Federated Virtual Learning on Heterogeneous Data with Local-global Distillation
arxiv(2023)
摘要
Despite Federated Learning (FL)'s trend for learning machine learning models in a distributed manner, it is susceptible to performance drops when training on heterogeneous data. Recently, dataset distillation has been explored in order to improve the efficiency and scalability of FL by creating a smaller, synthetic dataset that retains the performance of a model trained on the local private datasets. We discover that using distilled local datasets can amplify the heterogeneity issue in FL. To address this, we propose a new method, called Federated Virtual Learning on Heterogeneous Data with Local-Global Distillation (FEDLGD), which trains FL using a smaller synthetic dataset (referred as virtual data) created through a combination of local and global distillation. Specifically, to handle synchronization and class imbalance, we propose iterative distribution matching to allow clients to have the same amount of balanced local virtual data; to harmonize the domain shifts, we use federated gradient matching to distill global virtual data that are shared with clients without hindering data privacy to rectify heterogeneous local training via enforcing local-global feature similarity. We experiment on both benchmark and real-world datasets that contain heterogeneous data from different sources. Our method outperforms state-of-the-art heterogeneous FL algorithms under the setting with a very limited amount of distilled virtual data.
更多查看译文
关键词
heterogeneous data,learning,local-global
AI 理解论文
溯源树
样例
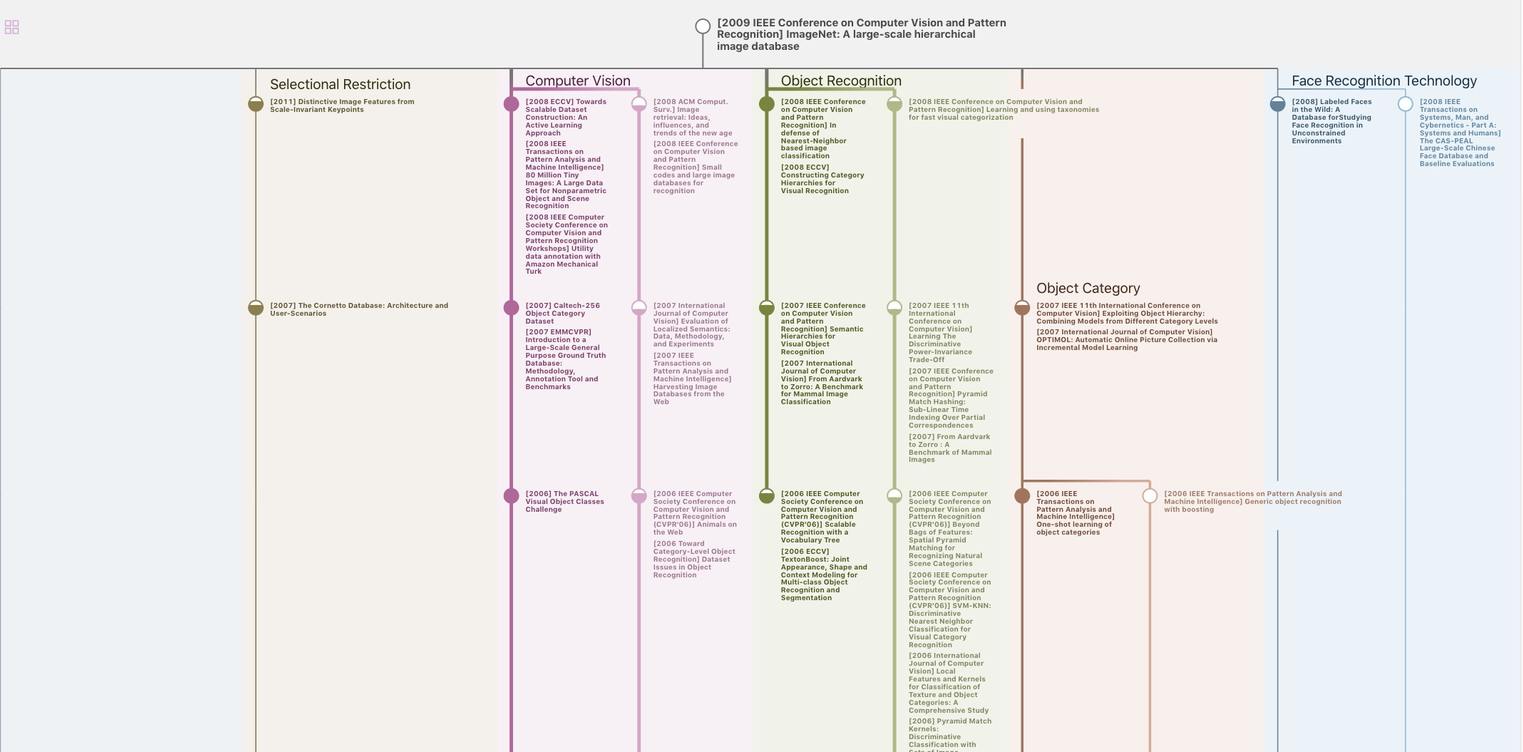
生成溯源树,研究论文发展脉络
Chat Paper
正在生成论文摘要