Ensemble based Dimensionality Reduction for Intrusion Detection using Random Forest in Wireless Networks
2023 5th International Conference on Smart Systems and Inventive Technology (ICSSIT)(2023)
摘要
In the present digital era, the evolution of internet technologies increases rapidly and as a result large number of devices is connected with the public network. Due to this, huge volume of data is been generated and transmitted over the network. Similarly the attacker has formulated large number of ways to access to information on the network. Due to the availability of internet, almost every device are interconnected and are exposed to data breach. Hence Intrusion Detection System (IDS) are mandatory to ensure the information is secure from the attackers. As technology is developing the attackers identifies a new technique to breach the secure data. Machine Learning approach is been employed in different domain and it has given better results in terms of performance and accuracy. In order to design an effective IDS, this study utilizes machine leaning models. This article has proposed an ensemble-based hybrid approach for intrusion detection in networks. In the initial stage, the important features are extracted using Blended Linear Discriminant Analysis (BLDA). Further the dimensionality reduced dataset are used to detect intrusion using Random Forest Classifier. Here, two benchmark datasets namely NSL-KDD and UNSW-NB15 are used to evaluate the potential of the proposed method. To prove the effectiveness of our approach, the proposed scheme is compared with classical LDA, PCA and PLS based feature selection schemes where the presented method provides. The accuracy of the proposed method is 90.12 % and 91.0 % for NSL-KDD and UNSW-NB15 datasets respectively. The results clearly shows that our proposed method provides considerable improvement in performance of IDS.
更多查看译文
关键词
Intrusion detection systenb Dimensionality reduction,Random Forest Classifier,Linear Discriminant Analysis
AI 理解论文
溯源树
样例
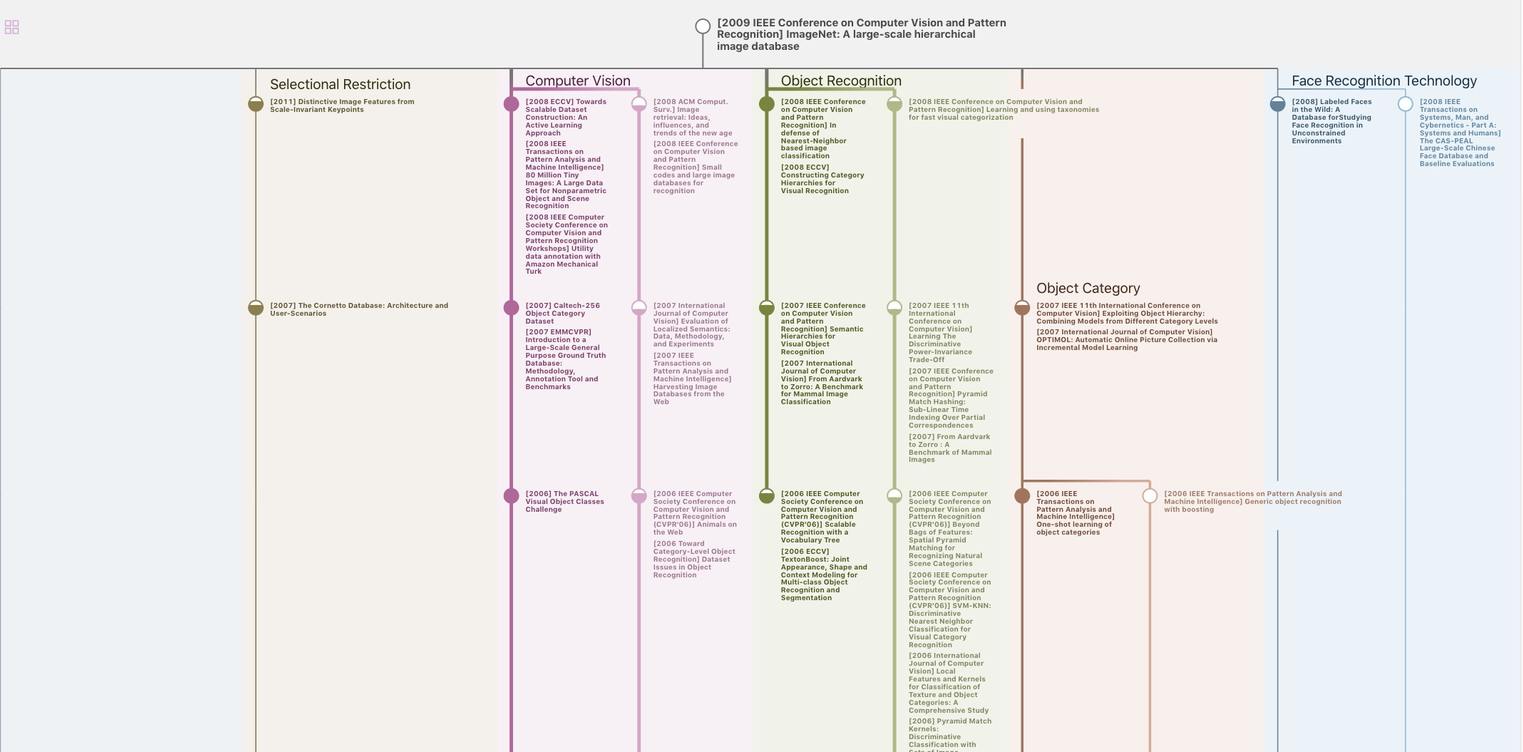
生成溯源树,研究论文发展脉络
Chat Paper
正在生成论文摘要