Feature Complement for Visual Tracking Based on Global Feature Comparison.
IEEE Access(2023)
摘要
Target tracking is one of the challenging tasks in computer vision. Usually, the center of target origins from the position with the largest response value, and the key to improving tracking performance is to learn reliable feature maps. This paper analyzes the characteristics of the tracking task, designs a global feature comparison function to extract the context, and proposes a feature supplement module based on the global comparison information for further performance improvement. In addition, we also design a template feature update module to supplement template features based on the search area features of the current frame to dynamically adjust model features, improve model generalization capabilities, and avoid model feature fixation. The proposed feature supplement model based on global feature comparison (FSGFC) is evaluated on five visual tracking benchmarks including OTB100, VOT2016, VOT2018, VOT2019 and UAV123. The experimental results show that the model obtains the state-of-the-art performance with a real-time speed.
更多查看译文
关键词
Feature extraction,Target tracking,Computer vision,Data mining,Computational modeling,Residual neural networks,Benchmark testing,Siamese network,feature extraction,template update
AI 理解论文
溯源树
样例
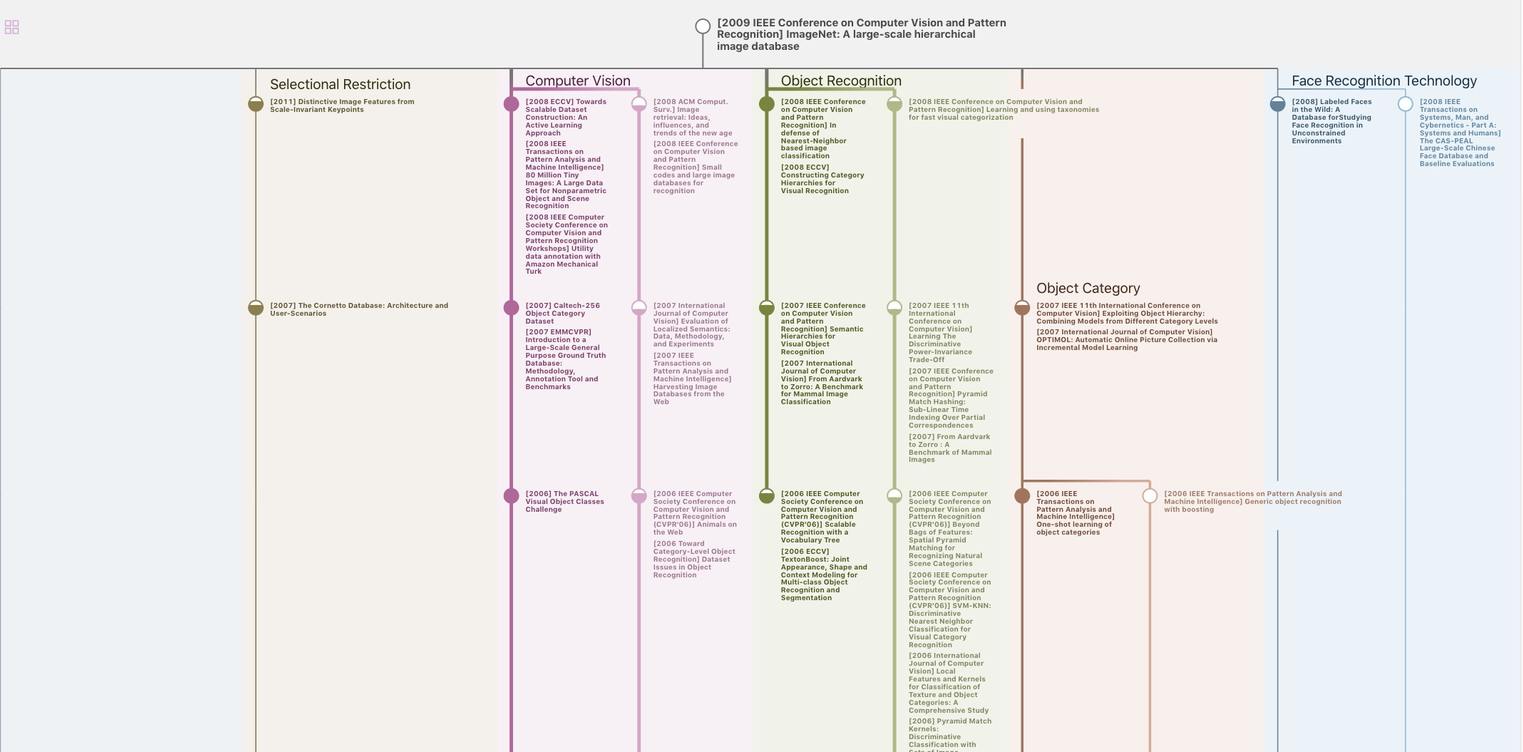
生成溯源树,研究论文发展脉络
Chat Paper
正在生成论文摘要