Gait classification for early detection and severity rating of Parkinson's disease based on hybrid signal processing and machine learning methods
COGNITIVE NEURODYNAMICS(2024)
摘要
Parkinson's disease (PD) is one of the cognitive degenerative disorders of the central nervous system that affects the motor system. Gait dysfunction represents the pathology of motor symptom while gait analysis provides clinicians with sub clinical information reflecting subtle differences between PD patients and healthy controls (HCs). Currently neurologists usually assess several clinical manifestations of the PD patients and rate the severity level according to some established criteria. This is highly dependent on clinician's expertise which is subjective and ineffective. In the present study we address these issues by proposing a hybrid signal processing and machine learning based gait classification system for gait anomaly detection and severity rating of PD patients. Time series of vertical ground reaction force (VGRF) data are utilized to represent discriminant gait information. First, phase space of the VGRF is reconstructed, in which the properties associated with the nonlinear gait system dynamics are preserved. Then Shannon energy is used to extract the characteristic envelope of the phase space signal. Third, Shannon energy envelope is decomposed into high and low resonance components using dual Q-factor signal decomposition derived from tunable Q-factor wavelet transform. Note that the high Q factor component consists largely of sustained oscillatory behavior, while the low Q-factor component consists largely of transients and oscillations that are not sustained. Fourth, variational mode decomposition is employed to decompose high and low resonance components into different intrinsic modes and provide representative features. Finally features are fed to five different types of machine learning based classifiers for the anomaly detection and severity rating of PD patients based on Hohen and Yahr (HY) scale. The effectiveness of this strategy is verified using a Physionet gait database consisting of 93 idiopathic PD patients and 73 age-matched asymptomatic HCs. When evaluated with 10-fold cross-validation method for early PD detection and severity rating, the highest classification accuracy is reported to be 98.20% and 96.69%, respectively, by using the support vector machine classifier. Compared with other state-of-the-art methods, the results demonstrate superior performance and support the validity of the proposed method.
更多查看译文
关键词
Parkinson's disease,Machine learning,Signal processing,Gait classification,Vertical ground reaction force (VGRF),Hohen and Yahr (HY) scale
AI 理解论文
溯源树
样例
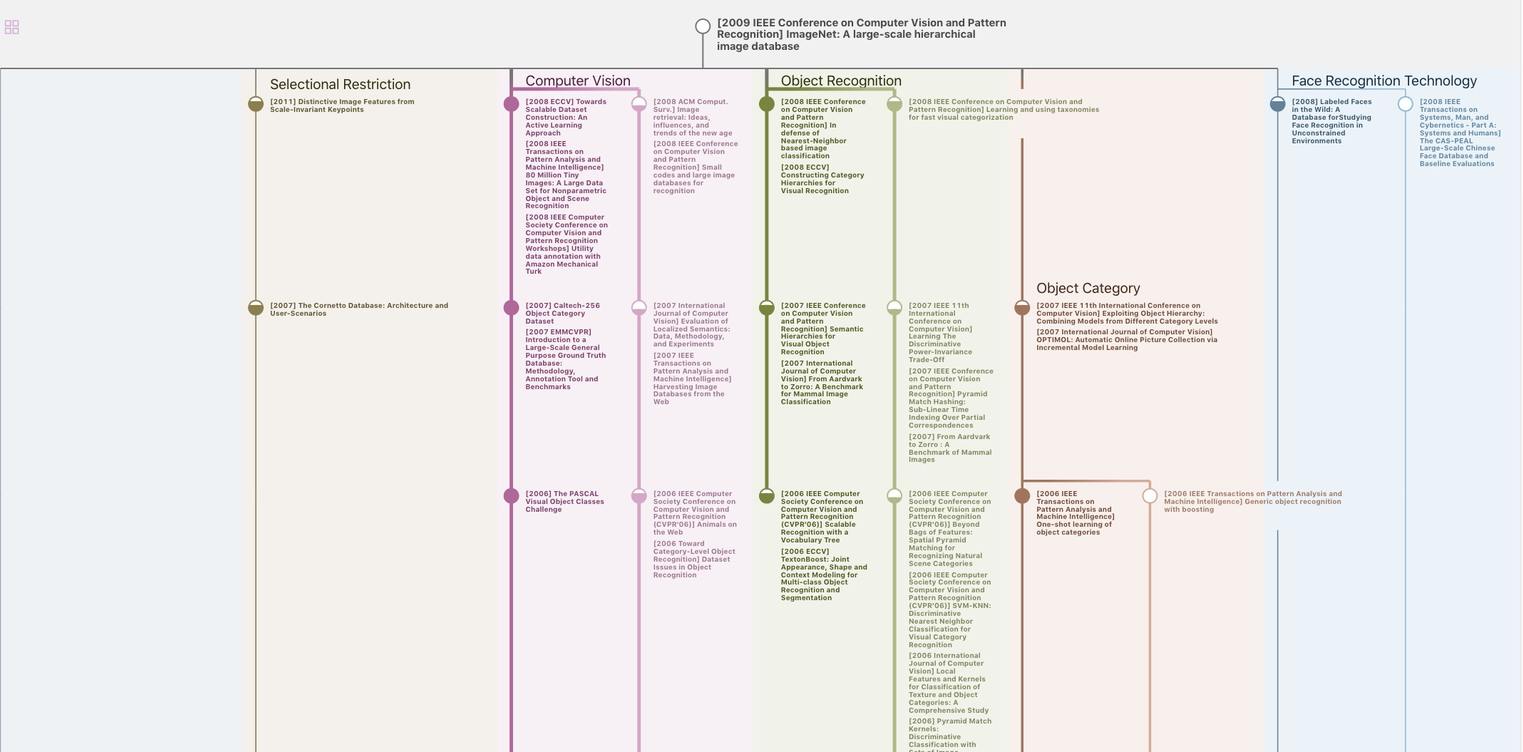
生成溯源树,研究论文发展脉络
Chat Paper
正在生成论文摘要