Gradient-Based Local Causal Structure Learning
IEEE TRANSACTIONS ON CYBERNETICS(2024)
摘要
Finding the causal structure from a set of variables given observational data is a crucial task in many scientific areas. Most algorithms focus on discovering the global causal graph but few efforts have been made toward the local causal structure (LCS), which is of wide practical significance and easier to obtain. LCS learning faces the challenges of neighborhood determination and edge orientation. Available LCS algorithms build on conditional independence (CI) tests, they suffer the poor accuracy due to noises, various data generation mechanisms, and small-size samples of real-world applications, where CI tests do not work. In addition, they can only find the Markov equivalence class, leaving some edges undirected. In this article, we propose a GradieNt-based LCS learning approach (GraN-LCS) to determine neighbors and orient edges simultaneously in a gradient-descent way, and, thus, to explore LCS more accurately. GraN-LCS formulates the causal graph search as minimizing an acyclicity regularized score function, which can be optimized by efficient gradient-based solvers. GraN-LCS constructs a multilayer perceptron (MLP) to simultaneously fit all other variables with respect to a target variable and defines an acyclicity-constrained local recovery loss to promote the exploration of local graphs and to find out direct causes and effects of the target variable. To improve the efficacy, it applies preliminary neighborhood selection (PNS) to sketch the raw causal structure and further incorporates an l(1) -norm-based feature selection on the first layer of MLP to reduce the scale of candidate variables and to pursue sparse weight matrix. GraN-LCS finally outputs LCS based on the sparse weighted adjacency matrix learned from MLPs. We conduct experiments on both synthetic and real-world datasets and verify its efficacy by comparing against state-of-the-art baselines. A detailed ablation study investigates the impact of key components of GraN-LCS and the results prove their contribution.
更多查看译文
关键词
Acyclicity,causal structural learning,direct causes and effects,feature selection,gradient-based search
AI 理解论文
溯源树
样例
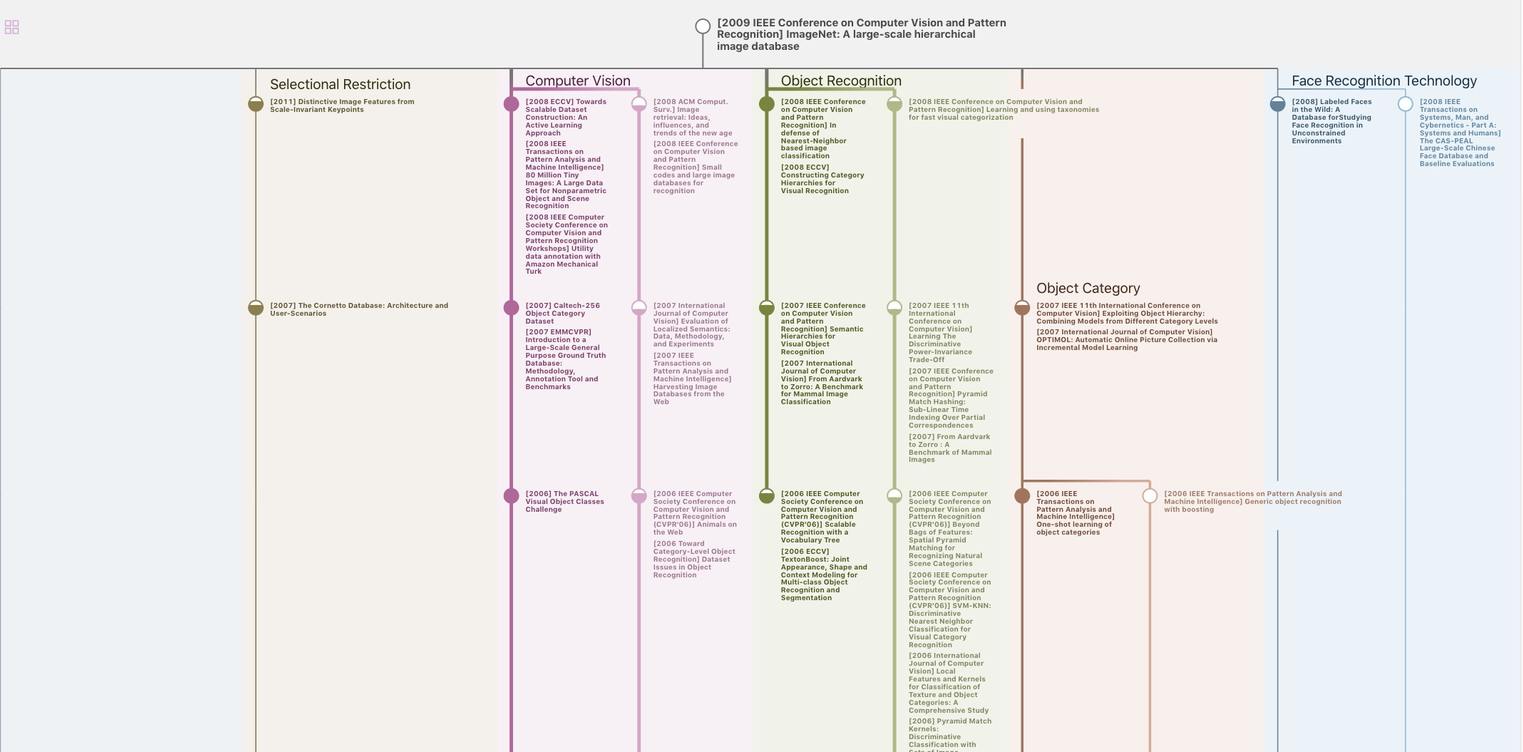
生成溯源树,研究论文发展脉络
Chat Paper
正在生成论文摘要