Real-Time Forecast of SMAP L3 Soil Moisture Using Spatial-Temporal Deep Learning Model with Data Integration.
Remote. Sens.(2023)
摘要
Soil moisture (SM) has significant impacts on the Earth's energy and water cycle system. Remote sensing, such as the Soil Moisture Active Passive (SMAP) mission, has delivered valuable estimations of global surface soil moisture. However, it has a 2 similar to 3 days revisit time leading to gaps between SMAP areas. To achieve accurate and comprehensive real-time forecast of SM, we propose a spatial-temporal deep learning model based on the Convolutional Gated Recursive Units with Data Integration (DI_ConvGRU) to capture the spatial and temporal variation in SM simultaneously by modeling the influence of adjacent SM values in space and time. Experiments show that the DI_ConvGRU outperforms the ConvGRU with Linear Interpolation (interp_ConvGRU) and the Long Short-Term Memory with Data Integration (DI_LSTM). The best performance (Bias = 0.0132 m(3)/m(3), ubRMSE = 0.022 m(3)/m(3), R = 0.977) has been achieved through the use of spatial-temporal deep learning model and Data Integration term. In comparison with interp_ConvGRU and DI_LSTM, DI_ConvGRU has improved the model performance in 74.88% and 68.99% of the regions according to RMSE, respectively. The predictability of SM depends highly on SM memory characteristics. DI_ConvGRU can provide accurate spatial-temporal forecast for SM with missing data, making them potentially useful for applications such as filling observational gaps in satellite data.
更多查看译文
关键词
soil moisture,deep learning,forecasting techniques,SMAP,remote sensing
AI 理解论文
溯源树
样例
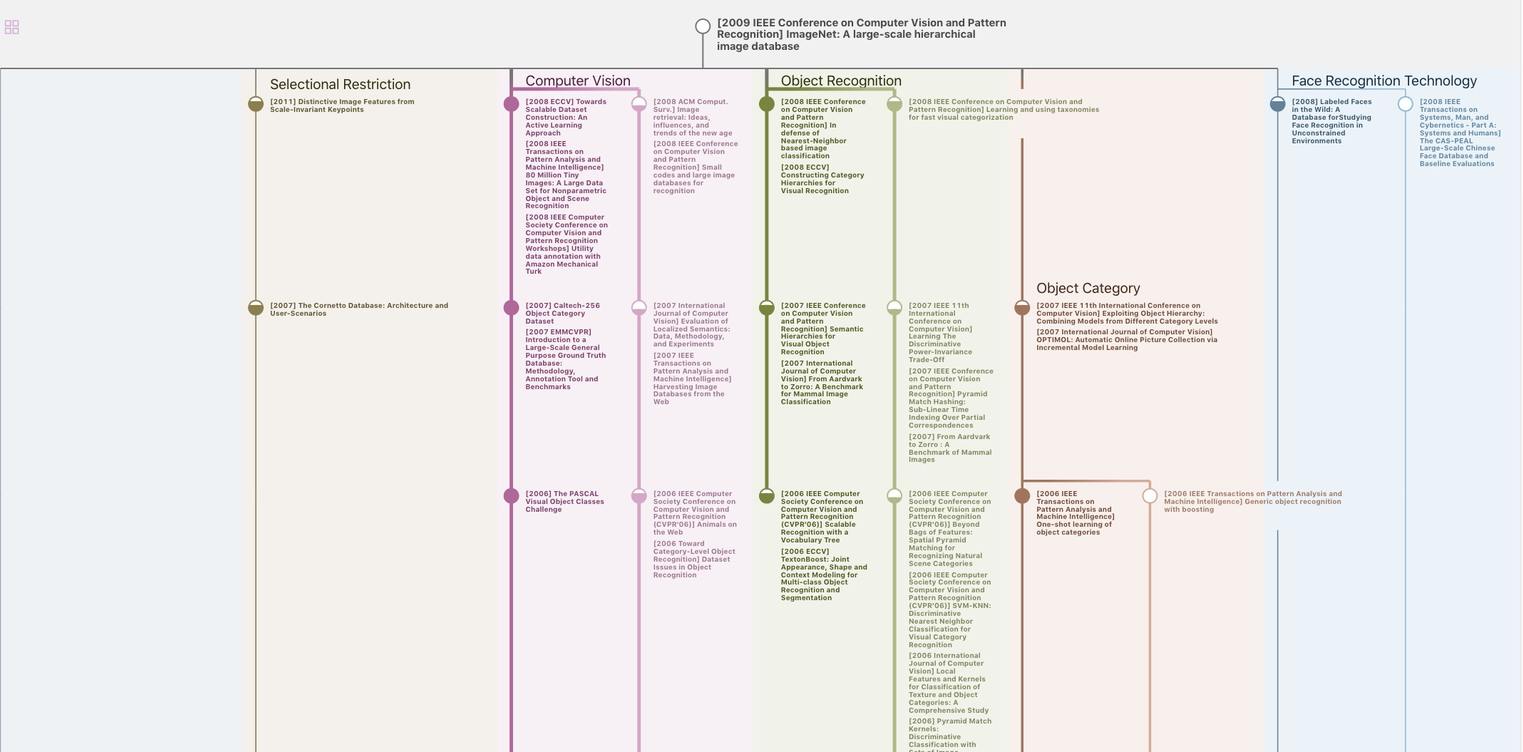
生成溯源树,研究论文发展脉络
Chat Paper
正在生成论文摘要