VARAHA: A Fast Non-Markovian sampler for estimating Gravitational-Wave posteriors
arxiv(2023)
摘要
This article introduces VARAHA, an open-source, fast, non-Markovian sampler for estimating gravitational-wave posteriors. VARAHA differs from existing Nested sampling algorithms by gradually discarding regions of low likelihood, rather than gradually sampling regions of high likelihood. This alternative mindset enables VARAHA to freely draw samples from anywhere within the high-likelihood region of the parameter space, allowing for analyses to complete in significantly fewer cycles. This means that VARAHA can significantly reduce both the wall and CPU time of all analyses. VARAHA offers many benefits, particularly for gravitational-wave astronomy where Bayesian inference can take many days, if not weeks, to complete. For instance, VARAHA can be used to estimate accurate sky locations, astrophysical probabilities and source classifications within minutes, which is particularly useful for multi-messenger follow-up of binary neutron star observations; VARAHA localises GW170817 $\sim 30$ times faster than LALInference. Although only aligned-spin, dominant multipole waveform models can be used for gravitational-wave analyses, it is trivial to extend this algorithm to include additional physics without hindering performance. We envision VARAHA being used for gravitational-wave studies, particularly estimating parameters using expensive waveform models, analysing subthreshold gravitational-wave candidates, generating simulated data for population studies, and rapid posterior estimation for binary neutron star mergers.
更多查看译文
关键词
posteriors,non-markovian,gravitational-wave
AI 理解论文
溯源树
样例
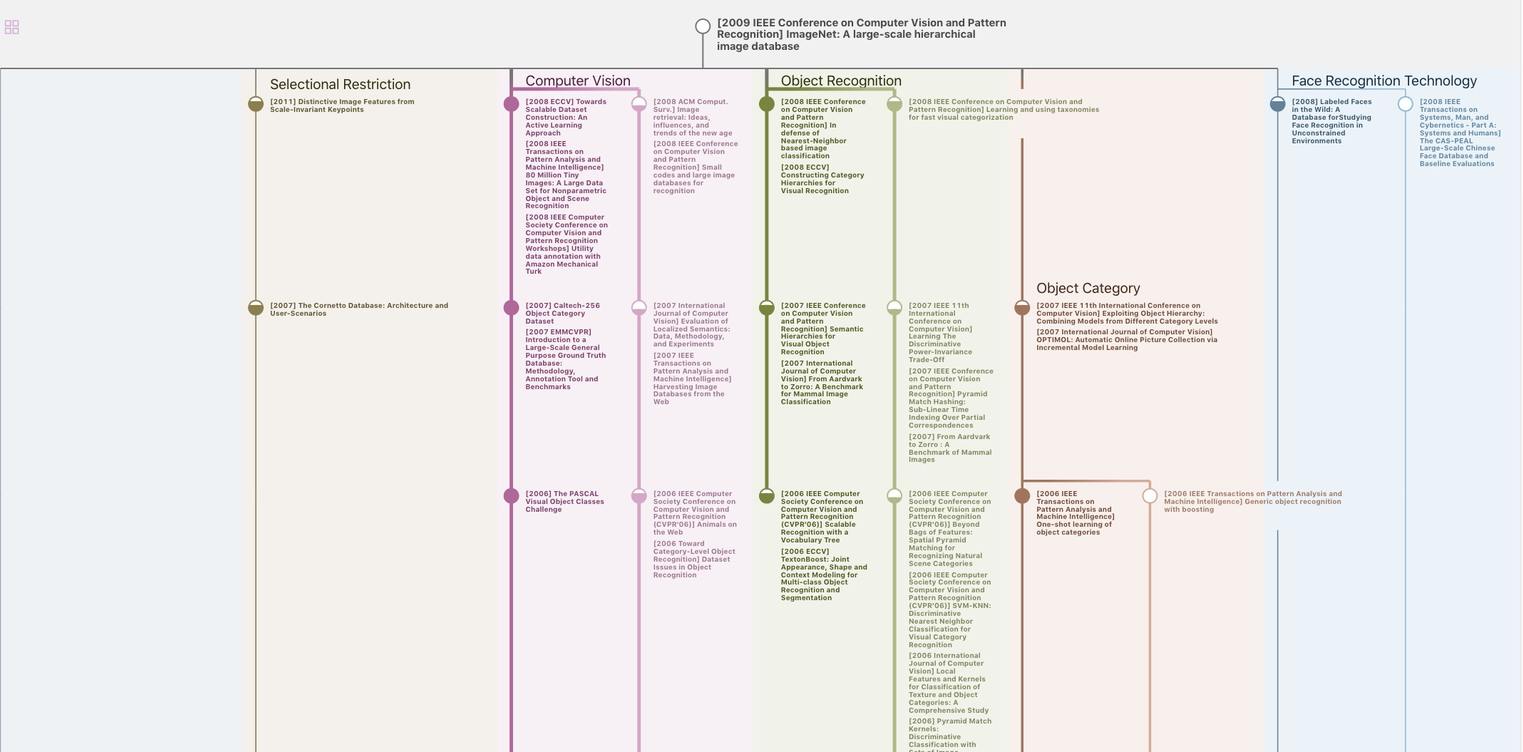
生成溯源树,研究论文发展脉络
Chat Paper
正在生成论文摘要