Deep unified attention-based sequence modeling for online anomalous trajectory detection
Future Generation Computer Systems(2023)
摘要
The continued development of GPS-enabled equipment and location-based services gives rise to the omnipresence of trajectory data. A trajectory is defined as a timestamped location sequence of a moving object. It is of great importance to enable a variety of data analysis algorithms regarding big trajectory data. In particular, anomalous trajectory detection is a fundamental functionality in spatial–temporal data mining and urban computing. Existing methods use single grid embeddings to represent trajectory points, while the adjacency of grids is ignored. In addition, current models consider the importance of each point in a trajectory to be equal, which is inconsistent with real-world applications. To solve these problems, we propose a Deep Unified Attention-based Sequence Modeling for Online Anomalous Trajectory Detection (UA-OATD). Our UA-OATD model unifies embeddings of mapped grids and embeddings of their adjacency grids, an attention mechanism is employed to extract trajectory features. Once the training is done, the UA-OATD model detects anomalous trajectories by calculating the anomaly score of target trajectories in an online manner. Extensive experiments on two real large-scale datasets demonstrate that our solution consistently outperforms existing state-of-the-art methods. Meanwhile, the high detection efficiency is capable of meeting real-time detection requirements.
更多查看译文
关键词
Anomalous trajectory detection,Sequence modeling,Attention mechanism
AI 理解论文
溯源树
样例
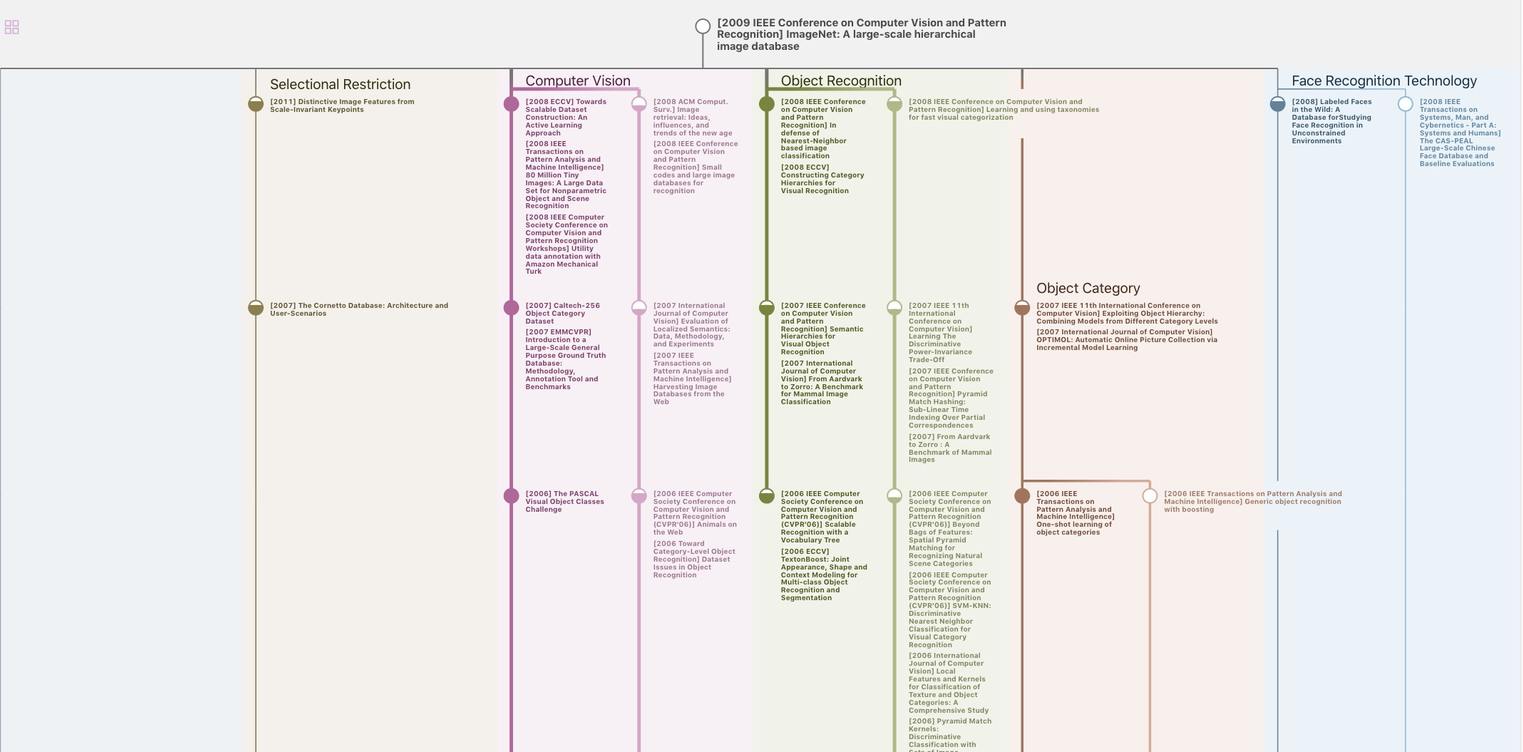
生成溯源树,研究论文发展脉络
Chat Paper
正在生成论文摘要