Prediction-accuracy improvement of neural network to ferromagnetic multilayers by Gaussian data augmentation and ensemble learning
COMPUTATIONAL MATERIALS SCIENCE(2023)
摘要
In materials informatics using machine learning and density functional theory (DFT) calculations, it is often hard to obtain enough database due to extremely large costs of DFT. Therefore, it is required a machine learning technique that learns a complex target relationship from a limited dataset. In the present work, to overcome this issue, we built a neural network by implementing two techniques: Gaussian data augmentation (GDA) method, which injects Gaussian noises into the training dataset, and ensemble learning, which employs multiple models to train and make prediction by averaging their outputs. With typical examples of magnetic moment and formation energy as a function of atomic-layer configuration in CoFe multilayers, the prediction accuracy can be greatly improved, e.g., by using a training dataset consisting of 10 similar to 30% of all data. We found that the use of GDA substantially increases the prediction accuracy for unknown test dataset where the improvement is attributed to a smoothing effect of a fitting curve of NN, and a combination with the ensemble learning brings further improvement with reducing the variance error originating from the selection of training sampling dataset in addition to a similar smoothing effect. The present approach, thus, can be generalized widely to materials informatics for which database is limited.
更多查看译文
关键词
Neural network,Gaussian data augmentation method,Ensemble learning,First-principles calculations,Magnetic multilayers
AI 理解论文
溯源树
样例
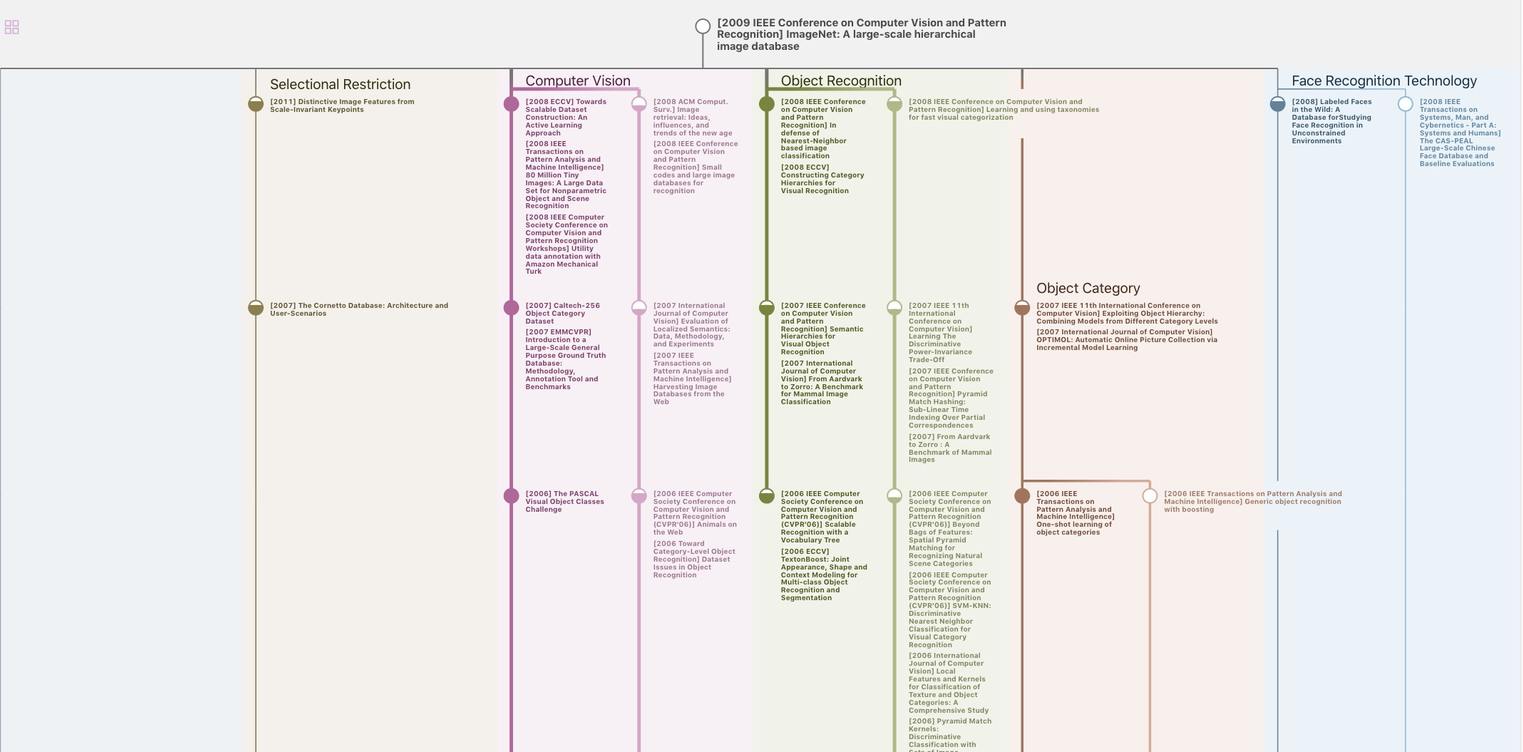
生成溯源树,研究论文发展脉络
Chat Paper
正在生成论文摘要