Material twins generation of woven polymer composites based on ResL-U-Net convolutional neural networks
Composite Structures(2023)
摘要
Using digital material twins reveals the fabric architectures of woven composites and details the morphological features of fiber tows and defects, which makes a unique approach to accessing the mechanical performance of woven composites, especially the damage and failure behaviors. However, the current major challenges are accurately and efficiently segmenting low-contrast digital images, such as mu CT images or MRI images, and reconstructing 3D braided structures. In this paper, a ResL-U-Net convolutional neural network is proposed, in which we use the leaky-ReLU activation function to improve efficiency. We use the residual structure to prevent network degradation and obtain excellent robustness and accuracy. The initial manufacturing defects are implanted into the finite element model by three-dimensional spatial mapping, which ensures the authenticity of defects on the one hand and high-quality mesh on the other. The damage evolution and fracture features of the GFRP material are investigated based on digital material twins; simultaneously, an in-situ CT tensile experiment is tailored to verify the simulations. It is shown that the simulations by the material twins demonstrated the mechanical performances of the GFRP very well, especially on the damage locations and material failure patterns.
更多查看译文
关键词
Polymer-matrix composites (PMCs),Deep learning,CT image segmentation,Finite element analysis (FEA),Digital material twins
AI 理解论文
溯源树
样例
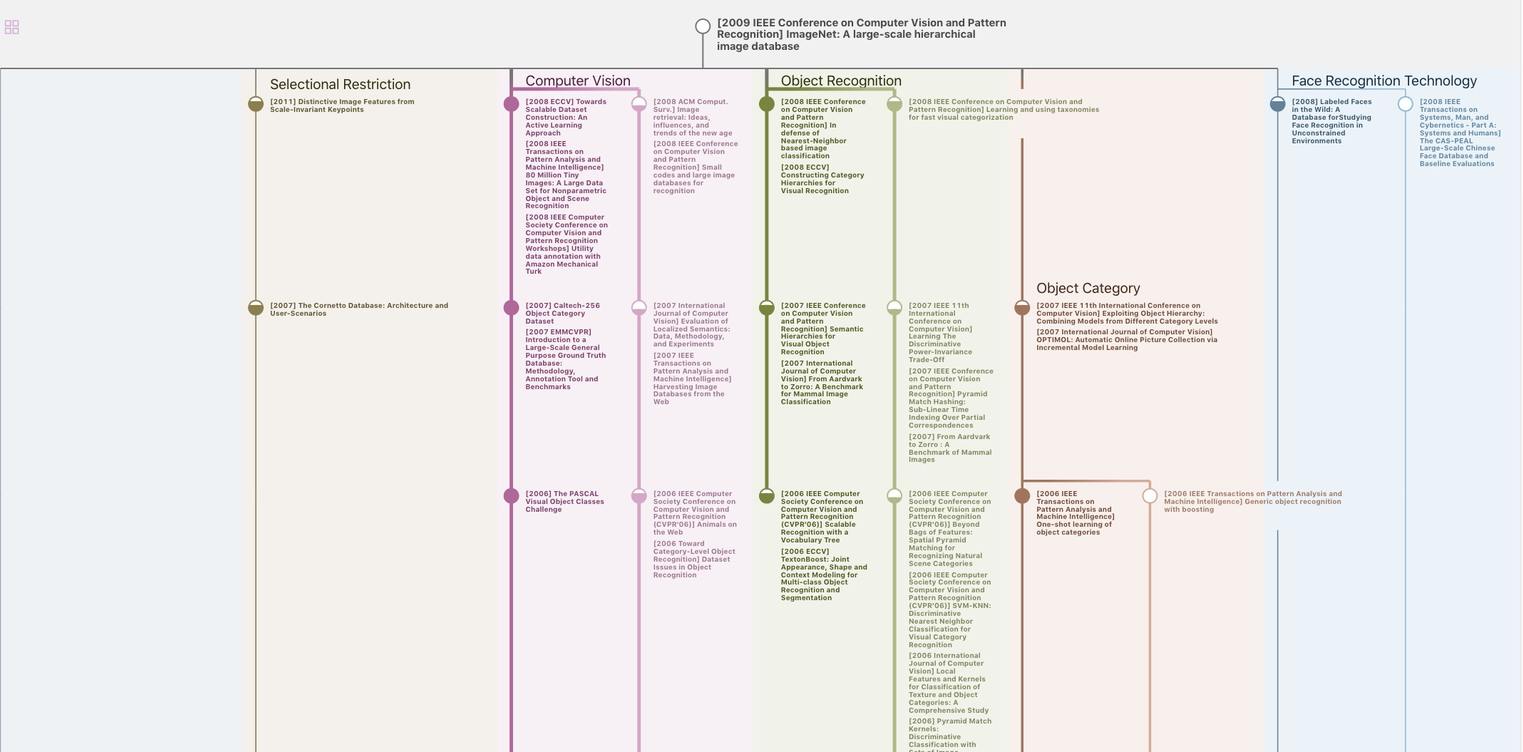
生成溯源树,研究论文发展脉络
Chat Paper
正在生成论文摘要