Incremental improvements in tractometry-based brain-age modeling with deep learning
biorxiv(2023)
摘要
Multivariate measurements of human brain white matter (WM) with diffusion MRI (dMRI) provide information about the role of WM in a variety of cognitive functions and in brain health. Statistical models take advantage of the regularities in these data to make inferences about individual differences. For example, dMRI data provide the basis for accurate brain-age models: models that predict the chronological age of participants from WM tissue properties. Deep learning (DL) models are powerful machine learning models, which have been shown to provide benefits in many multivariate analysis settings. We investigated whether DL would provide substantial improvements for brain-age models based on dMRI measurements of WM in a large sample of children and adolescents. We found that some DL models fit the data better than a linear baseline, but the differences are small. In particular, recurrent neural network architectures provide up to ~6% improvement in accuracy. This suggests that information about WM development is mostly accessible with linear models, and does not require the additional invariance and non-linearity offered by DL models. However, in some applications this incremental improvement may prove critical. We provide open-source software that fits DL models to dMRI data (https://yeatmanlab.github.io/AFQ-Insight).
### Competing Interest Statement
The authors have declared no competing interest.
更多查看译文
关键词
deep learning,incremental improvements,tractometry-based,brain-age
AI 理解论文
溯源树
样例
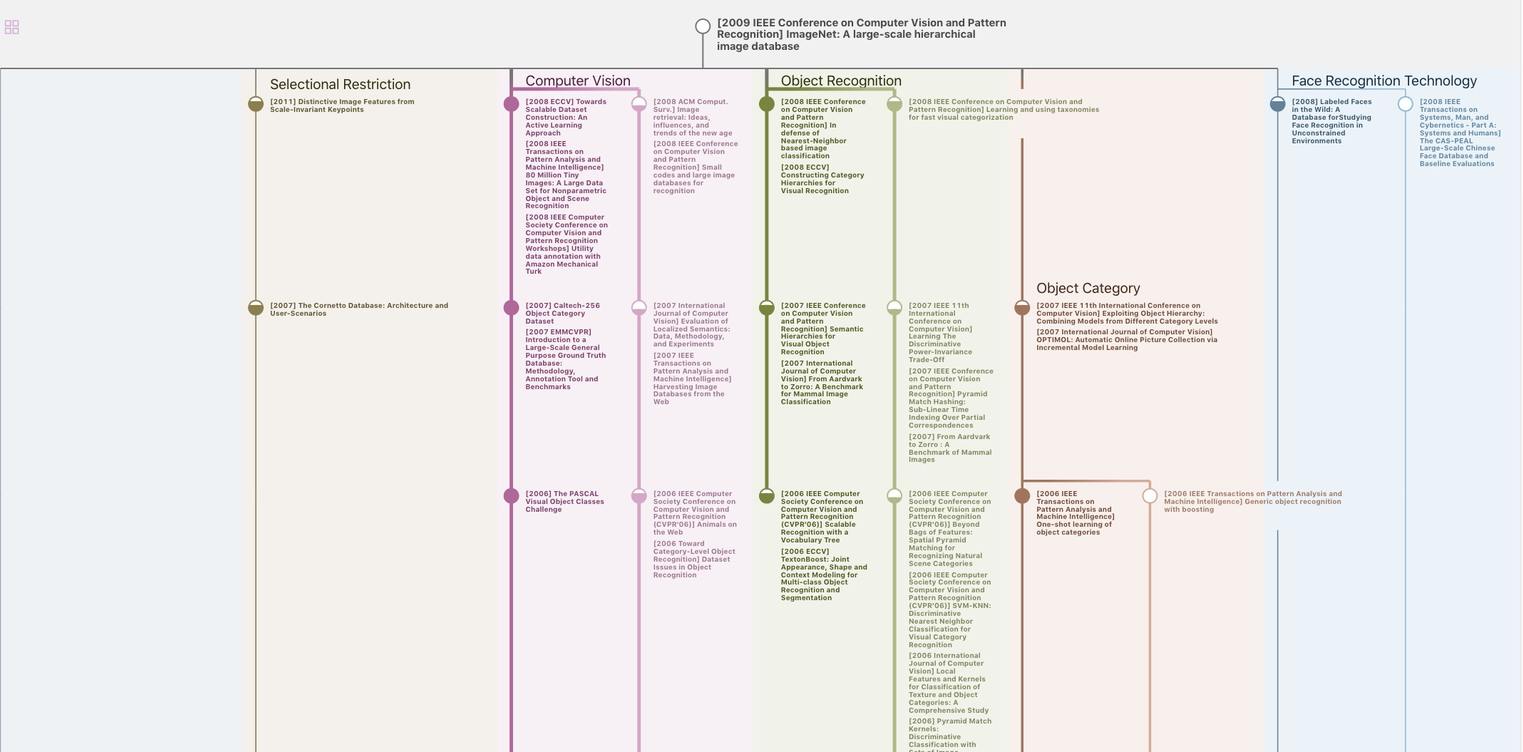
生成溯源树,研究论文发展脉络
Chat Paper
正在生成论文摘要