Learning Perceptual Position-Aware Shapelets for Time Series Classification
ECML/PKDD (6)(2023)
摘要
Shapelets are time series subsequences that effectively distinguish time series classes. Recently, time series classifiers based on shapelets have gained interest from the community thanks to their high accuracy and interpretable results. However, these shapelet-based methods still have some problems in both shapelet initialization and learning shapelet phases that limit their performances. In this paper, we propose a novel shapelet-based classifier, called Perceptual Position-aware Shapelet Network (PPSN), to effectively discover and optimize the shapelets. Our method effectively utilizes the perceptually important points to extract a small number of high-quality shapelet candidates and leverages the position-aware subsequence distance for evaluating these candidates. In the learning shapelet phase, our model applied the fixed normalization on each shapelet’s transformed values to address the negative impact of their different value ranges. It also uses the stop-gradient connection in the first few epochs to reduce the unwelcome effect of the non-optimal weights of the final linear layer. Experimental results on 112 UCR datasets demonstrate that our model is state-of-the-art compared to existing non-ensemble methods and competitive with the current most accurate classifier, HIVE-COTE 2.0, while retaining the advantage of low computational time and the power of interpretation.
更多查看译文
关键词
time series,classification,position-aware
AI 理解论文
溯源树
样例
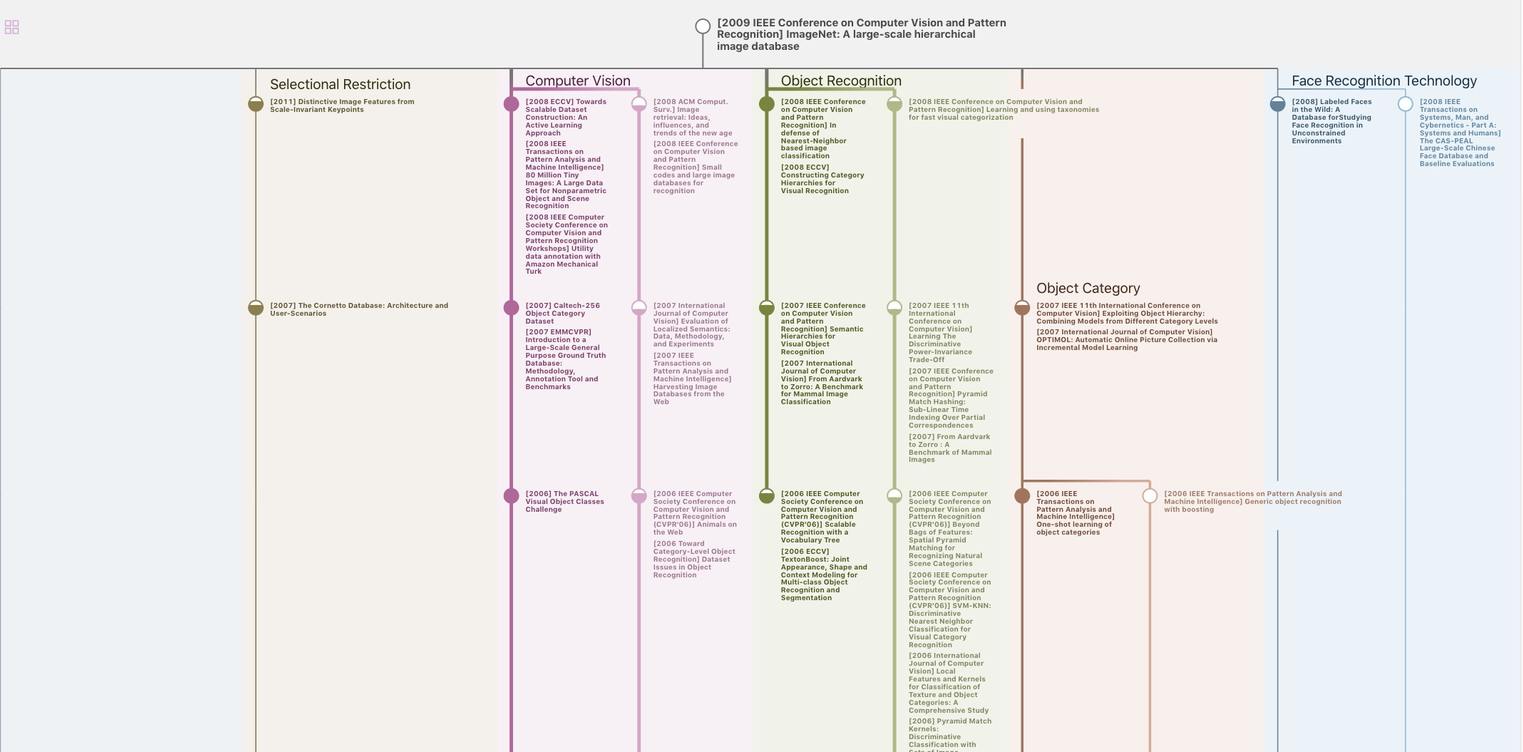
生成溯源树,研究论文发展脉络
Chat Paper
正在生成论文摘要