CT-free quantitative SPECT for automatic evaluation of %thyroid uptake based on deep-learning
EJNMMI physics(2023)
摘要
Purpose Quantitative thyroid single-photon emission computed tomography/computed tomography (SPECT/CT) requires computed tomography (CT)-based attenuation correction and manual thyroid segmentation on CT for %thyroid uptake measurements. Here, we aimed to develop a deep-learning-based CT-free quantitative thyroid SPECT that can generate an attenuation map (μ-map) and automatically segment the thyroid. Methods Quantitative thyroid SPECT/CT data ( n = 650) were retrospectively analyzed. Typical 3D U-Nets were used for the μ-map generation and automatic thyroid segmentation. Primary emission and scattering SPECTs were inputted to generate a μ-map, and the original μ-map from CT was labeled (268 and 30 for training and validation, respectively). The generated μ-map and primary emission SPECT were inputted for the automatic thyroid segmentation, and the manual thyroid segmentation was labeled (280 and 36 for training and validation, respectively). Other thyroid SPECT/CT ( n = 36) and salivary SPECT/CT ( n = 29) were employed for verification. Results The synthetic μ-map demonstrated a strong correlation ( R 2 = 0.972) and minimum error (mean square error = 0.936 × 10 −4 , %normalized mean absolute error = 0.999%) of attenuation coefficients when compared to the ground truth ( n = 30). Compared to manual segmentation, the automatic thyroid segmentation was excellent with a Dice similarity coefficient of 0.767, minimal thyroid volume difference of − 0.72 mL, and a short 95% Hausdorff distance of 9.416 mm ( n = 36). Additionally, %thyroid uptake by synthetic μ-map and automatic thyroid segmentation (CT-free SPECT) was similar to that by the original μ-map and manual thyroid segmentation (SPECT/CT) (3.772 ± 5.735% vs. 3.682 ± 5.516%, p = 0.1090) ( n = 36). Furthermore, the synthetic μ-map generation and automatic thyroid segmentation were successfully performed in the salivary SPECT/CT using the deep-learning algorithms trained by thyroid SPECT/CT ( n = 29). Conclusion CT-free quantitative SPECT for automatic evaluation of %thyroid uptake can be realized by deep-learning.
更多查看译文
关键词
Quantification,Single-photon emission computed tomography,Deep-learning,Attenuation correction,Segmentation
AI 理解论文
溯源树
样例
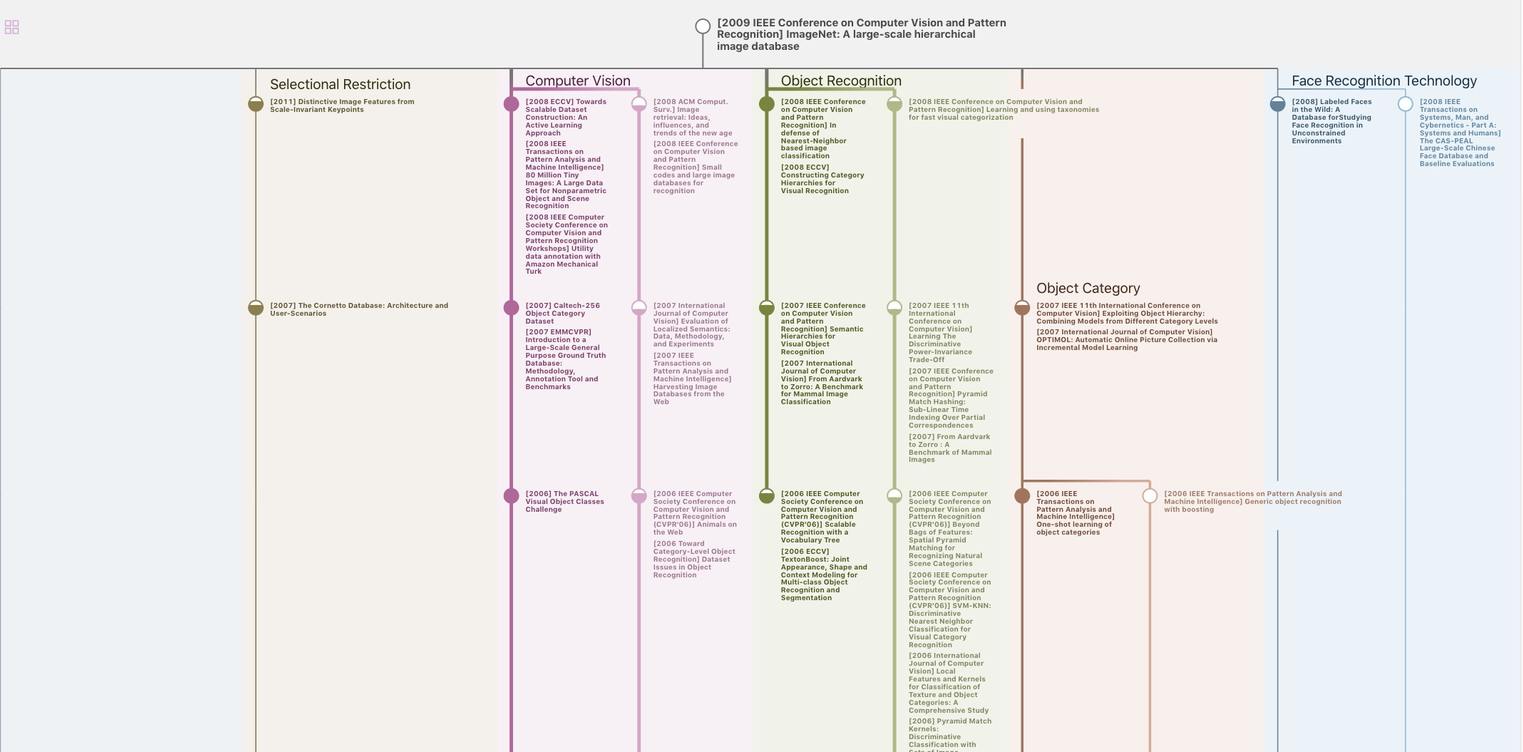
生成溯源树,研究论文发展脉络
Chat Paper
正在生成论文摘要