Online Binaural Speech Separation of Moving Speakers With a Wavesplit Network
arxiv(2023)
摘要
Binaural speech separation in real-world scenarios often involves moving speakers. Most current speech separation methods use utterance-level permutation invariant training (u-PIT) for training. In inference time, however, the order of outputs can be inconsistent over time particularly in long-form speech separation. This situation which is referred to as the speaker swap problem is even more problematic when speakers constantly move in space and therefore poses a challenge for consistent placement of speakers in output channels. Here, we describe a real-time binaural speech separation model based on a Wavesplit network to mitigate the speaker swap problem for moving speaker separation. Our model computes a speaker embedding for each speaker at each time frame from the mixed audio, aggregates embeddings using online clustering, and uses cluster centroids as speaker profiles to track each speaker throughout the long duration. Experimental results on reverberant, long-form moving multitalker speech separation show that the proposed method is less prone to speaker swap and achieves comparable performance with u-PIT based models with ground truth tracking in both separation accuracy and preserving the interaural cues.
更多查看译文
关键词
online binaural speech separation,moving speakers,wavesplit
AI 理解论文
溯源树
样例
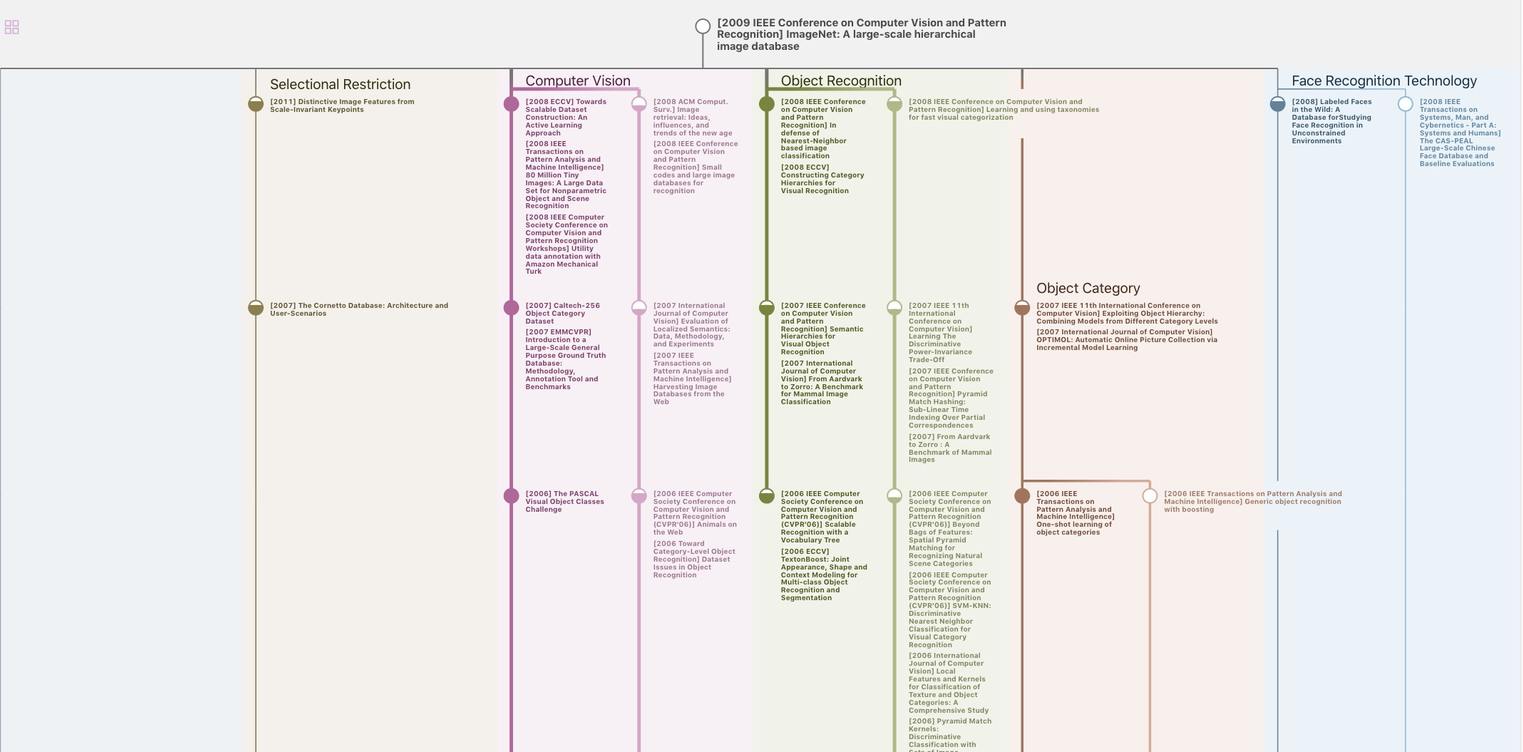
生成溯源树,研究论文发展脉络
Chat Paper
正在生成论文摘要