Privacy-Preserving Split Learning for Large-Scaled Vision Pre-Training.
IEEE Trans. Inf. Forensics Secur.(2023)
Abstract
The growing concerns about data privacy in society lead to restrictions on the computer vision research gradually. Several collaboration-based vision learning methods have recently emerged, e.g., federated learning and split learning. These methods protect user data from leaving local devices, and make training performed only by uploading gradients, parameters, or activations, etc. However, there is little research on collaborative learning based on state-of-the-art and large-scaled models, mainly due to the high computation or communication overheads of the latest models. Training these models may be still unrealized for users' terminals. In this paper, we make a first attempt at the sensitive image pre-training with large-scaled models in the collaborative learning scenario, and propose a new lightweight framework for split learning based on mask, Masked Split Learning (MaskSL). We further ensure its security by differential privacy. Besides, we model the computation and communication overheads of several collaborative learning approaches by deduction to illustrate advantages of our scheme. Finally, we design and conduct a series of experiments on real-world datasets, e.g., in face recognition and medical image classification tasks, to demonstrate the performance of MaskSL.
MoreTranslated text
Key words
Computational modeling,Training,Federated learning,Privacy,Data models,Transformers,Task analysis,Split learning,self pre-training,differential privacy,masked autoencoder
AI Read Science
Must-Reading Tree
Example
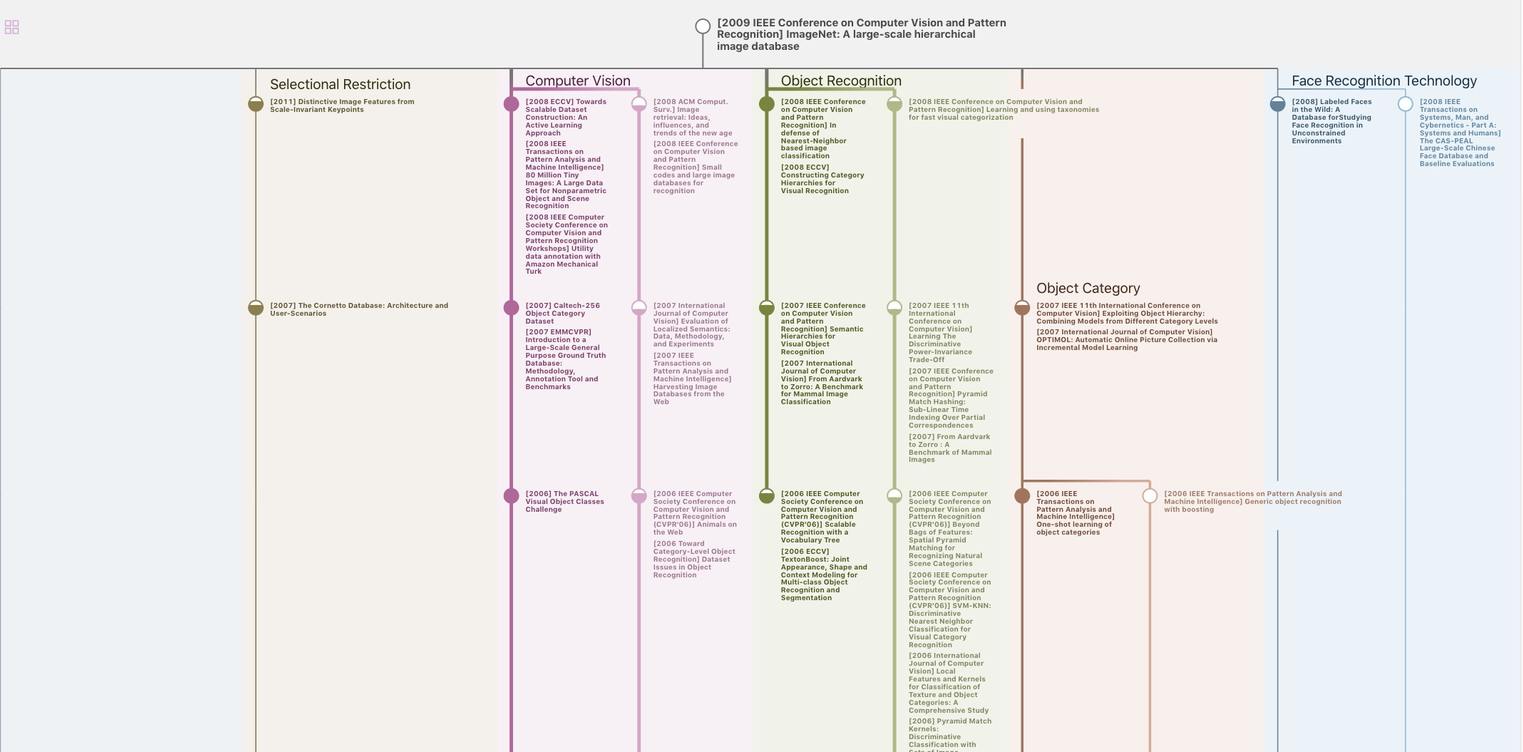
Generate MRT to find the research sequence of this paper
Chat Paper
Summary is being generated by the instructions you defined