A similar structural and semantic integrated method for RDF entity embedding
Applied Intelligence(2023)
摘要
Resource Description Framework (RDF) graphs have become an important data source for many knowledge discovery algorithms and data mining tasks. However, most complex analyses that use knowledge discovery algorithms require data in a vector representation format. As a result, several RDF entity embedding techniques have emerged in which entities in the RDF graph are represented as low-dimensional vectors. These techniques generate sequences of entities using graph walk and use language modeling techniques to extract the feature from the sequences used to learn the embedding. However, sequences produced by graph walks only capture structural context; they are unable to capture latent context, such as semantically related information, which is an important property of RDF data. In this paper, we present a novel method that consists of a series of steps that generate sequences. These sequences not only capture structural context but also semantic property. The method for structural context includes (1) a new concept of similar entities in which tradeoffs are made between similar outgoing edges and outgoing nodes and (2) a new structural similarity, which calculates the similarity between two entities in each sequence. We can generate sequences based on structural similarity so that similar entities contain sequences with similar structures. The method for the semantic property combines sequences with the same semantic to generate latent sequences that cannot be generated by traversing the graph. This paper presents experimental results and a case study using real graphs to show that the proposed method outperforms existing methods in terms of quality and efficiency.
更多查看译文
关键词
RDF graph, Graph embeddings
AI 理解论文
溯源树
样例
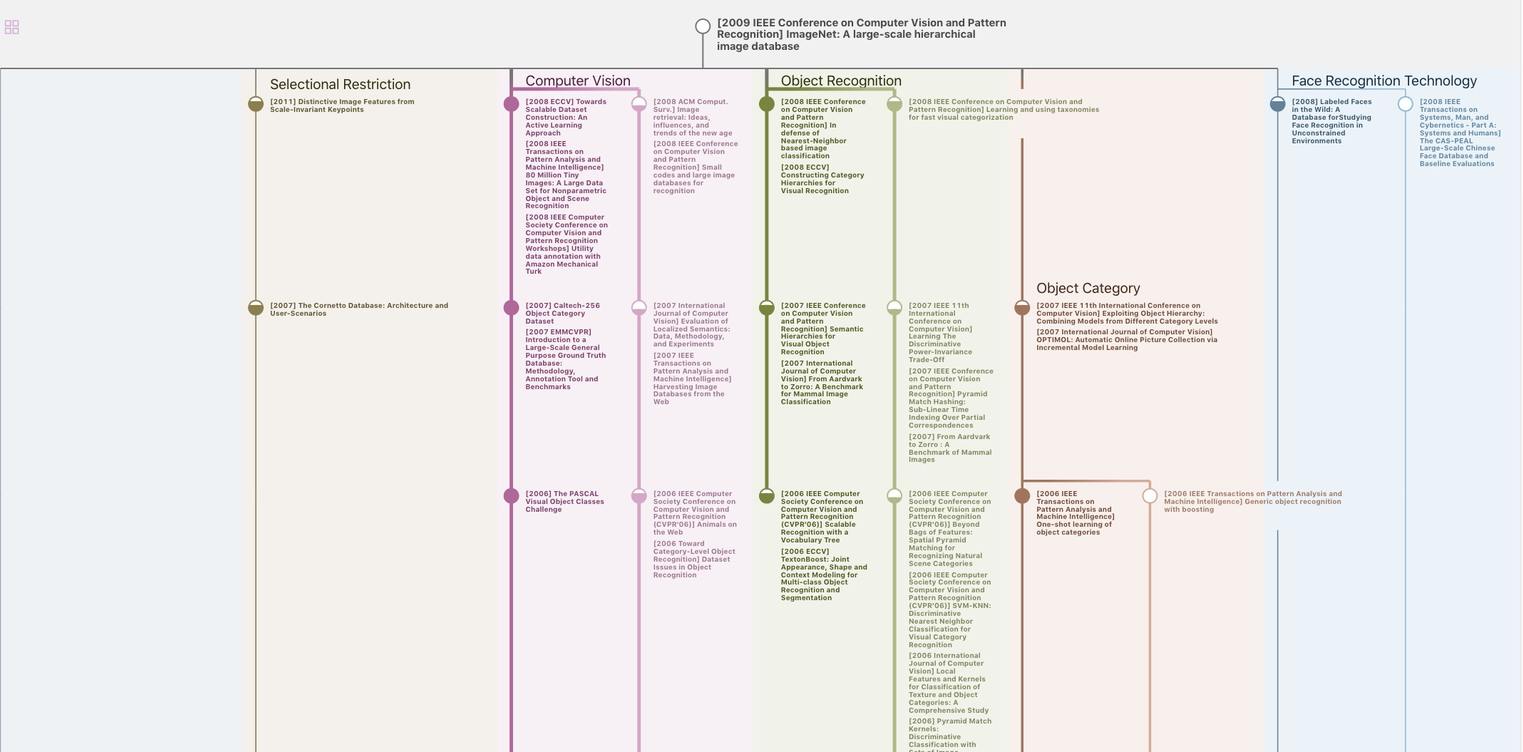
生成溯源树,研究论文发展脉络
Chat Paper
正在生成论文摘要