Accurate prediction of transcriptional activity of single missense variants in HIV Tat with deep learning
biorxiv(2023)
摘要
Tat is an essential gene for increasing the transcription of all HIV genes, and it affects HIV replication, HIV exit from latency, and AIDS progression. The Tat gene frequently mutates in vivo producing variants with diverse activities, contributing to HIV viral heterogeneity, as well as drug-resistant clones. Thus, identifying the transcriptional activities of Tat variants will help to better understand AIDS pathology and treatment. We recently reported the missense mutation landscape of all single amino acid Tat variants. In these experiments, a fraction of double missense alleles exhibited intragenic epistasis. It is too time-consuming and costly to determine a variants’ effect for all double mutant alleles with experiments. Therefore, we propose a combined GigaAssay/Deep learning approach. As a first step for determining activity landscapes for complex variants, we evaluated a deep learning framework using previously reported GigaAssay experiments to predict how transcription activity is affected by Tat variants with single missense substitutions. Our approach achieves a 0.94 Pearson correlation coefficient when comparing experimental to predicted activities. This hybrid approach should be extensible to more complex Tat alleles for better understanding the genetic control of HIV genome transcription.
### Competing Interest Statement
Martin R. Schiller and CJ Giacoletto are associated with Heligenics, a company pursuing commercial interests for the GigaAssay.
更多查看译文
关键词
variant effect,deep learning,HIV,tat protein
AI 理解论文
溯源树
样例
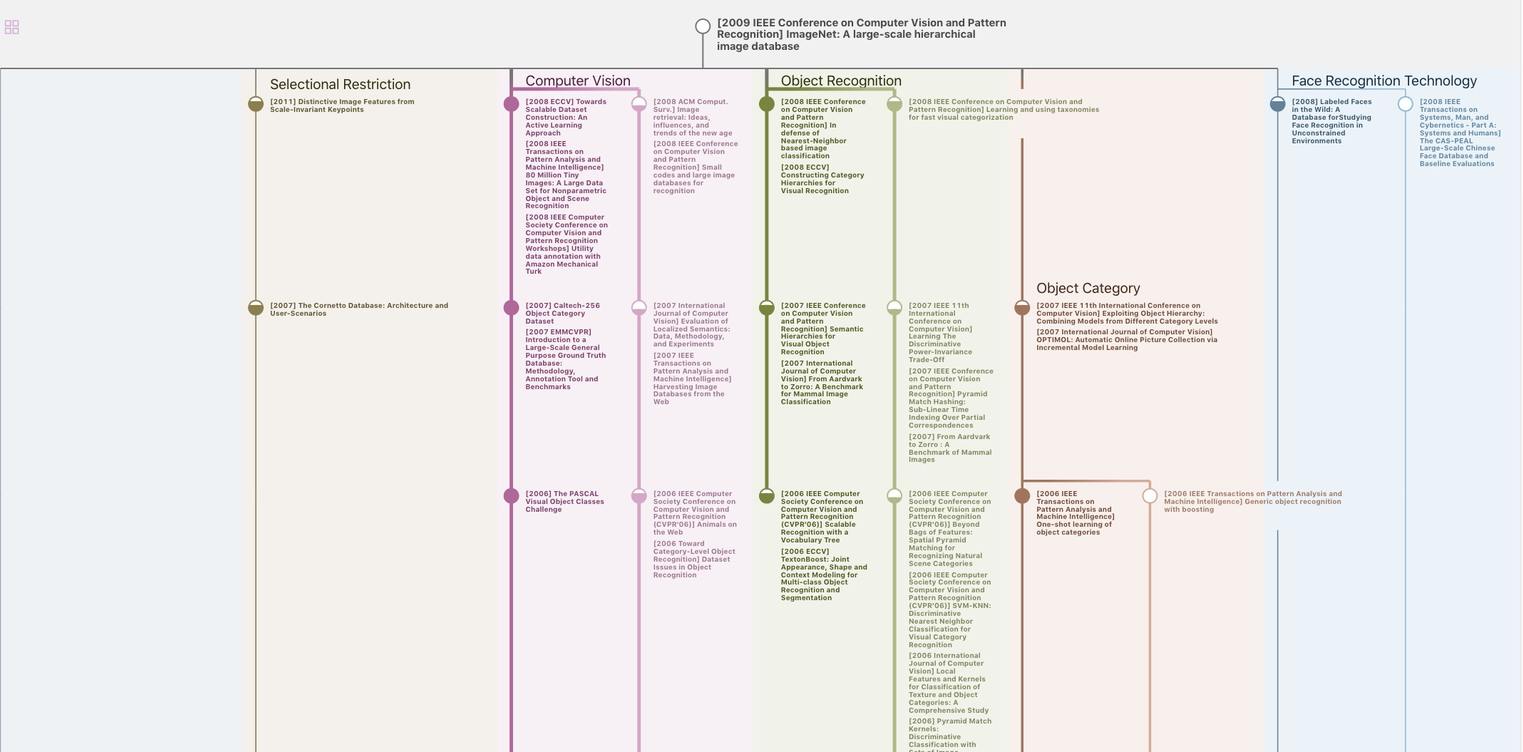
生成溯源树,研究论文发展脉络
Chat Paper
正在生成论文摘要