Predicting muscle invasion in bladder cancer based on MRI: A comparison of radiomics, and single-task and multi-task deep learning
Computer methods and programs in biomedicine(2023)
摘要
Background and objectives: Radiomics and deep learning are two popular technologies used to develop computer-aided detection and diagnosis schemes for analysing medical images. This study aimed to com-pare the effectiveness of radiomics, single-task deep learning (DL) and multi-task DL methods in predict-ing muscle-invasive bladder cancer (MIBC) status based on T2-weighted imaging (T2WI). Methods: A total of 121 tumours (93 for training, from Centre 1; 28 for testing, from Centre 2) were included. MIBC was confirmed with pathological examination. A radiomics model, a single-task model, and a multi-task model based on T2WI were constructed in the training cohort with five-fold cross -validation, and validation was conducted in the external test cohort. Receiver operating characteristic (ROC) curve analysis was performed to evaluate the diagnostic performance of each model. DeLong's test and a permutation test were used to compare the performance of the models. Results: The area under the ROC curve (AUC) values of the radiomics, single-task and multi-task models in the training cohort were: 0.920, 0.933 and 0.932, respectively; and were 0.844, 0.884 and 0.932, re-spectively, in the test cohort. The multi-task model achieved better performance in the test cohort than did the other models. No statistically significant differences in AUC values and Kappa coefficients were observed between pairwise models, in either the training or test cohorts. According to the Grad-CAM fea-ture visualization results, the multi-task model focused more on the diseased tissue area in some samples of the test cohort compared with the single-task model. Conclusions: The T2WI-based radiomics, single-task, and multi-task models all exhibited good diagnostic performance in preoperatively predicting MIBC, in which the multi-task model had the best diagnostic performance. Compared with the radiomics method, our multi-task DL method had the advantage of saving time and effort. Compared with the single-task DL method, our multi-task DL method had the advantage of being more lesion-focused and more reliable for clinical reference. (c) 2023 Published by Elsevier B.V.
更多查看译文
关键词
Bladder cancer,Muscle invasion,Radiomics,Deep learning,Multi-task learning,Magnetic resonance imaging
AI 理解论文
溯源树
样例
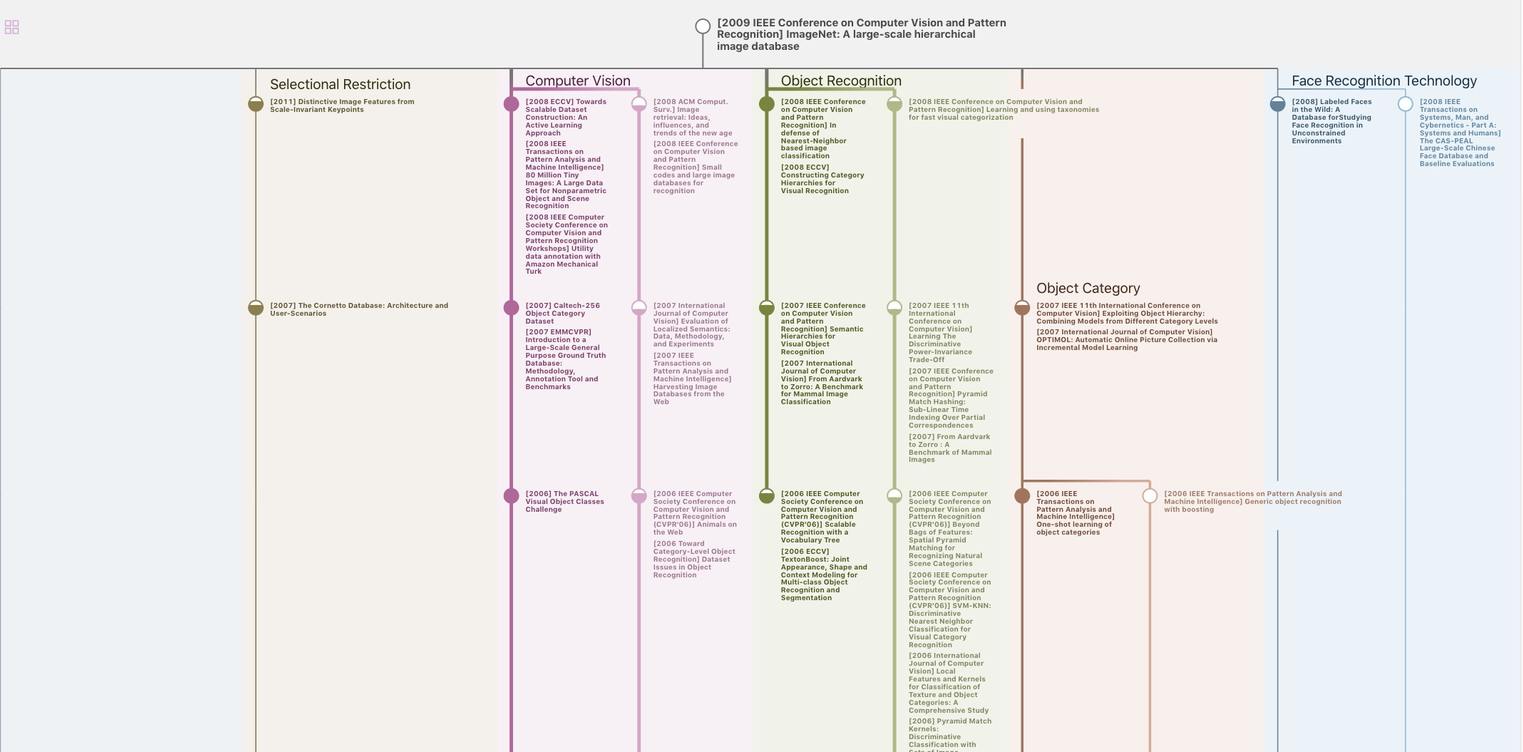
生成溯源树,研究论文发展脉络
Chat Paper
正在生成论文摘要