Wasserstein Adversarial Examples on Univariant Time Series Data
CoRR(2023)
摘要
Adversarial examples are crafted by adding indistinguishable perturbations to normal examples in order to fool a well-trained deep learning model to misclassify. In the context of computer vision, this notion of indistinguishability is typically bounded by $L_{\infty}$ or other norms. However, these norms are not appropriate for measuring indistinguishiability for time series data. In this work, we propose adversarial examples in the Wasserstein space for time series data for the first time and utilize Wasserstein distance to bound the perturbation between normal examples and adversarial examples. We introduce Wasserstein projected gradient descent (WPGD), an adversarial attack method for perturbing univariant time series data. We leverage the closed-form solution of Wasserstein distance in the 1D space to calculate the projection step of WPGD efficiently with the gradient descent method. We further propose a two-step projection so that the search of adversarial examples in the Wasserstein space is guided and constrained by Euclidean norms to yield more effective and imperceptible perturbations. We empirically evaluate the proposed attack on several time series datasets in the healthcare domain. Extensive results demonstrate that the Wasserstein attack is powerful and can successfully attack most of the target classifiers with a high attack success rate. To better study the nature of Wasserstein adversarial example, we evaluate a strong defense mechanism named Wasserstein smoothing for potential certified robustness defense. Although the defense can achieve some accuracy gain, it still has limitations in many cases and leaves space for developing a stronger certified robustness method to Wasserstein adversarial examples on univariant time series data.
更多查看译文
关键词
examples
AI 理解论文
溯源树
样例
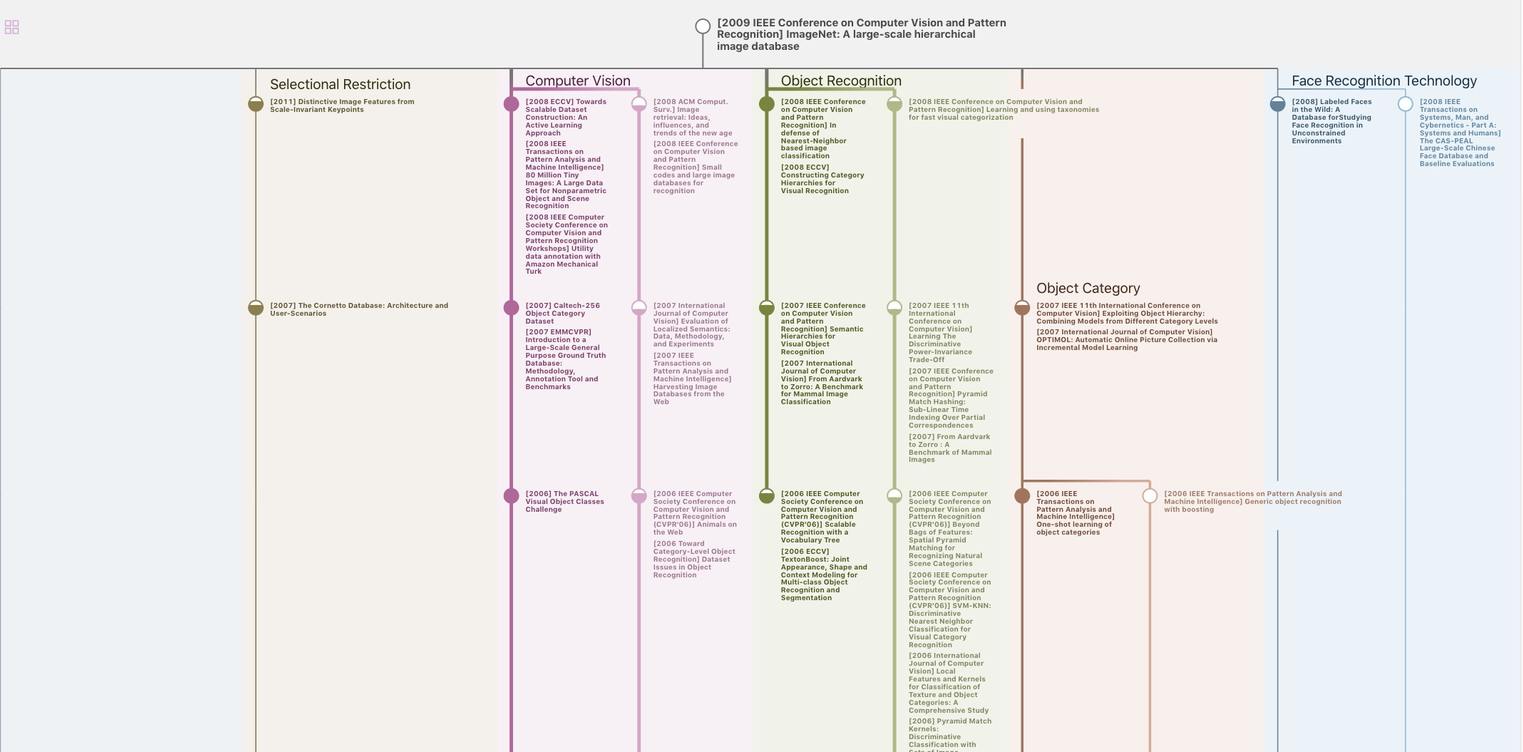
生成溯源树,研究论文发展脉络
Chat Paper
正在生成论文摘要