Lung Nodule Segmentation and Low-Confidence Region Prediction with Uncertainty-Aware Attention Mechanism
arxiv(2023)
摘要
Radiologists have different training and clinical experiences, which may result in various segmentation annotations for lung nodules, causing segmentation uncertainty. Conventional methods usually select a single annotation as the learning target or try to learn a latent space of various annotations, but these approaches waste the valuable information of consensus or disagreements ingrained in the multiple annotations. In this paper, we propose an Uncertainty-Aware Attention Mechanism (UAAM) that utilizes consensus and disagreements among multiple annotations to facilitate better segmentation. To achieve this, we introduce the Multi-Confidence Mask (MCM), which is a combination of a Low-Confidence (LC) Mask and a High-Confidence (HC) Mask. The LC mask indicates regions with a low segmentation confidence, which may cause different segmentation options among radiologists. Following UAAM, we further design an Uncertainty-Guide Segmentation Network (UGS-Net), which contains three modules: a Feature Extracting Module that captures a general feature of a lung nodule, an Uncertainty-Aware Module that produces three features for the annotations' union, intersection, and annotation set, and an Intersection-Union Constraining Module that uses distances between the three features to balance the predictions of final segmentation, LC mask, and HC mask. To fully demonstrate the performance of our method, we propose a Complex Nodule Validation on LIDC-IDRI, which tests UGS-Net's segmentation performance on lung nodules that are difficult to segment using U-Net. Experimental results demonstrate that our method can significantly improve the segmentation performance on nodules with poor segmentation by U-Net.
更多查看译文
AI 理解论文
溯源树
样例
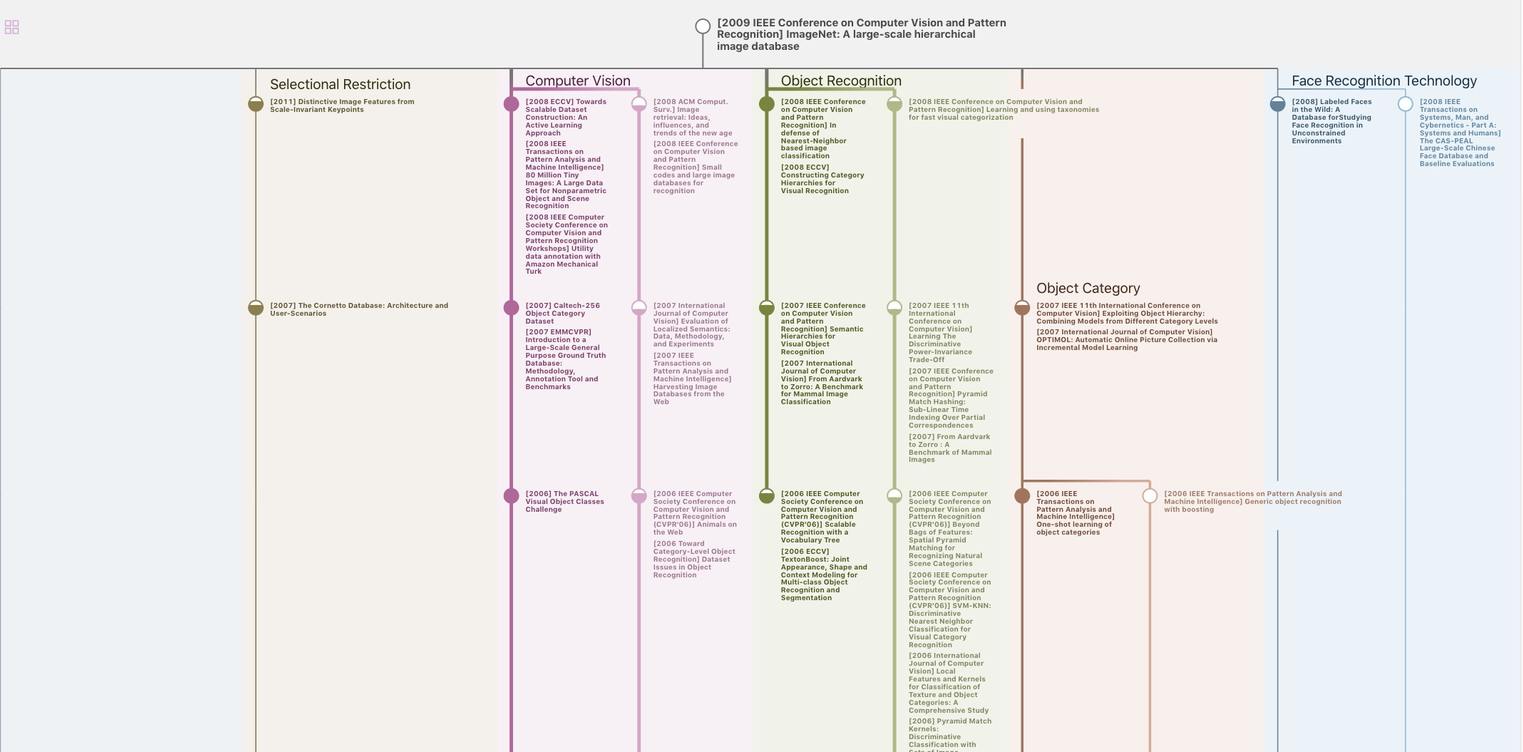
生成溯源树,研究论文发展脉络
Chat Paper
正在生成论文摘要