Study of Distractors in Neural Models of Code
CoRR(2023)
Abstract
Finding important features that contribute to the prediction of neural models is an active area of research in explainable AI. Neural models are opaque and finding such features sheds light on a better understanding of their predictions. In contrast, in this work, we present an inverse perspective of distractor features: features that cast doubt about the prediction by affecting the model's confidence in its prediction. Understanding distractors provide a complementary view of the features' relevance in the predictions of neural models. In this paper, we apply a reduction-based technique to find distractors and provide our preliminary results of their impacts and types. Our experiments across various tasks, models, and datasets of code reveal that the removal of tokens can have a significant impact on the confidence of models in their predictions and the categories of tokens can also play a vital role in the model's confidence. Our study aims to enhance the transparency of models by emphasizing those tokens that significantly influence the confidence of the models.
MoreTranslated text
Key words
Explainable AI,Distractors,Models of Code
AI Read Science
Must-Reading Tree
Example
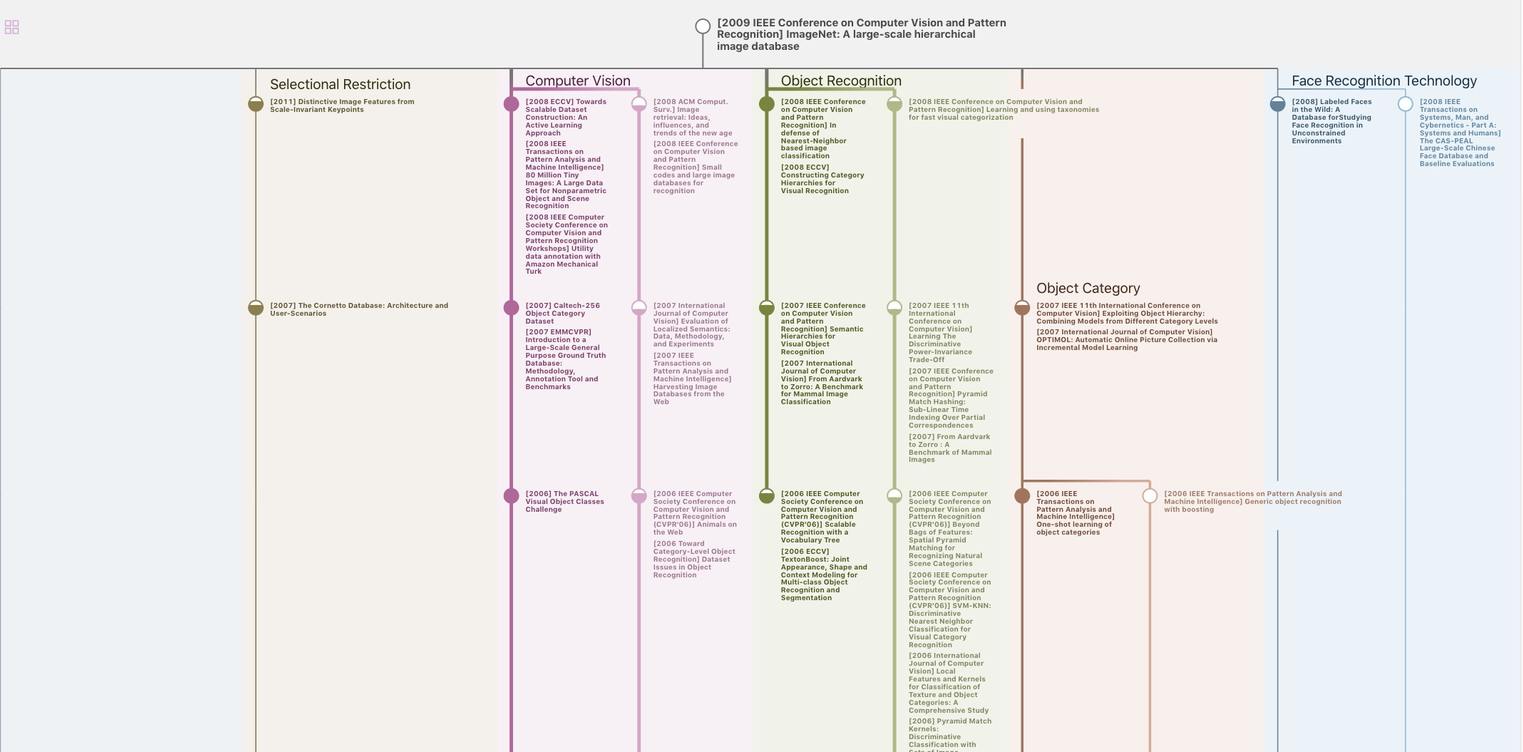
Generate MRT to find the research sequence of this paper
Chat Paper
Summary is being generated by the instructions you defined