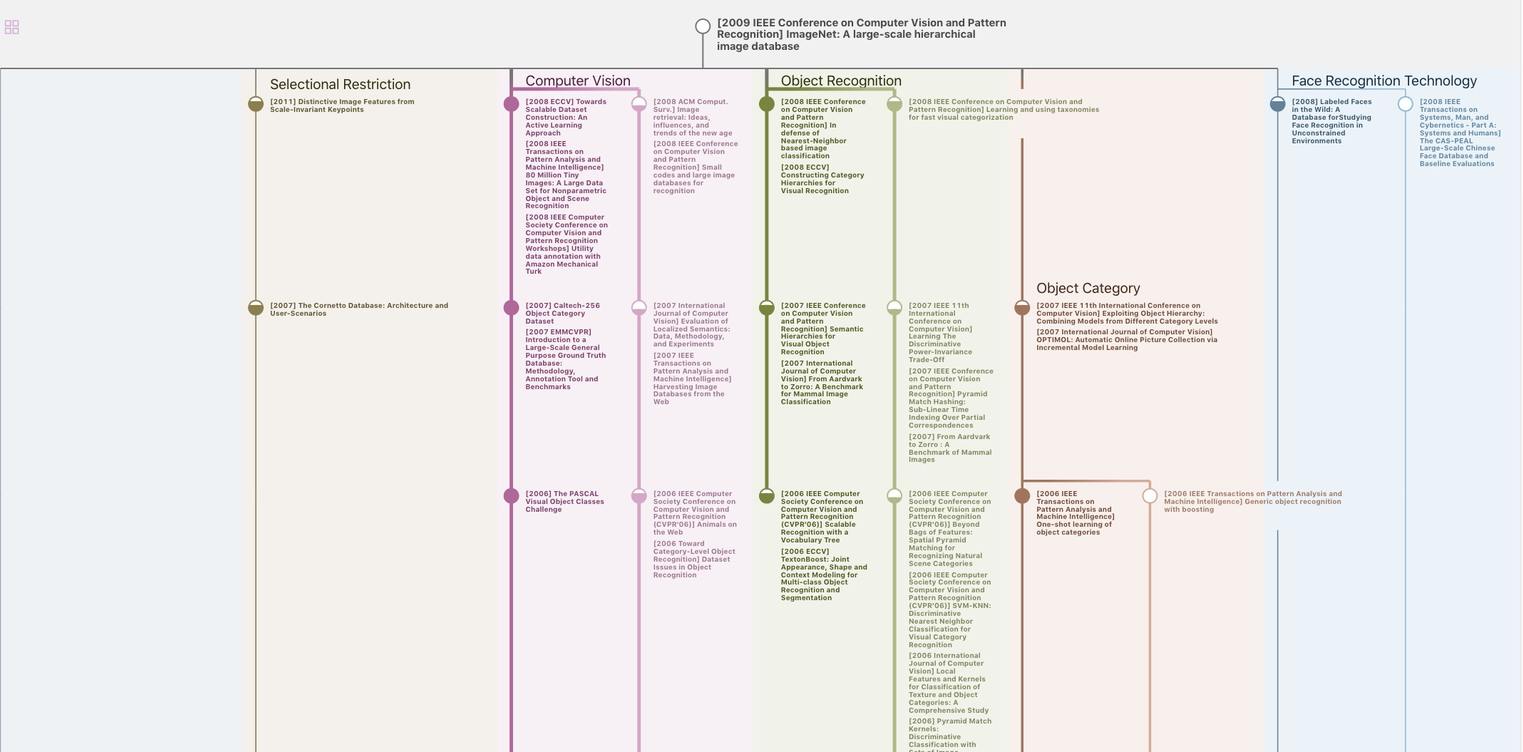
生成溯源树,研究论文发展脉络
Chat Paper
正在生成论文摘要