Internet Financial Credit Scoring Models Based on Deep Forest and Resampling Methods.
IEEE Access(2023)
摘要
In recent years, deep learning credit scoring models have become a hot research topic in Internet finance. However, most of the existing studies are based on deep neural network models, whose structure is difficult to design. Moreover, previous research seldom considers the impact of class imbalance problems on credit scoring performance. To fill this gap, we propose a new deep learning credit scoring model based on deep forest (DF) and resampling methods. First, we combine DF with five resampling methods including random over-sampling (ROS), random under-sampling (RUS), synthetic minority over-sampling technique (SMOTE), tomek links and SMOTE+ Tomek, respectively, to build responding models. We validate that the RUS-DF model has the best credit scoring performance among the above models. Then, to further evaluate the advantages of the deep ensemble model RUS-DF, we compare it with four models building by combining RUS with multilayer perceptron, convolutional neural network, and long short-term memory and random forests, respectively. All the experiments are conducted on four Internet financial credit scoring datasets. The results show that the RUS-DF model obtains better classification performance and stability than other models and is suitable for solving the credit scoring problem with imbalanced data.
更多查看译文
关键词
Internet,Deep learning,Random forests,Regression analysis,Data models,Finance,Credit scoring,class imbalance,deep forest,resampling method
AI 理解论文
溯源树
样例
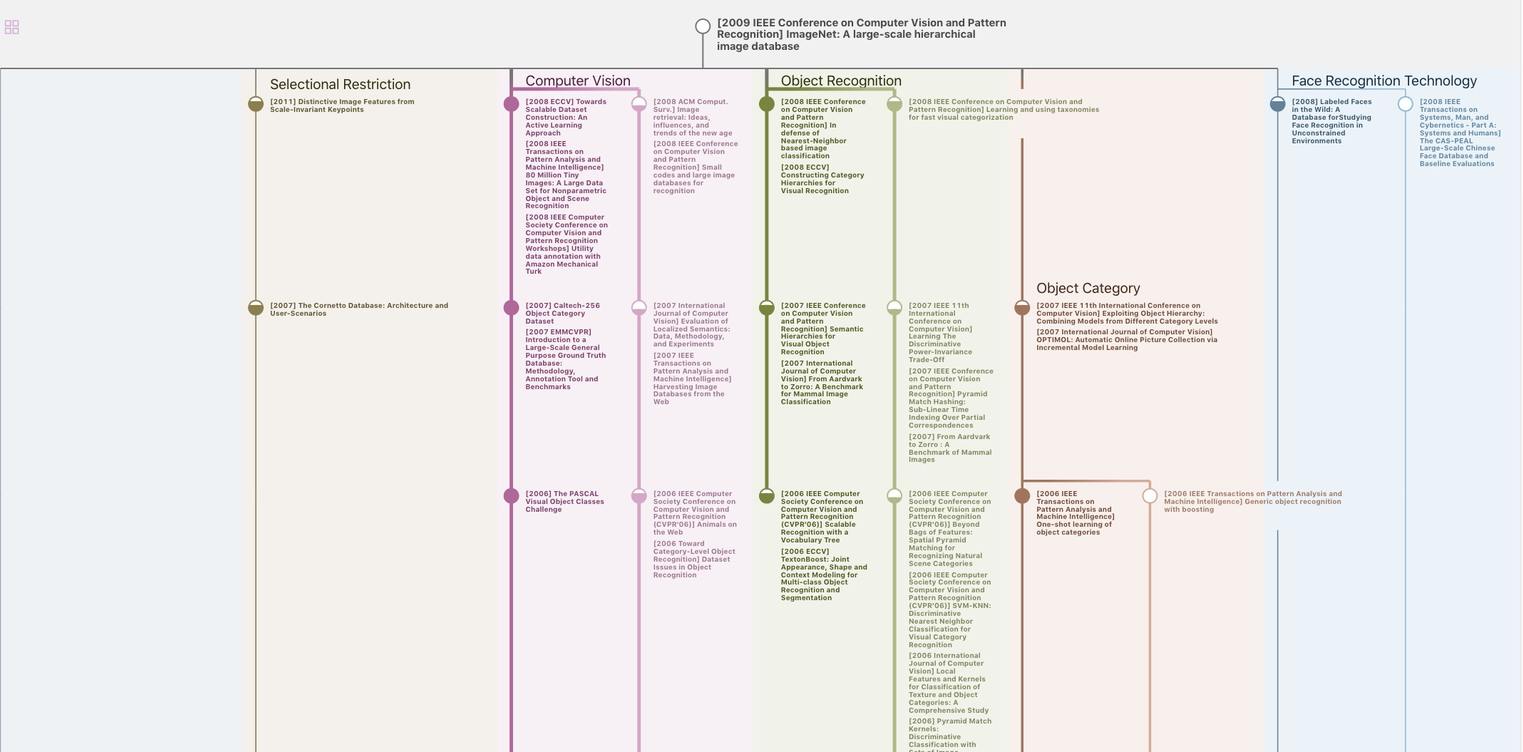
生成溯源树,研究论文发展脉络
Chat Paper
正在生成论文摘要