Real-time and effective detection of agricultural pest using an improved YOLOv5 network
Journal of Real-Time Image Processing(2023)
摘要
Pest detection and control can effectively guarantee the quality and yield of crops. The existing CNN-based object detection methods provide feasible strategies for pest detection; however, their low accuracy and speed have restricted the deployment and application in actual agricultural scenarios. In this paper, an improved YOLOv5 model for real-time and effective agricultural pest detection is proposed. First, a lightweight feature extraction network GhostNet is adopted as the backbone, and an efficient channel attention mechanism is introduced to enhance feature extraction. Then, high-resolution feature maps are added to the bidirectional feature pyramid network to enhance the data flow path of the neck, which enriches semantic information and highlights small pests. Furthermore, to assign dynamic weights to features at different receptive fields and highlight the unequal contributions of different receptive fields to global information, feature fusion with attentional multi-receptive fields is proposed. Finally, the experimental results on a large-scale public pest dataset (Pest24) demonstrate that our method exceeds the original YOLOv5 and other state-of-the-art models. The mAP improves from 71.5 to 74.1%, the detection speed improves from 79.4 FPS to 104.2 FPS, the FLOPs decrease by 42% and the model size is compressed by 39%. The proposed method enables real-time and effective pest detection.
更多查看译文
关键词
Deep learning,Pest detection,Precision agriculture,Feature pyramid,Feature fusion
AI 理解论文
溯源树
样例
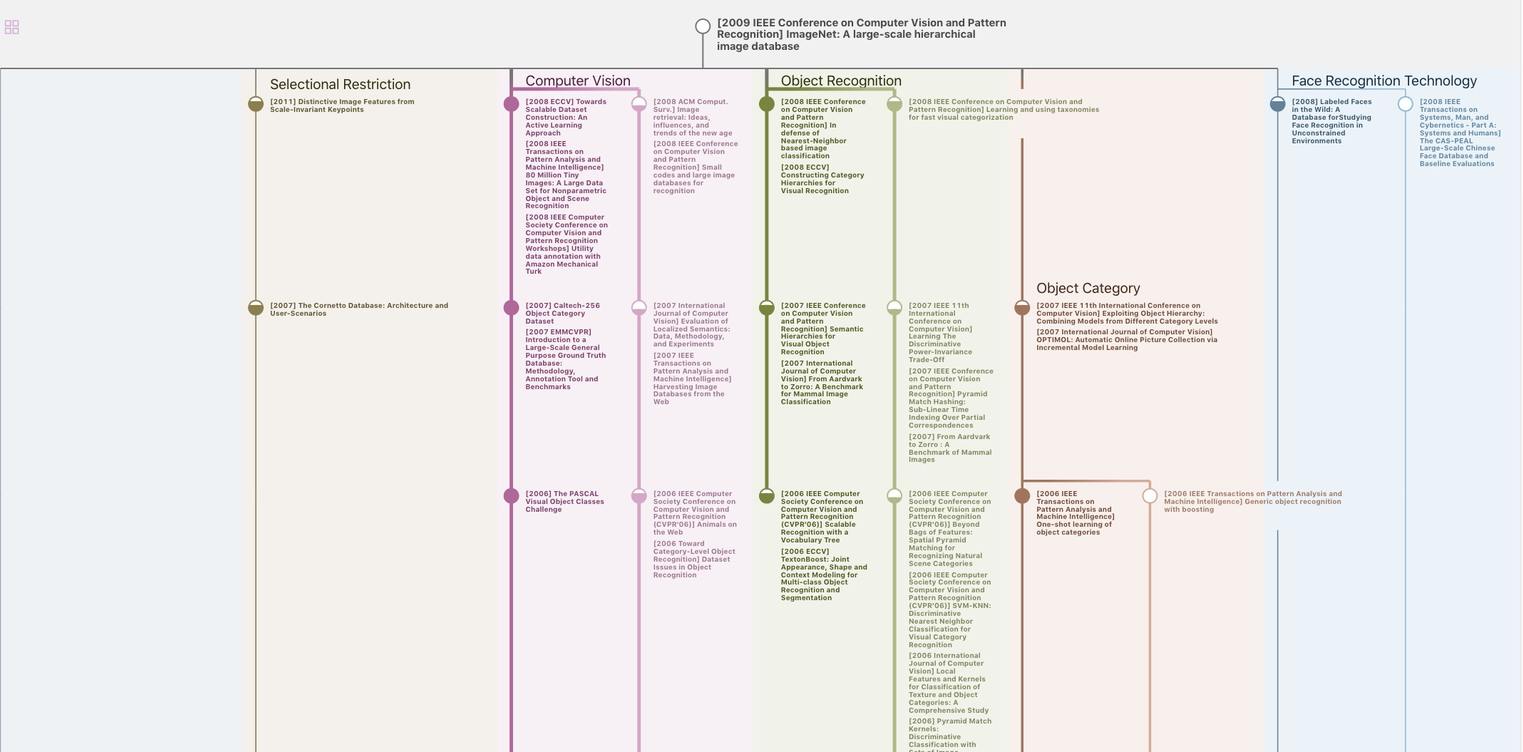
生成溯源树,研究论文发展脉络
Chat Paper
正在生成论文摘要