SGU-Net: Shape-Guided Ultralight Network for Abdominal Image Segmentation
IEEE Journal of Biomedical and Health Informatics(2023)
摘要
Convolutional neural networks (CNNs) have achieved significant success in medical image segmentation. However, they also suffer from the requirement of a large number of parameters, leading to a difficulty of deploying CNNs to low-source hardwares, e.g., embedded systems and mobile devices. Although some compacted or small memory-hungry models have been reported, most of them may cause degradation in segmentation accuracy. To address this issue, we propose a shape-guided ultralight network (SGU-Net) with extremely low computational costs. The proposed SGU-Net includes two main contributions: it first presents an ultralight convolution that is able to implement double separable convolutions simultaneously, i.e., asymmetric convolution and depthwise separable convolution. The proposed ultralight convolution not only effectively reduces the number of parameters but also enhances the robustness of SGU-Net. Secondly, our SGU-Net employs an additional adversarial shape-constraint to let the network learn shape representation of targets, which can significantly improve the segmentation accuracy for abdomen medical images using self-supervision. The SGU-Net is extensively tested on four public benchmark datasets, LiTS, CHAOS, NIH-TCIA and 3Dircbdb. Experimental results show that SGU-Net achieves higher segmentation accuracy using lower memory costs, and outperforms state-of-the-art networks. Moreover, we apply our ultralight convolution into a 3D volume segmentation network, which obtains a comparable performance with fewer parameters and memory usage.
更多查看译文
关键词
Medical image segmentation,deep lear- ning,ultralight convolution,adversarial shape-constraint
AI 理解论文
溯源树
样例
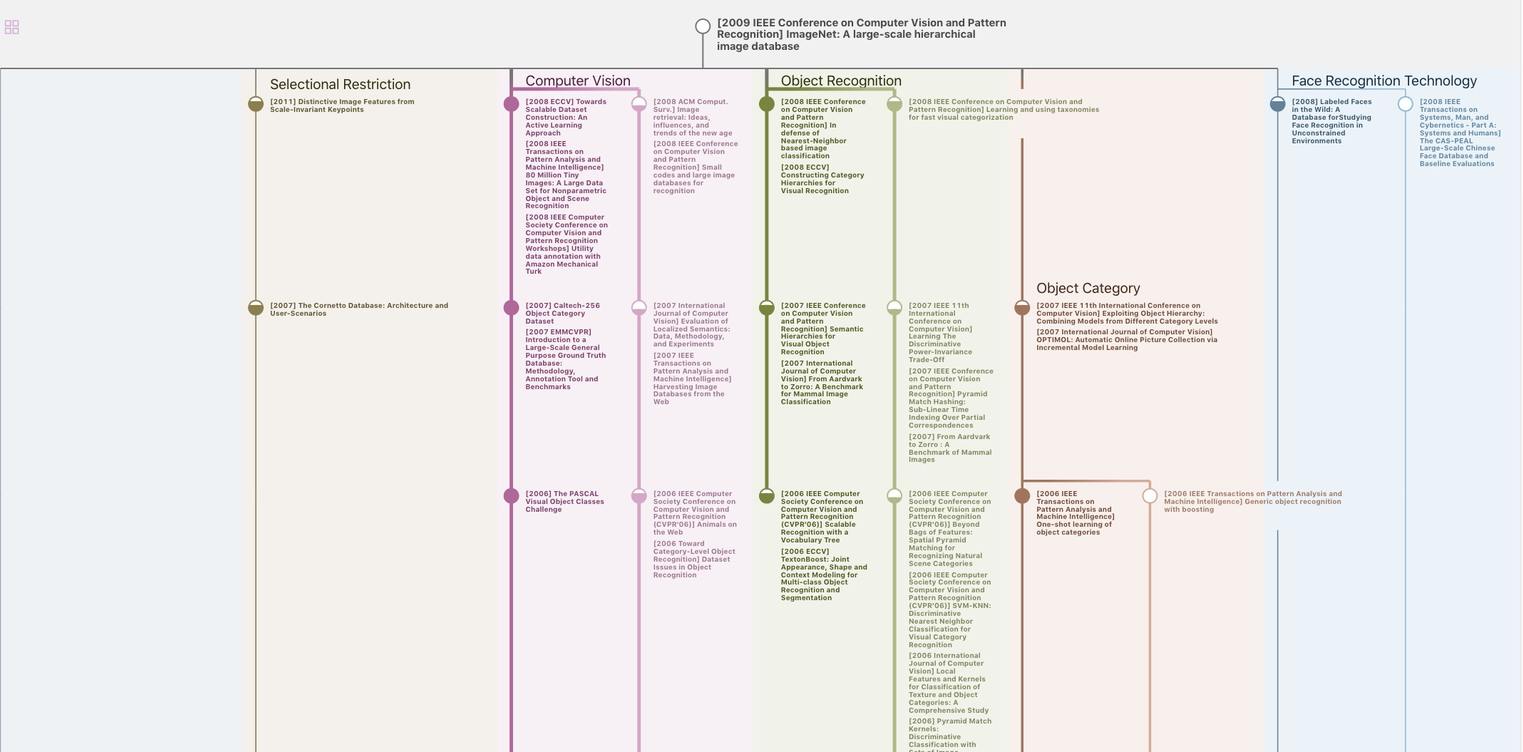
生成溯源树,研究论文发展脉络
Chat Paper
正在生成论文摘要