Oracle-Preserving Latent Flows.
CoRR(2023)
摘要
A fundamental task in data science is the discovery, description, and identification of any symmetries present in the data. We developed a deep learning methodology for the simultaneous discovery of multiple non-trivial continuous symmetries across an entire labeled dataset. The symmetry transformations and the corresponding generators are modeled with fully connected neural networks trained with a specially constructed loss function, ensuring the desired symmetry properties. The two new elements in this work are the use of a reduced-dimensionality latent space and the generalization to invariant transformations with respect to high-dimensional oracles. The method is demonstrated with several examples on the MNIST digit dataset, where the oracle is provided by the 10-dimensional vector of logits of a trained classifier. We find classes of symmetries that transform each image from the dataset into new synthetic images while conserving the values of the logits. We illustrate these transformations as lines of equal probability (“flows”) in the reduced latent space. These results show that symmetries in the data can be successfully searched for and identified as interpretable non-trivial transformations in the equivalent latent space.
更多查看译文
关键词
symmetry transformations,symmetry invariant,Lie groups,Lie algebras,supervised learning,deep learning
AI 理解论文
溯源树
样例
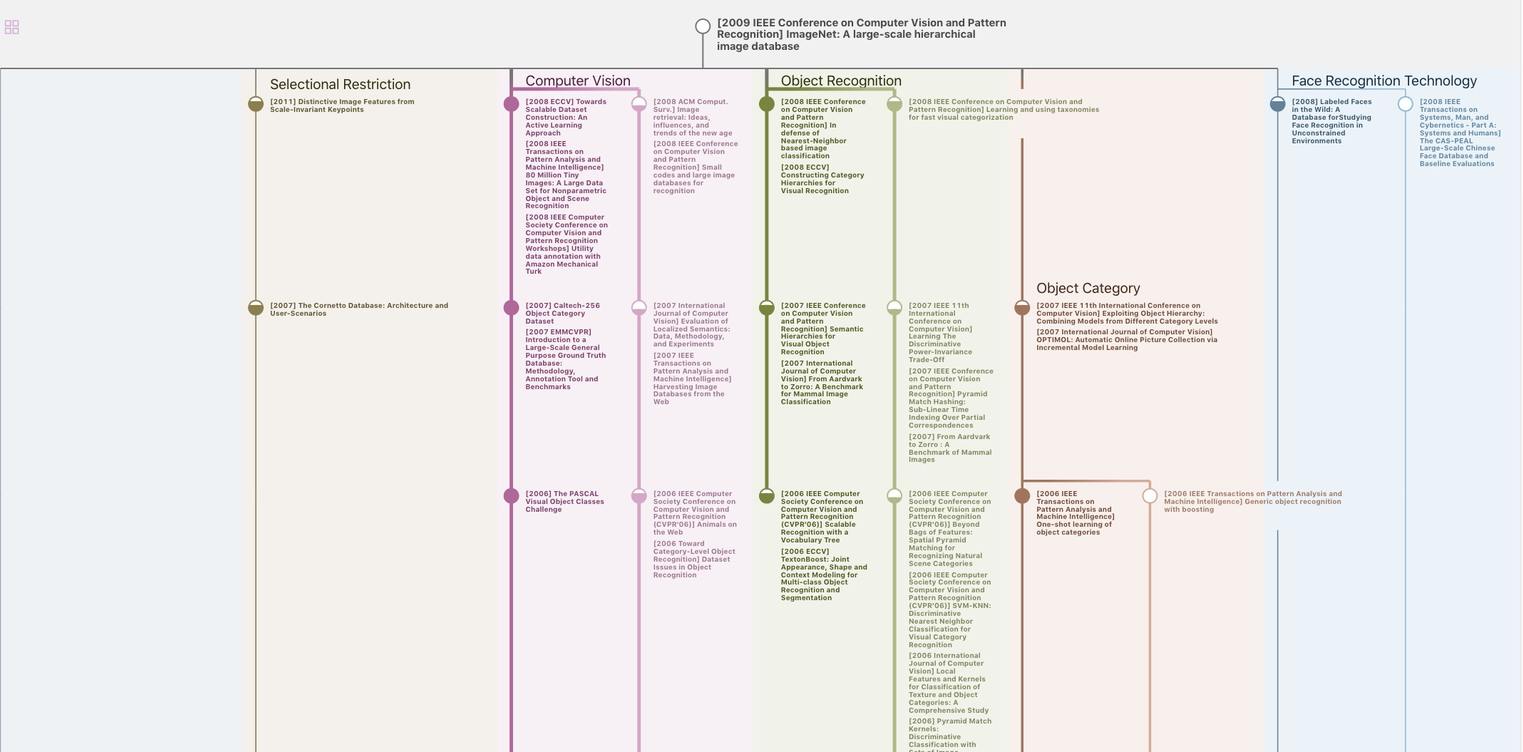
生成溯源树,研究论文发展脉络
Chat Paper
正在生成论文摘要