Scaling Vision Transformers to 22 Billion Parameters
CoRR(2023)
摘要
The scaling of Transformers has driven breakthrough capabilities for language models. At present, the largest large language models (LLMs) contain upwards of 100B parameters. Vision Transformers (ViT) have introduced the same architecture to image and video modelling, but these have not yet been successfully scaled to nearly the same degree; the largest dense ViT contains 4B parameters (Chen et al., 2022). We present a recipe for highly efficient and stable training of a 22B-parameter ViT (ViT-22B) and perform a wide variety of experiments on the resulting model. When evaluated on downstream tasks (often with a lightweight linear model on frozen features), ViT-22B demonstrates increasing performance with scale. We further observe other interesting benefits of scale, including an improved tradeoff between fairness and performance, state-of-the-art alignment to human visual perception in terms of shape/texture bias, and improved robustness. ViT-22B demonstrates the potential for "LLM-like" scaling in vision, and provides key steps towards getting there.
更多查看译文
关键词
vision transformers,parameters
AI 理解论文
溯源树
样例
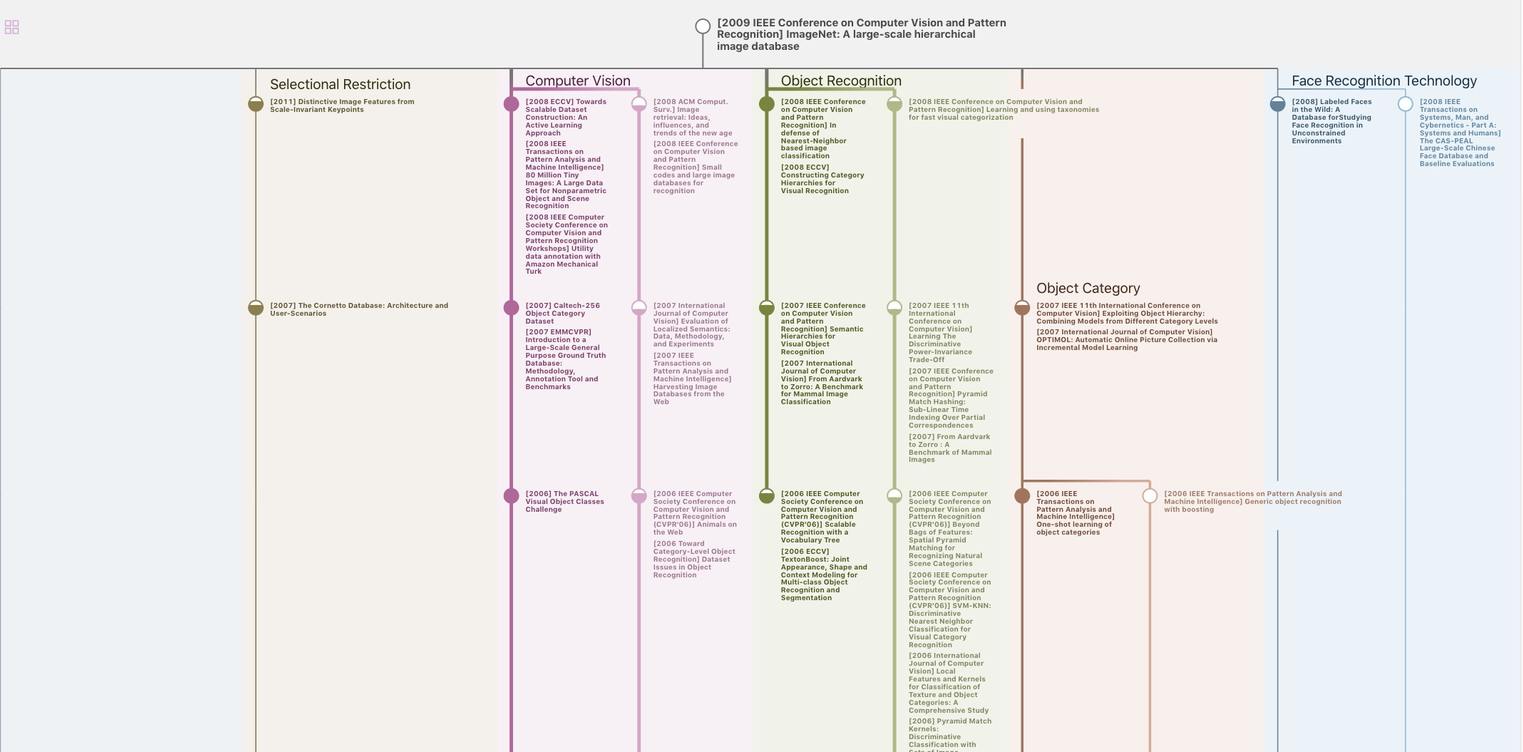
生成溯源树,研究论文发展脉络
Chat Paper
正在生成论文摘要