Deep Learning for Inertial Positioning: A Survey
arXiv (Cornell University)(2023)
摘要
Inertial sensors are widely utilized in smartphones, drones, robots, and IoT devices, playing a crucial role in enabling ubiquitous and reliable localization. Inertial sensor-based positioning is essential in various applications, including personal navigation, location-based security, and human-device interaction. However, low-cost MEMS inertial sensors' measurements are inevitably corrupted by various error sources, leading to unbounded drifts when integrated doubly in traditional inertial navigation algorithms, subjecting inertial positioning to the problem of error drifts. In recent years, with the rapid increase in sensor data and computational power, deep learning techniques have been developed, sparking significant research into addressing the problem of inertial positioning. Relevant literature in this field spans across mobile computing, robotics, and machine learning. In this article, we provide a comprehensive review of deep learning-based inertial positioning and its applications in tracking pedestrians, drones, vehicles, and robots. We connect efforts from different fields and discuss how deep learning can be applied to address issues such as sensor calibration, positioning error drift reduction, and multi-sensor fusion. This article aims to attract readers from various backgrounds, including researchers and practitioners interested in the potential of deep learning-based techniques to solve inertial positioning problems. Our review demonstrates the exciting possibilities that deep learning brings to the table and provides a roadmap for future research in this field.
更多查看译文
关键词
inertial positioning,deep learning
AI 理解论文
溯源树
样例
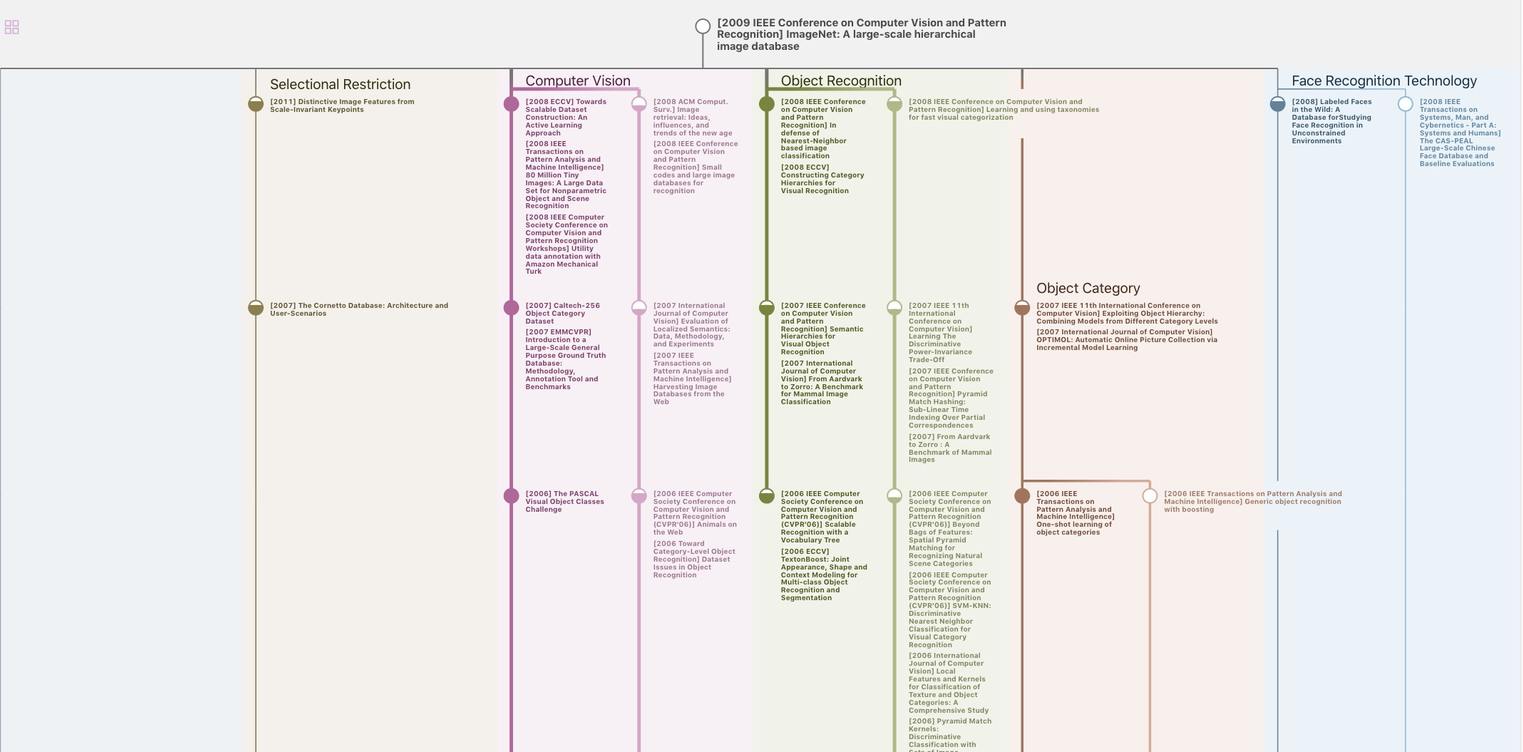
生成溯源树,研究论文发展脉络
Chat Paper
正在生成论文摘要