Domain-aware triplet loss in domain generalization
Computer Vision and Image Understanding(2024)
摘要
Despite the considerable advances in deep learning for object recognition, there are still several factors that hinder the performance of deep learning models. One of these factors is domain shift, which occurs due to variations in the distribution of the testing and training data. This paper addresses the issue of compact feature clustering in domain generalization, with the aim of optimizing the embedding space from multi-domain data. Specifically, we propose a domain-aware triplet loss for domain generalization, which not only facilitates clustering of similar semantic features but also disperses features that arise from the domain. Unlike previous methods that focus on aligning distributions, our algorithm disperses domain information in the embedding space. Our approach is based on the assumption that embedding features can be clustered based on domain information, which is supported mathematically and empirically in this paper.Furthermore, in our investigation of feature clustering in domain generalization, we observe that the factors that influence the convergence of metric learning loss in domain generalization are more significant than the pre-defined domains. To address this issue, we utilize two methods to normalize the embedding space and reduce the internal covariate shift of the embedding features. Our ablation study illustrates the effectiveness of our algorithm. Additionally, our experiments on benchmark datasets, including PACS, VLCS, and Office-Home, demonstrate that our method outperforms related approaches that focus on domain discrepancy. Notably, our results on RegnetY-16GF are substantially better than state-of-the-art methods on the benchmark datasets. Our code is available at https://github.com/workerbcd/DCT.
更多查看译文
关键词
Domain generalization,Contrastive learning,Domain dispersion
AI 理解论文
溯源树
样例
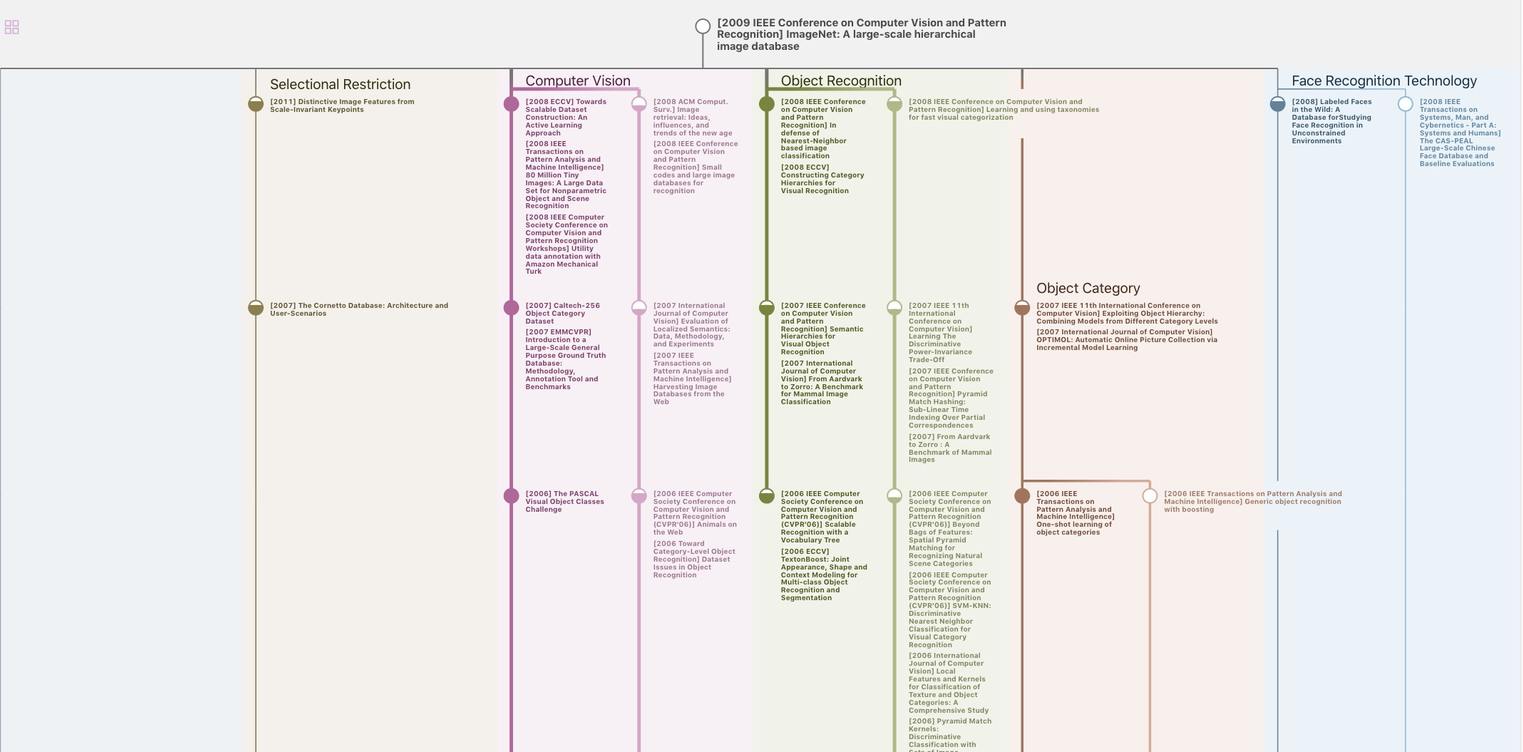
生成溯源树,研究论文发展脉络
Chat Paper
正在生成论文摘要