Variational Principles for Mirror Descent and Mirror Langevin Dynamics
arXiv (Cornell University)(2023)
摘要
Mirror descent, introduced by Nemirovski and Yudin in the 1970s, is a primal-dual convex optimization method that can be tailored to the geometry of the optimization problem at hand through the choice of a strongly convex potential function. It arises as a basic primitive in a variety of applications, including large-scale optimization, machine learning, and control. This paper proposes a variational formulation of mirror descent and of its stochastic variant, mirror Langevin dynamics. The main idea, inspired by the classic work of Brezis and Ekeland on variational principles for gradient flows, is to show that mirror descent emerges as a closed-loop solution for a certain optimal control problem, and the Bellman value function is given by the Bregman divergence between the initial condition and the global minimizer of the objective function.
更多查看译文
关键词
mirror langevin dynamics,mirror descent
AI 理解论文
溯源树
样例
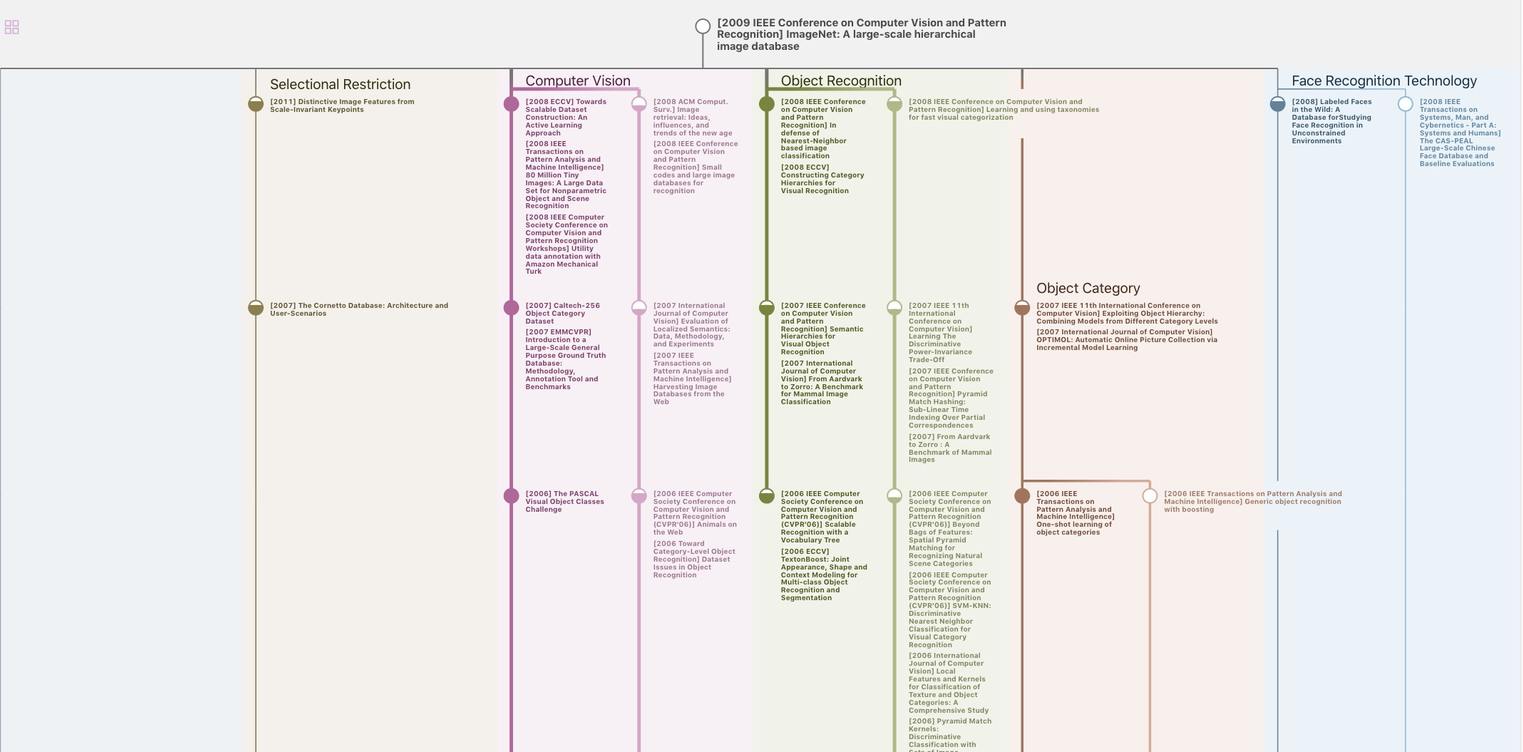
生成溯源树,研究论文发展脉络
Chat Paper
正在生成论文摘要