LRA&LDRA: Rethinking Residual Predictions for Efficient Shadow Detection and Removal
WACV(2023)
摘要
The majority of the state-of-the-art shadow removal models (SRMs) reconstruct whole input images, where their capacity is needlessly spent on reconstructing non-shadow regions. SRMs that predict residuals remedy this up to a degree, but fall short of providing an accurate and flexible solution. In this paper, we rethink residual predictions and propose Learnable Residual Attention (LRA) and Learnable Dense Reconstruction Attention (LDRA) modules, which operate over the input and the output of SRMs. These modules guide an SRM to concentrate on shadow region reconstruction, and limit reconstruction of non-shadow regions. The modules improve shadow removal (up to 20%) and detection accuracy across various backbones, and even improve the accuracy of other removal methods (up to 10%). In addition, the modules have minimal overhead (+<1MB memory) and are implemented in a few lines of code. Furthermore, to combat the challenge of training SRMs with small datasets, we present a synthetic dataset generation pipeline. Using our pipeline, we create a dataset called PITSA, which has 10 times more unique shadow-free images than the largest benchmark dataset. Pre-training models on the PITSA significantly improves shadow removal (+2 MAE on shadow regions) and detection accuracy of multiple methods. Our results show that LRA&LDRA, when plugged into a lightweight architecture pre-trained on the PITSA, outperform state-of-the-art shadow removal (+0.7 all-region MAE) and detection (+0.1 BER) methods on the benchmark ISTD and SRD datasets, despite running faster (+5%) and consuming less memory (x150).
更多查看译文
关键词
efficient shadow detection,residual predictions
AI 理解论文
溯源树
样例
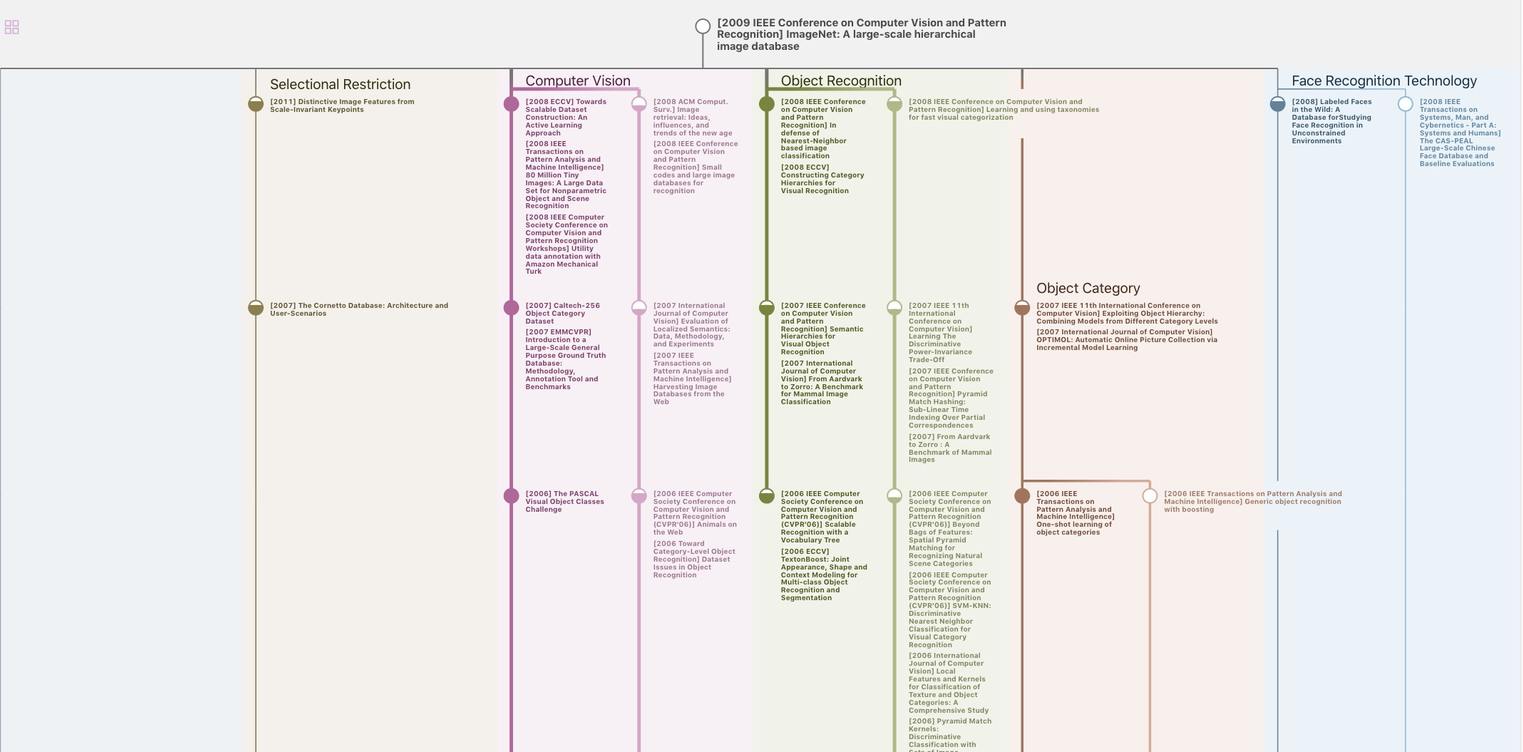
生成溯源树,研究论文发展脉络
Chat Paper
正在生成论文摘要